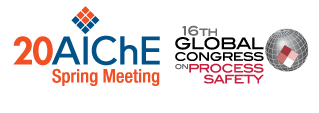
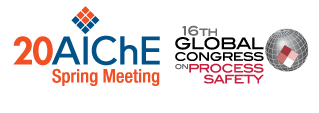
Liquid heat capacity (LCP) is another property where QSPRs can be helpful prediction tools. Researchers have shown strong correlation between the LCP and various molecular descriptors, but these predictions are limited to a single temperature, usually 298.15 K. Engineers often need LCP prediction methods that can be extrapolated through a large temperature range for safe process design, and currently available QSPR methods for LCP lack this ability. Another important consideration is the steep increase in heat capacity as the liquid nears its critical temperature, an issue that is not accounted for in traditional polynomial-based predictions, such as the Ruzicka-Domalski group contribution method.
In this work, multiple QSPRs at various temperatures are combined using a modified corresponding states approach. LCP data was compiled or collected at several temperatures and QSPR predictions were made at each temperature point. Then, using statistical analysis to combine uncertainties, a modified corresponding states method was used to link the individual QSPRs while maintaining the known behavior as the critical point is approached.
This method improves on existing QSPRs for LCP by using unique descriptors and adding a temperature dependence that has not been previously implemented. This functionality gives process engineers a thermodynamically rigorous prediction method that can be used to extrapolate up to the critical temperature.
Presenter(s)
Language
Pricing
Individuals
AIChE Member Credits | 0.5 |
AIChE Pro Members | $19.00 |
AIChE Graduate Student Members | Free |
AIChE Undergraduate Student Members | Free |
RAPID Members | Free |
AIChE Explorer Members | $29.00 |
Non-Members | $29.00 |