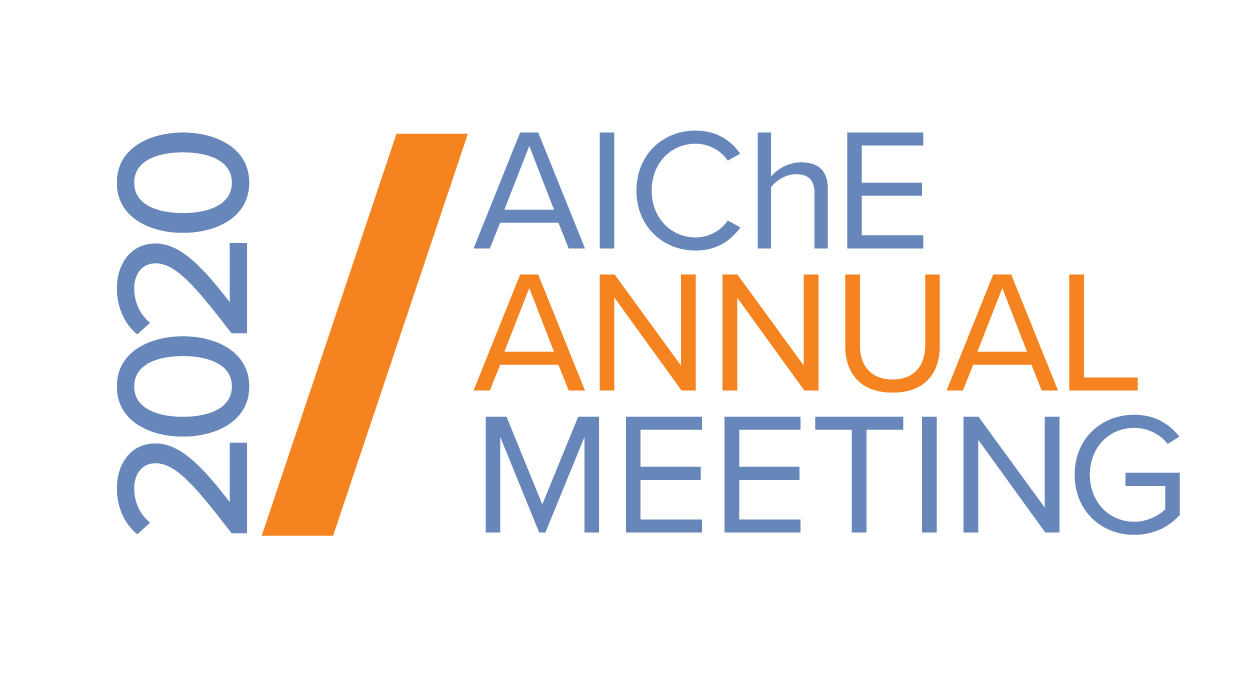
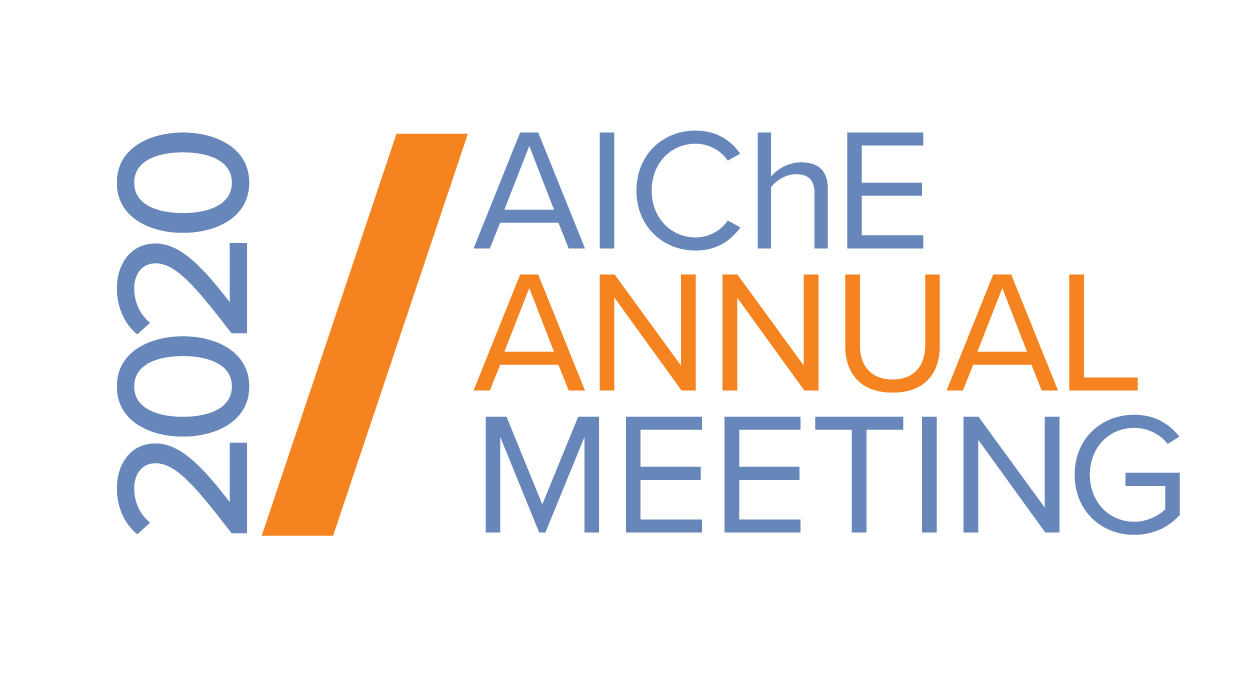
The AI methods that focuses on the use of large and diverse data sets in training new potentials, has consistently proven to be universally applicable to systems containing the atomic species in the training set. Focusing on parametrization for organic molecules (with CHNOSFCl atoms so far), we have developed a universal neural network potential which is highly accurate compared to reference QM calculations at speeds 107 faster. The potential is shown to accurately represent the underlying physical chemistry of molecules through various test cases including: chemical reactions (both thermodynamics and kinetics), thermochemistry, structural optimization, and molecular dynamics simulations. The results presented in this talk will provide evidence of the universal applicability of deep learning to various chemistry problems involving organic molecules.