(169o) Multimodal Language and Graph Learning of Adsorption Energy Prediction
AIChE Annual Meeting
2024
2024 AIChE Annual Meeting
Computational Molecular Science and Engineering Forum
Poster Session: Computational Molecular Science and Engineering Forum
Monday, October 28, 2024 - 3:30pm to 5:00pm
In machine learning models designed for catalyst screening, adsorption energy is often the primary modeling target because it acts as a key descriptor of reactivity. This energy is determined by identifying the minimum energy among various adsorption configurations on the catalytic surface. This process necessitates the models to differentiate subtle differences in energy levels and structures across the adsorption configurations. Graph Neural Networks (GNNs) have emerged as the favored method for modeling atomic systems. However, the graph representation requires exact atomic spatial coordinates and often lacks human interpretability. Conversely, recent advances in language models have extended their utility in predicting catalytic properties, presenting an alternative to complex graph representations. These models are proficient in processing human-readable text data, facilitating the seamless integration of observable features. However, their energy prediction accuracy, with a mean absolute error (MAE) of 0.71 eV, is insufficient for discerning the subtle differences in energy that could vary from 0.1 to 0.3 eV between adsorption configurations. This study aims to address this limitation in accuracy by introducing a self-supervised multimodal learning strategy, termed graph-assisted pretraining. This technique employs a contrastive loss between embeddings from graph and text encoders, thereby enabling the knowledge transfer from graph to text embeddings by aligning them across the same systems. This method has notably enhanced the MAE to 0.35 eV, achieving accuracy on par with DimeNet++ while utilizing merely 0.4% of the training data. Moreover, the Transformer encoder of the language model enables an examination of feature focus through its attention scores. This indicates that our multimodal training approach effectively redirects the modelâs focus toward relevant adsorption configurations and adsorbate-related features. This study lays the groundwork for merging different modality representations in adsorbate-catalyst system studies.
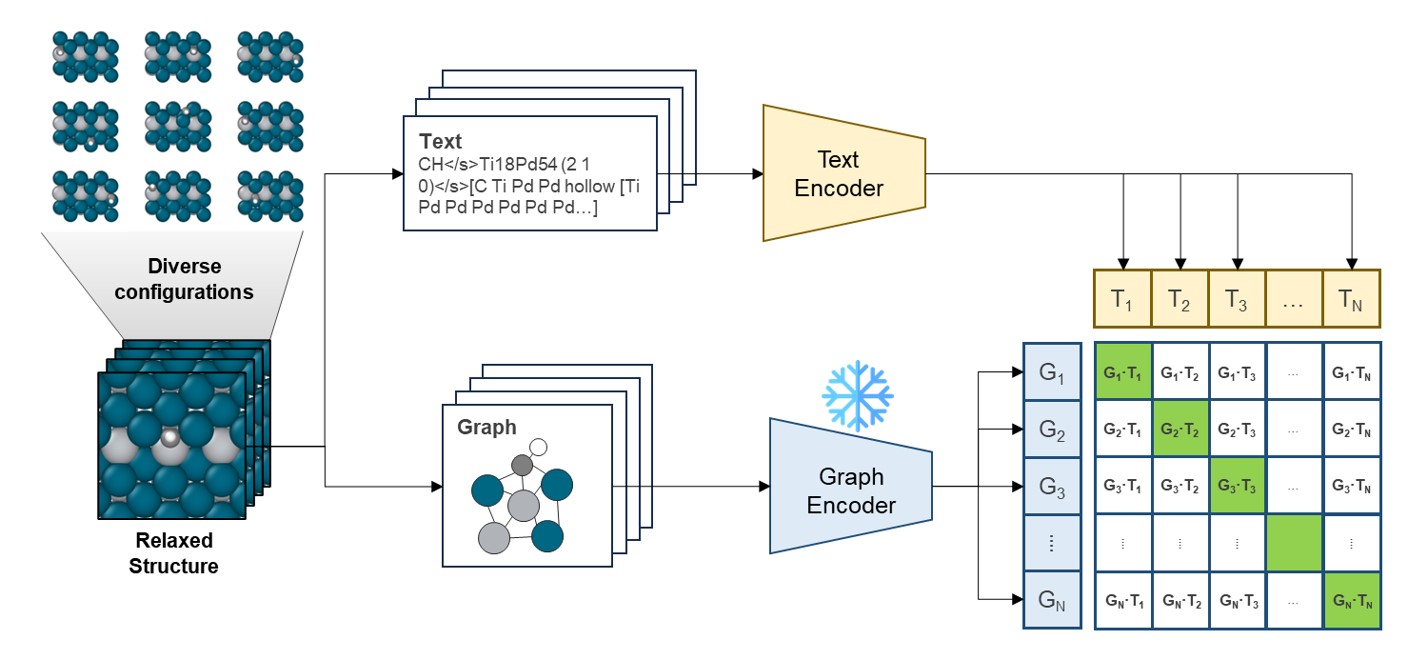
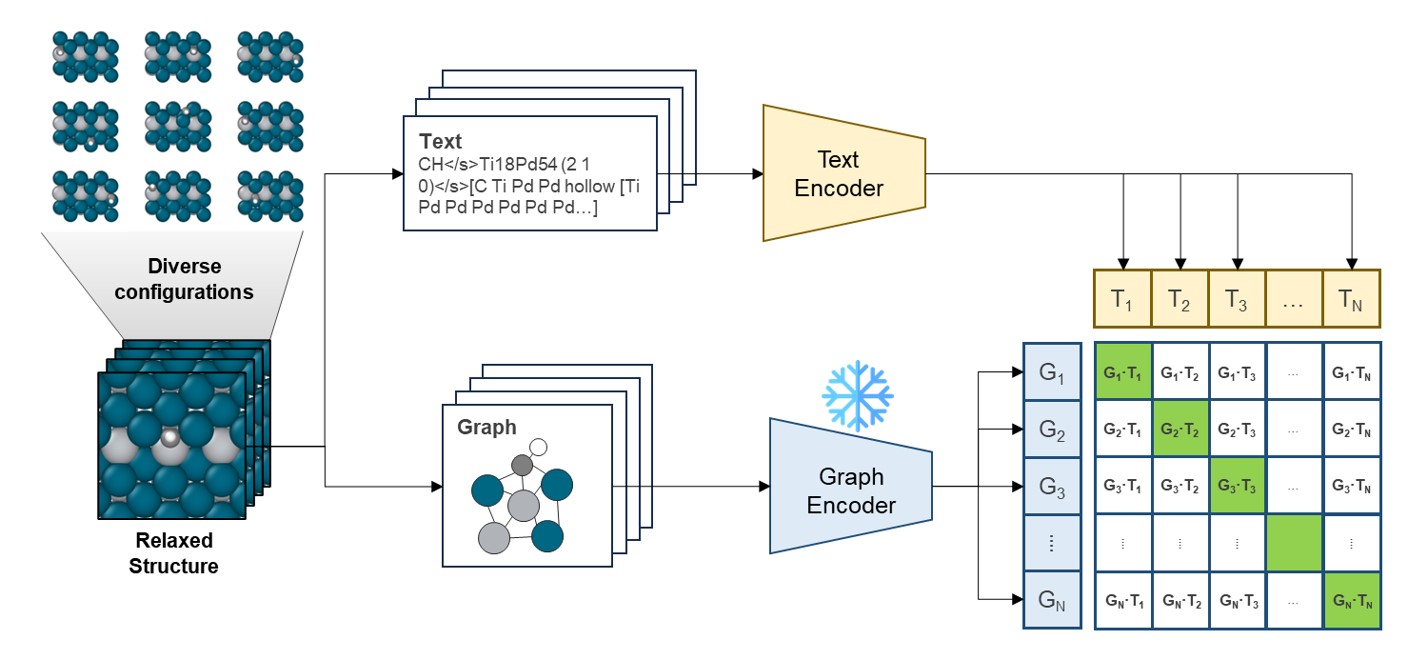