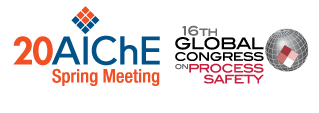
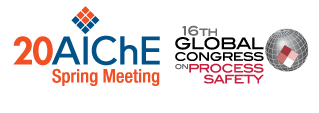
Pipeline system with hazardous liquid has spread for 0.2 billion miles, caused 3000 incidents, and resulted in an economic loss of 3 billion, only in USA [1]. To reduce this loss, causation analysis of pipeline failure using past incident data has been in practice for major causes, such as third-party interference, corrosion and material failure since more than two decades [2]. However, existing methods for utilizing information about past failures are not able to fully capture the nonlinear interaction among causes and their contribution to the pipeline failures. Hence, there is a need to understand the synergy among causes and their collective ability to affect the integrity of the pipeline. To address this challenge, this work proposes an Artificial Neural Network based causal reasoning model for pipeline integrity.
Artificial Neural Network (ANN) learns the cause-effect relationship between the pipeline failure causes and the failure by considering examples from past incidents. A large number of ANN application have been utilized for pattern learning and classification in various fields [3]. Another advantage of ANN is that that it does not oversimplify the causal relationships leading to identification of both linear and non-linear components of causality. Additional advantage of ANN is its non-imposition of restriction on the input variables (such as their distribution). It lead to its ability to learn hidden relationships making full use of vast database of pipeline incidents. The present work utilizes the advantages of ANN to build a causal representation of pipeline failure. The new technique has been demonstrated using PHMSA database of hazardous liquids.
Keywords: Pipeline integrity, incident data, artificial neural network, causal network
References:
- Pipeline Incident 20 Year Trends, in: Pipeline and Hazardous Materials Safety Administration (PHMSA), https://www.phmsa.dot.gov/data-and-statistics/pipeline/pipelineincident-... (Accessed 4/19/2018), 2018.
- P. Hopkins, âTransmission pipelines: how to improve their integrity and prevent failures,â in Pipeline Technology, Proceedings of the 2nd international pipeline technology conference, R. Denys, Ed., vol. 1, pp. 683â702, 1995.
- R. Sun, A Neural Network Model of Causality. IEEE transactions on neural networks, 1994, 5
Presenter(s)
Language
Pricing
Individuals
AIChE Member Credits | 0.5 |
AIChE Pro Members | $19.00 |
AIChE Graduate Student Members | Free |
AIChE Undergraduate Student Members | Free |
Computing and Systems Technology Division Members | Free |
AIChE Explorer Members | $29.00 |
Non-Members | $29.00 |