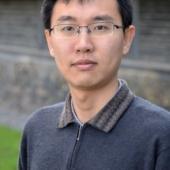
Session Sponsored by M-Star Simulations
*All session and speaker information is subject to change pending finalization
Session Chairs:
- Mathieu Cura, Optimistik
Schedule:
TIME (EDT) | PRESENTATION | SPEAKER |
---|---|---|
7:00 AM | Accelerated Chemical Reaction optimization with Active Learning to build your Reaction Digital Twin | Thomas Galeandro-Diamant, ChemIntelligence |
7:25 AM | A Hybrid Digital Twin of the Sintering Process of Manganese Ore | Haoxue Han, Eramet Ideas; Axel Vansteene, Eramet Ideas |
7:50 AM | Digital Twin Applied on Crystallisation Process | Justine Henriques, Inevo Technologies |
8:15 AM | Numerical Twins of Physical Systems | John Thomas, M-Star |
8:30 AM | Anomaly detection in multivariate process by using machine learning algorithms on time series | Katarina Firdova, Optimistik |
Abstracts:
Accelerated Chemical Reaction optimization with Active Learning to build your Reaction Digital Twin
Thomas Galeandro-Diamant, ChemIntelligence
The optimization of chemical reactions is very often performed using a trial-and-error methodology and modifying one experimental variable at a time. In this presentation, we will show how the active learning methodology is able to reduce the number of experiments required to optimize chemical reactions by building a “digital twin” of the chemical reaction system.
We will present one of the most well-known active learning algorithms: Bayesian optimization. This algorithms combines two strategies (exploration and exploitation) to build a surrogate machine learning model of the chemical reaction experiment-by-experiment. This surrogate model, the “digital twin” of our chemical reaction system, is combined with an acquisition function in order to iteratively and quickly find an optimal combination of reaction parameters.
We will discuss the advantages and challenges of optimizing chemical reactions using Bayesian optimization algorithms and compare this machine learning-based approach with the traditional Design of Experiments (DoE) methodology.
A Hybrid Digital Twin of the Sintering Process of Manganese Ore
Haoxue Han, Eramet Ideas; Axel Vansteene, Eramet Ideas
The enrichment and sintering plant of the Industrial Complex of Moanda (CIM) enables the valorization of Mn-rich fines generated during the production of MMA and MMD ores.
Thanks to the analysis of historical data of the sintering plant, a data-driven Digital Twin of the process was developed. The goal behind the development of this Digital Twin is to precisely mimic the behavior of the industrial process and enable the identification of optimal setpoints in the sintering process.
Certain key parameters of the process, such as the permeability of the load and its temperature during sintering, are nevertheless difficult to measure online and therefore missing in the digital twin. To gain information on these parameters, a First-Principle model of the sintering strand was developed. Such a physics-driven model enriches the Digital Twin to deduce the behavior of the process beyond its conventional operating ranges. Based on kinetics of coke combustion, thermodynamics of the sintering reaction, and aeraulics of the gas flow within the load, the advance of the flame front and the temperature profile in the load were simulated. When run in an inverse manner coupled to an optimization algorithm, the model enabled the calculation of the load’s permeability. Thereafter, the permeability values were used to enrich the Digital Twin.
The Digital Twin and the physical model have thus been interconnected. This provides a powerful tool for optimizing CIM performance. In the future, this digital tool developed at Eramet Ideas will be deployed in the plant. On-site missions will allow us to receive user feedback and work directly on improving the user interface.
Digital Twin Applied on Crystallisation Process
Justine Henriques, Inevo Technologies
Crystallization is a complex process which is consisting in forming crystals from a fluid. Industrial crystallization encounters malfunction problems: solid wall deposition, mechanical issues, sensor measurement errors of sensors, etc.
With the Industry 4.0, it could be possible to get a better understanding of industrial crystallizers using a digital twin. The digital twin estimate in real time key parameters for process performance and design. This calculated data can be compared with measurements, design requirements and product specifications to optimize operating conditions and anticipate dysfunctions.
Numerical Twins of Physical Systems
John Thomas, M-Star
In many agitated tanks and fluid handling systems, yield and performance is governed by complex turbulent fluid mechanics. These turbulent motions, which can inform both bulk transport and reaction kinetics, occur over a large range of length and time-scales. From a modeling perspective, direct numerical simulation of this entire turbulence spectrum is required to obtain complete insights into systems performance. Due to time and resource constraints, however, such detailed simulations have not historically been practical within most industrial settings.
In this work, I will show how graphical processing units (GPUs) can make direct numerical simulation (DNS) of industrial mixing systems practical and timely. Although GPUs have historically been used for image rendering, in recent years they have emerged and as computationally superior computing paradigm relative to traditional CPUs. We will begin the presentation by introducing the concepts governing fluid modeling on GPUs. We then show how, given identical algorithms, a single scientific GPU can execute simulations two to three orders-of-magnitude faster than a single CPU. We then present various criteria for monitoring the convergence from large-eddy simulation (LES) to direct numerical simulation, within the context of the turbulent fluid motion. We then apply these criteria to study blending, energy dissipation, and reaction rates in a benchtop reactor.
Anomaly detection in multivariate process by using machine learning algorithms on time series
Katarina Firdova, Optimistik