(420a) Predicting Phase-Specific Polymerization Thermodynamics for Circular Polymer Design Using Machine Learning
AIChE Annual Meeting
2023
2023 AIChE Annual Meeting
Computational Molecular Science and Engineering Forum
Automated Molecular and Materials Discovery: Integrating Machine Learning, Simulation, and Experiment II
Tuesday, November 7, 2023 - 3:30pm to 3:45pm
The swift rise in plastics production and usage, alongside inadequate recycling rates, has created a demand for sustainable materials that are easy to recycle. Intrinsically circular polymers (ICPs) are a promising class of materials that can be recycled at mild conditions through chemical conversion to their pure monomer form, making them an attractive alternative to conventional plastics. Designing ICPs computationally requires precise knowledge of the polymerizing systemâs thermodynamics, which are difficult to predict using molecular modeling methods such as density functional theory (DFT). This complexity arises from the interplay between monomer structure, polymer chain length and phase, with the entropic contributions particularly challenging to capture accurately. To rein in the high-dimensional nature of this problem, we propose using machine learning (ML) methods to predict thermodynamics of polymerization using features that describe system-wide interactions while staying within the constraints of the design space, focusing initially on the enthalpy of polymerization. This approach uses an experimental data set collected from literature and insights gained from simulation to select a relevant feature set. The models are benchmarked using a variety of ML workflows. The overall workflow for this approach is outlined in Figure 1. Our model can predict the enthalpy of polymerization for a given monomer structure and phase/solvent combination. By combining this machine learning approach with ML-informed chemical network expansion methods, we aim to design novel ICPs with desirable thermodynamic properties for experimental synthesis, with the goal of contributing to plastic waste reduction.
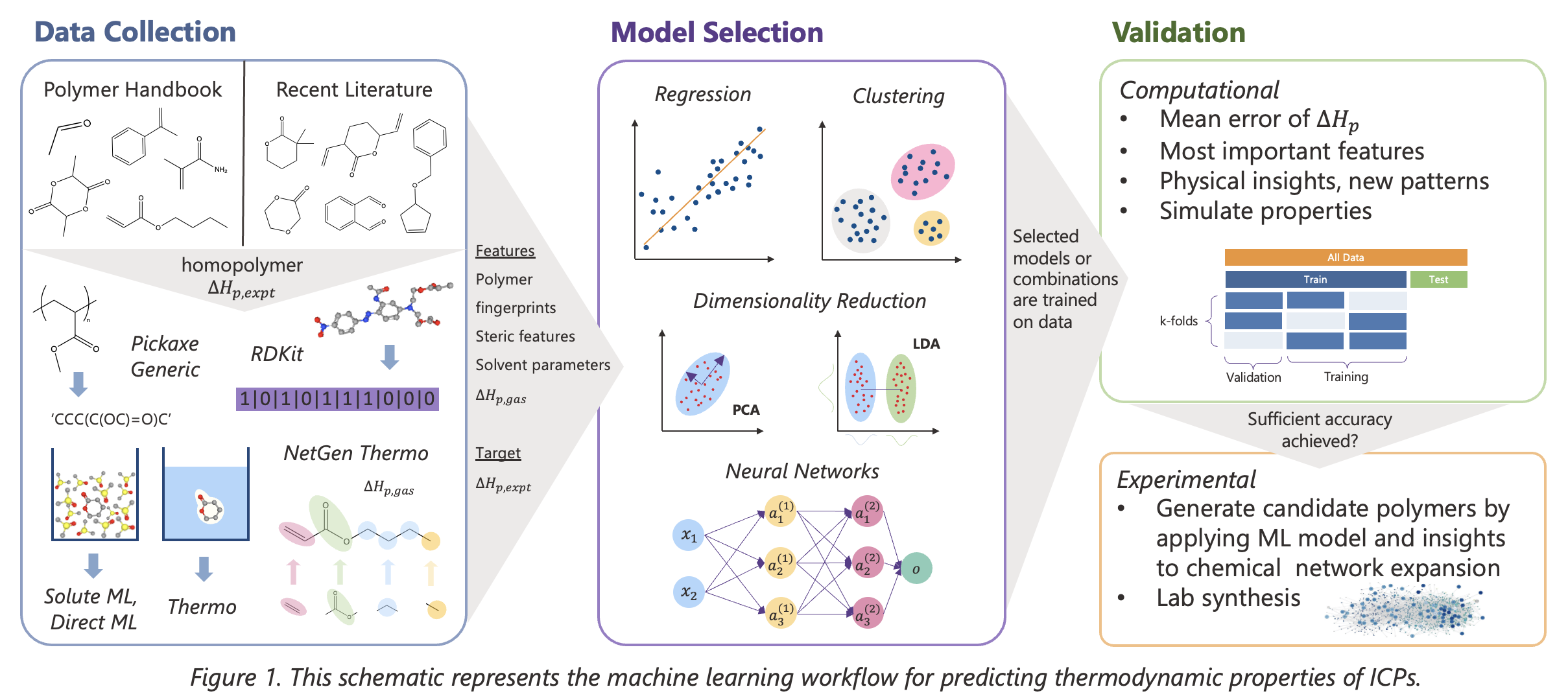
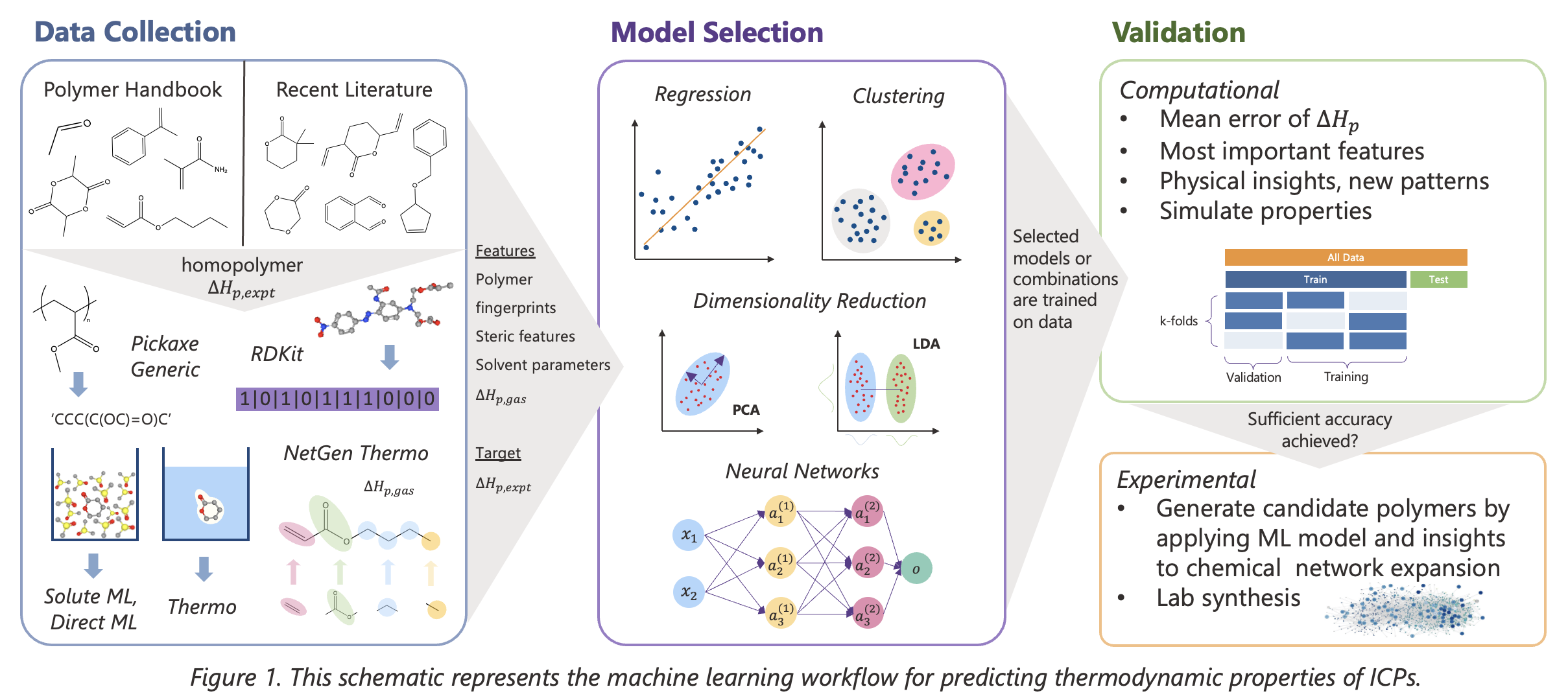