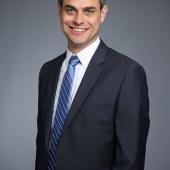
Session Chairs:
- Patrick Heider, The Dow Chemical Company
- Kushal Sinha, Abbvie Inc.
Session Description:
The increasing access to powerful computers and computational tools has enabled the process development community to gain unique insights into the operation of a process. The use of advanced data analytics and modeling with other specialized codes provide a way to multiply the knowledge gained from experiments. This ultimately reduces the time and work required to develop and improve processes making the process engineer more efficient. This session will highlight the use of computational codes to more efficiently address process development challenges or focus on the fundamental problems within a chemical process.
*All session and speaker information is subject to change pending finalization
Schedule:
TIME | PRESENTATION | SPEAKER |
8:55am | Population Balance Modeling Used as a Tool for Process Scale Up | Ben Freireich, PSRI |
9:25am | Computer-Aided ChemicalSynthesis: Progress and Opportunities | Connor Coley, MIT |
9:55am | Chemical Process Data Science for Industry 4.0 | Mark Joswiak, The Dow Chemical Company |
Abstracts:
Population Balance Modeling Used as a Tool for Process Scale Up
Ben Freireich, PSRI
Engineers are born with mass and energy balances as a goto tool, useful for everything from process design and scale up to troubleshooting their dishwasher at home. Some more math hungry engineers may even venture into the space of momentum balances for fluid mechanical calculations. However, few are exposed to population balances. The math can be somewhat intimidating, making conceptual understanding inaccessible. Nevertheless, the tool is useful for grasping complex ideas about distributed quantities (e.g. particle size distributions, age distributions, composition distributions) with almost the same ease as mass and energy balances. In this talk I intend to help overcome the conceptual and mathematical challenges of population balances so that process development engineers may add them to their tool box.
Computer-Aided Chemical Synthesis: Progress and Opportunities
Connor Coley, MIT
Advances in laboratory automation promise to decrease the manual effort of synthesis, but determining how to synthesize a compound continues to require time and effort investment from expert chemists. To achieve full autonomous chemical synthesis, one must have robust synthesis planning software that can propose fully-specified synthetic routes to target molecules. In this talk, I will describe our recent efforts to develop such software. The overarching theme of our work is how to most effectively leverage historical reaction data to inform decision-making in small molecule pathway design.
The overall synthesis planning workflow contains a number of interconnected modules. We focus on two critical aspects of computer-aided synthesis planning and how machine learning and other data-driven techniques have enabled new approaches to both challenges. First, we discuss the problem of retrosynthetic planning (i.e., identification of suitable starting materials) and how the recursive expansion and search strategy are both conducive to machine learning approaches. Second, we discuss the challenge of in silico reaction validation, which can be addressed by solving the inverse problem of forward reaction prediction. We summarize neural network-based approaches we have taken to develop models that can anticipate the products of a chemical reaction after being trained on previously published reactions. Finally, we describe how these techniques for retrosynthesis and forward prediction are integrated into an overall workflow that, for a given molecular target, predicts a rank ordered list of reaction paths that connect the target to purchasable starting materials via a series of plausible reaction steps. The integrated program offers additional features for excluding specific reactions or chemicals, e.g., for IP or toxicity concerns.
Chemical Process Data Science for Industry 4.0
Mark Joswiak, Dow
Digitization, robotics, and artificial intelligence are among the key aspects in the ongoing fourth industrial revolution. In this era of big data and powerful computers, the hype around data analytics is palpable and inescapable. While numerous industries have capitalized on analytics for business intelligence (i.e., targeted sales, marketing, etc.), the process industry has not realized the same level of utilization, in part because the fundamental science that governs the processing industry cannot be ignored. Process data science is typically utilized to improve operational efficiency via, e.g., fault detection, process monitoring, and inferential sensors developed using multivariate latent variable techniques, such as principal component analysis. In this talk, an overview of process data science is discussed along with extensions to other applications. For example, it can elucidate key process variables in root cause analyses, leading to a better understanding of the process and, e.g., an improvement in asset utilization. For these projects to be successful, the first-principles cannot be ignored and their incorporation into process models is vital, which is shown in the development of a hybrid fundamental/data-driven reactor model.