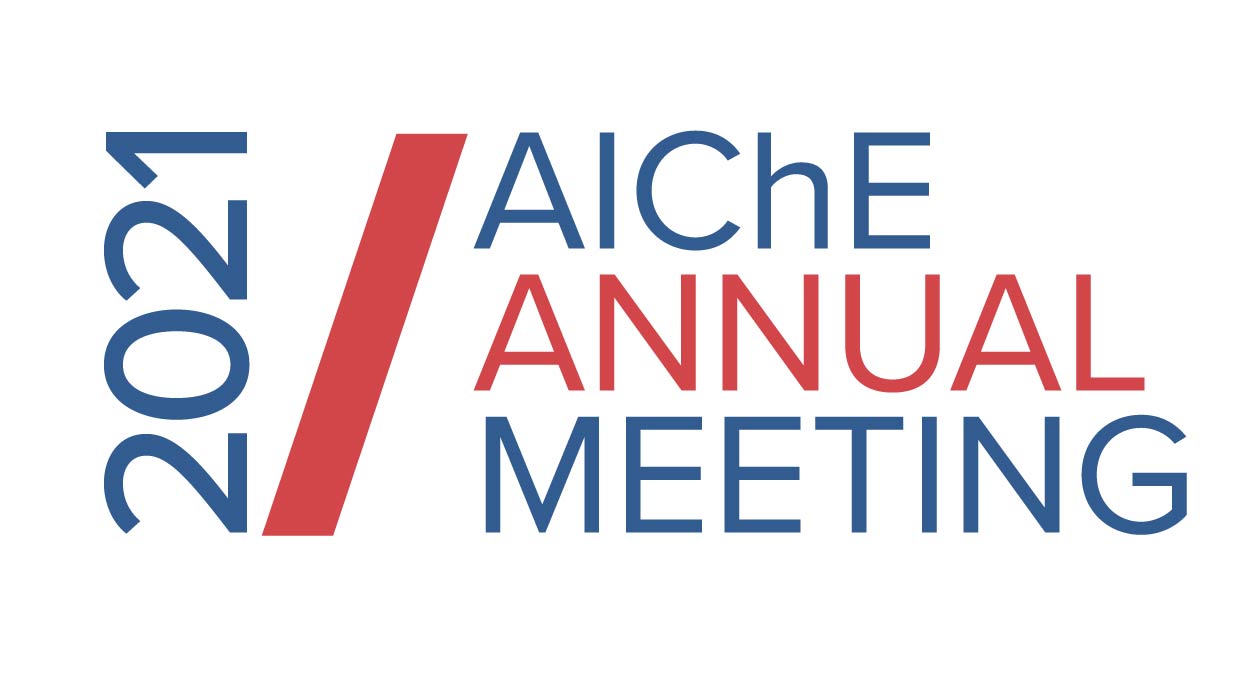
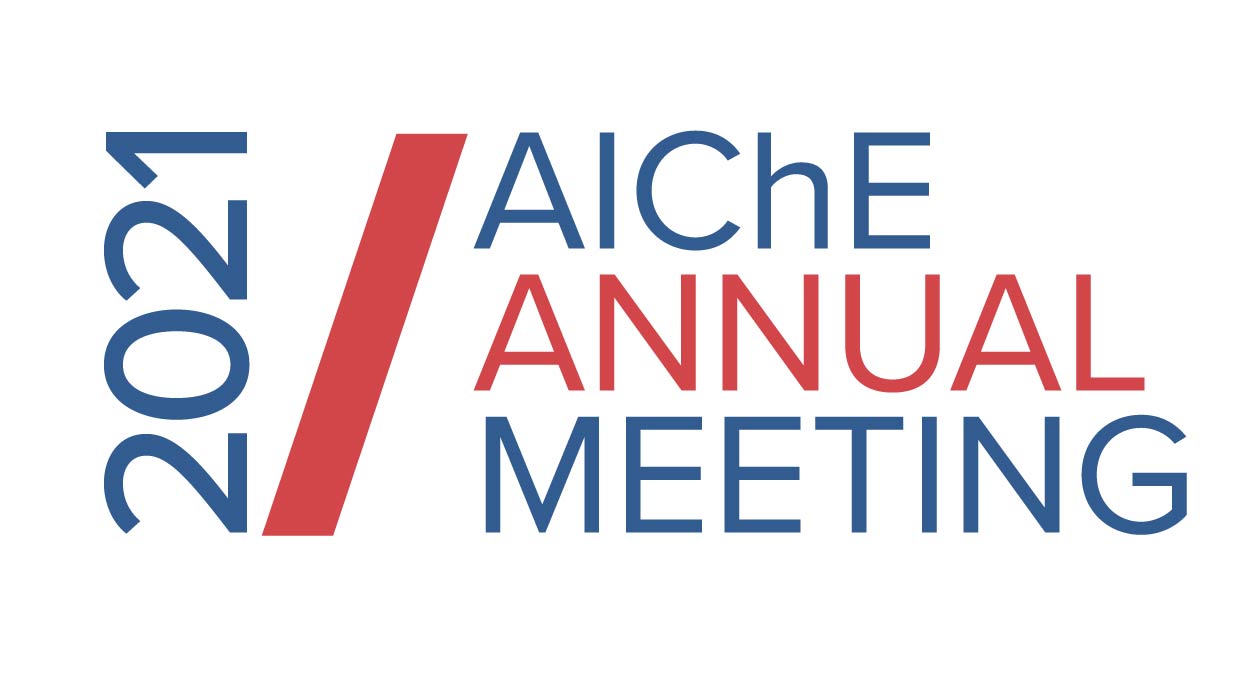
Here, we present a computational framework to characterize supported single-atoms and subnanometer clusters from adsorbate vibrational excitations determined from IR spectroscopy. We combine data-based approaches with physics-driven surrogate models to generate realistic synthetic IR spectra from first-principles vibrational calculations of carbon monoxide on palladium clusters supported on ceria. Due to the relative expense of first-principles vibrational calculations, their direct use for exploring the vast combinatorial space to directly match experimental spectra is beyond our current computational capabilities. Rather, we utilize calculations of energetically viable structures and apply realistic peak broadening to generate single-cluster primary spectra, analogous to pure component spectra in gas-phase IR spectroscopy. Finally, we perform peak deconvolution of complex synthetic and experimental spectra under the Bayesian Inference framework to predict cluster size distributions and quantify uncertainty. We discuss extensions of this computational methodology for characterization of other complex materials under realistic working conditions.