(166n) Machine-Learning Driven Potential Energy Surface for Nanoparticles Alloy System
AIChE Annual Meeting
2020
2020 Virtual AIChE Annual Meeting
Nanoscale Science and Engineering Forum
Poster Session: Nanoscale Science and Engineering
Thursday, November 19, 2020 - 8:00am to 9:00am
In developing machine-learning potential energy surface for nanoparticles, it is difficult to map the training data of the structure. Conventional input symmetry functions of atomic distance and angle is restricted in bulk system. However, the structures of nanoparticles are dynamic which makes the machine-learned potential predict the energies and forces. Therefore, it is of importance to account structures that are off from the crystalline. Here, we obtain the structures from the real TEM images, understand the effect of input structures for the machine-learning potentials and extend the methodology to alloy system to characterize the stability of the nanoparticles. This will provide the efficient way to investigate the nanoparticle growth mechanism.
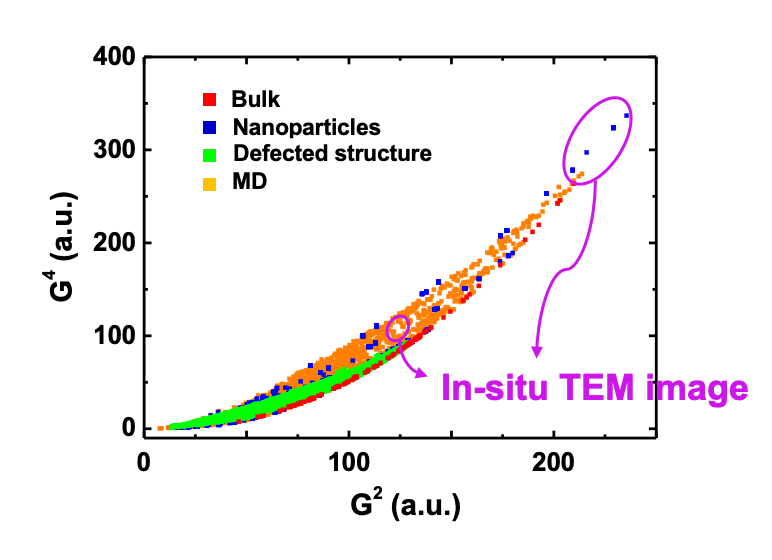
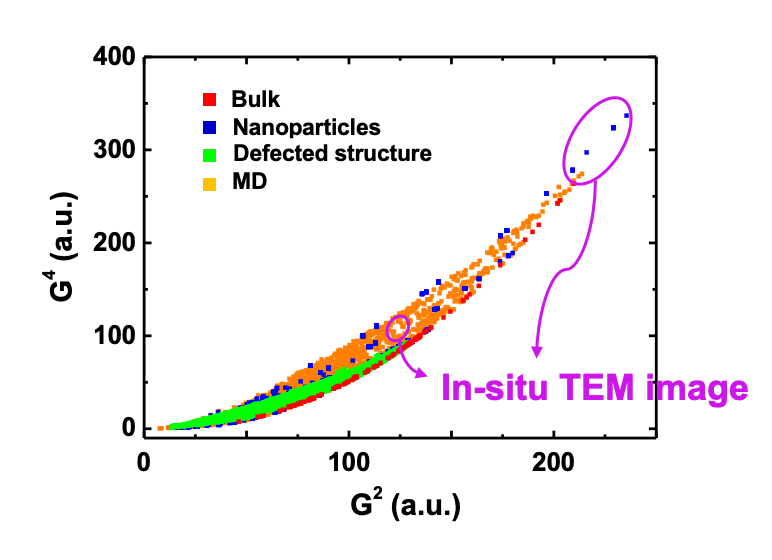