(420i) Learning Material Stability Scores from Computationally Generated Kinetic Data
AIChE Annual Meeting
2023
2023 AIChE Annual Meeting
Computational Molecular Science and Engineering Forum
Automated Molecular and Materials Discovery: Integrating Machine Learning, Simulation, and Experiment II
Tuesday, November 7, 2023 - 5:30pm to 5:45pm
Despite the rapid progress in computational property prediction of novel materials, the absence of computational methods to predict kinetic degradation characteristics represents a costly bottleneck to inserting new materials into applications. While there are experimental stress testing methods to characterize material stability, such methods sacrifice the time and cost benefits of computational design and only supply information retrospectively rather than during the materials design phase. Additionally, on-the-fly screening of prospective materials is critical to exploratory material development workflows. Thus, it is crucial to have inexpensive computational tools capable of characterizing chemical degradation kinetics in advance of costly synthesis and stress testing to provide qualitative guidance on the susceptibility of a material to various stressors. Here, we report the development of an inexpensive scalar surrogate called the Stability Score, which reflects the stressor-specific stability rank order of materials. We use the thermal degradation of alkanes as an exemplar system to set up the methodology and show how we tackled the problem of kinetic data scarcity. Using a model reaction-based approach for reaction condensation and a depth-wise kinetically pruned reaction generation approach for network reduction, thermal degradation pathways with ~1B potential reactions were reduced to computationally tractable 59 DFT level activation barrier calculations and systems of ~1k kinetically relevant reactions. We generated thermal degradation half-lives for 32k alkanes, benchmarked the stability trends with the limited available experimental kinetic data, and derived non-trivial insights from experimental thermodynamic data. Using this half-life data for training and testing, we trained a multi-layer perceptron (MLP) with a modified hinge-loss function to predict a scaled rank order of these half-lives (Stability Score), and compared the performance with a more complex Message Passing Neural Network (MPNN). These models were successful on extrapolation tasks on multiple train-test data splits. We also demonstrate a simple material design problem that uses Stability Scores to guide the search. This work provides first-of-its-kind evidence that kinetic data can be generated in a robust manner to train deep learning models to learn the relative kinetic information between different molecules to predict chemical stressor-specific stability and even extrapolate that information to aid material design. Furthermore, this work sets the initial groundwork for exploring the degradation of more complex chemistries in the future.
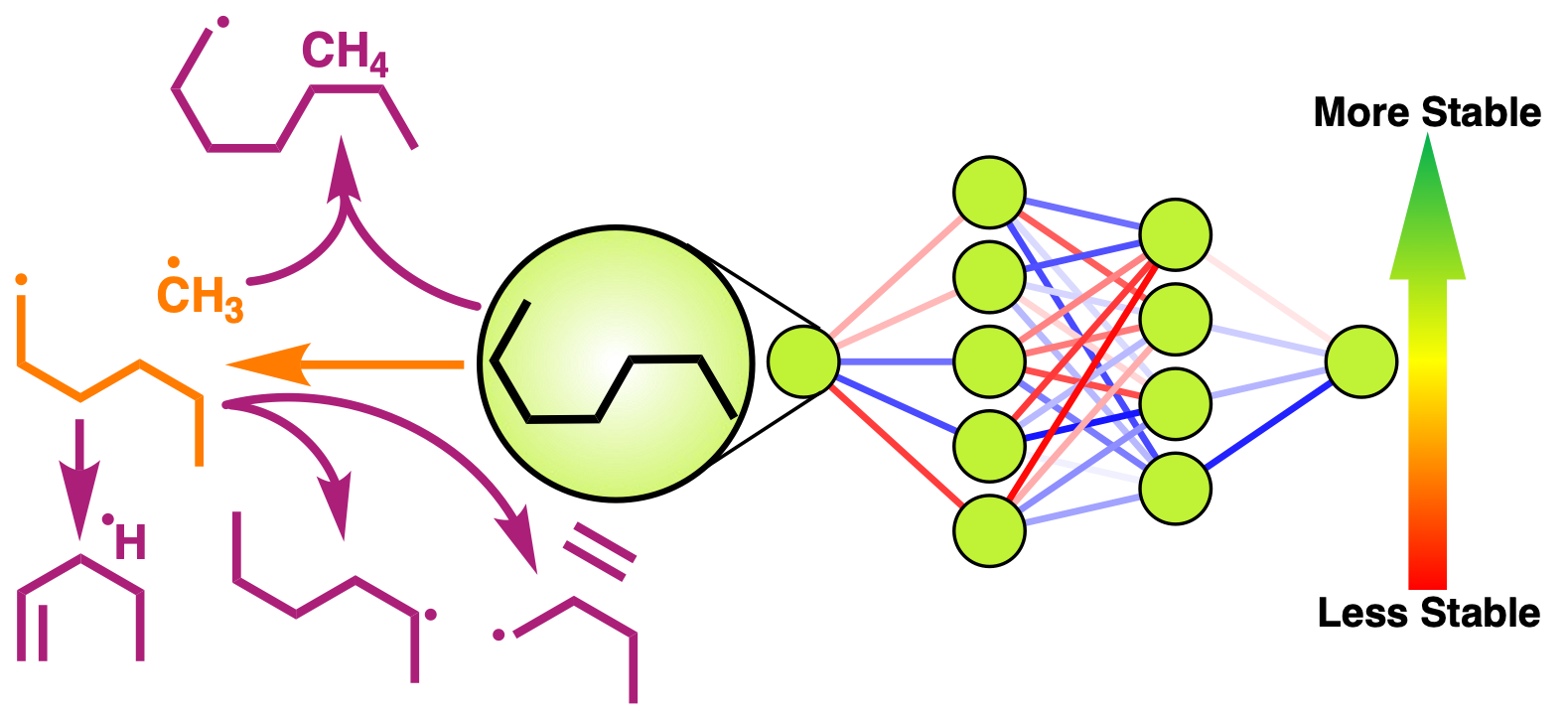
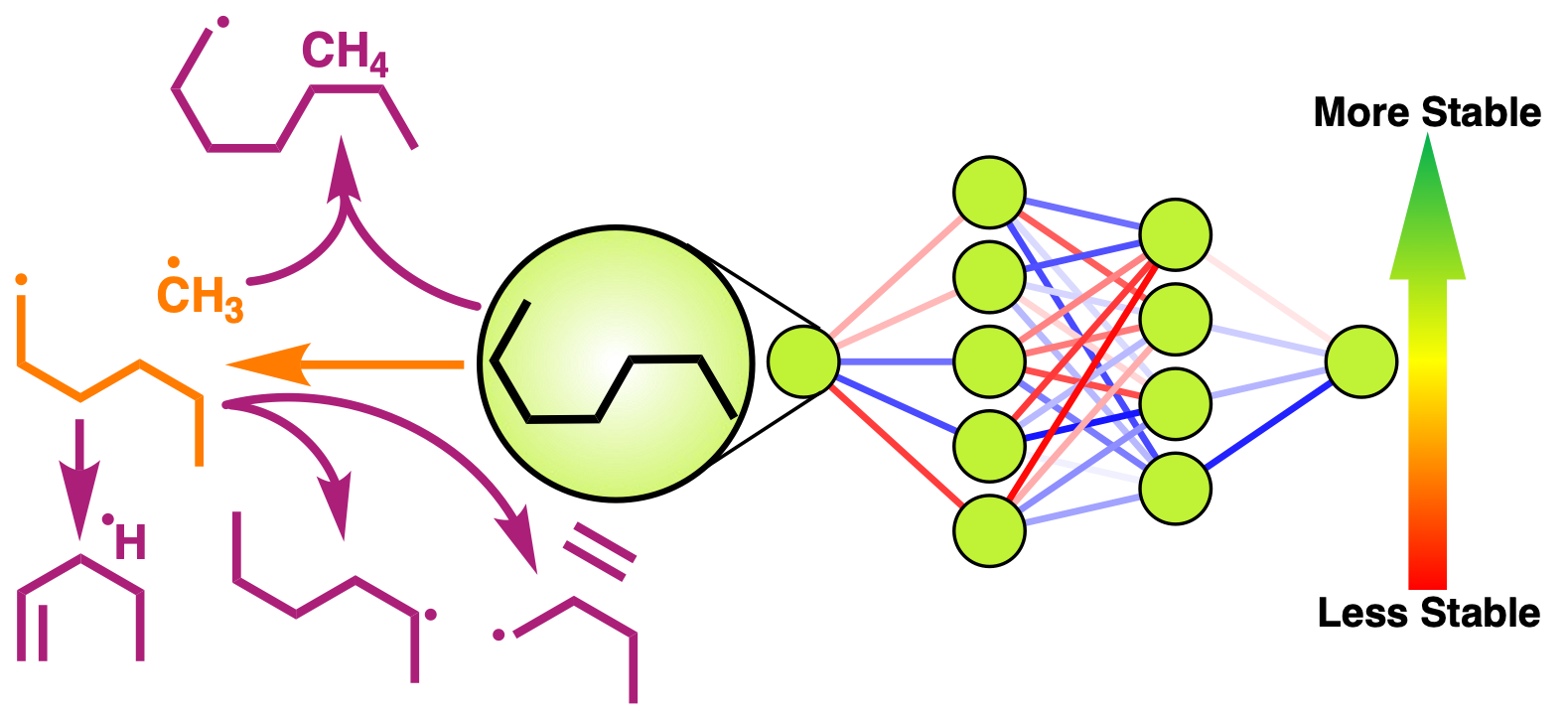