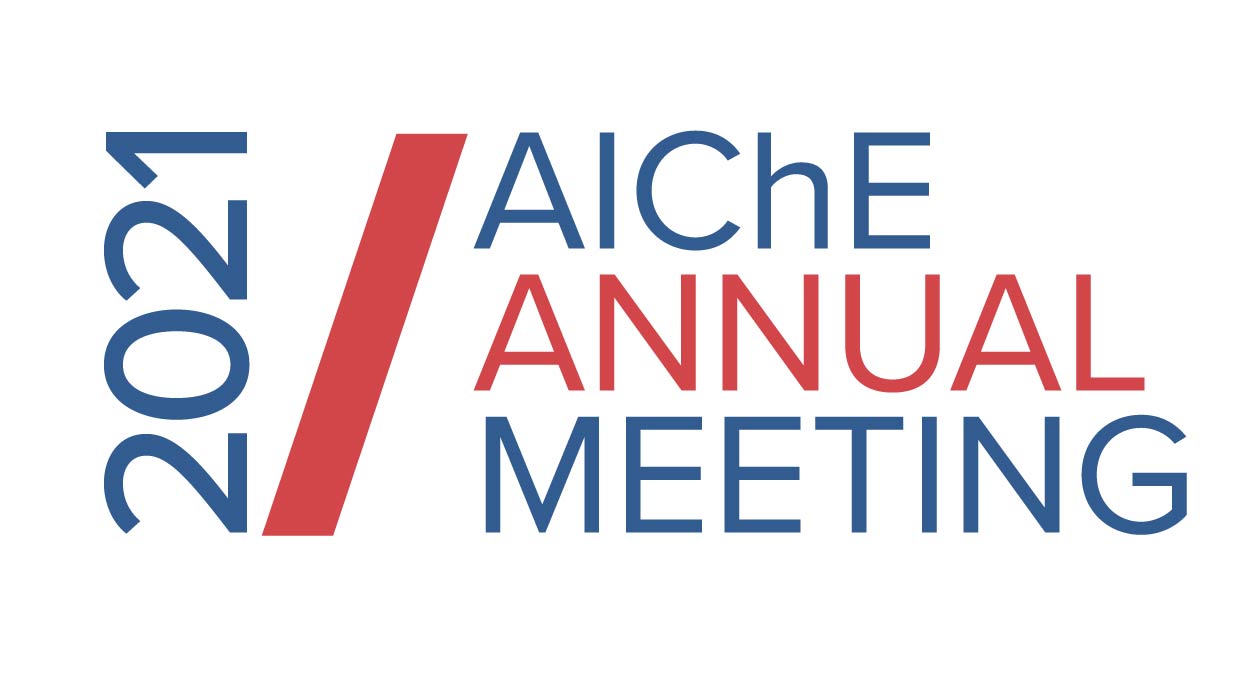
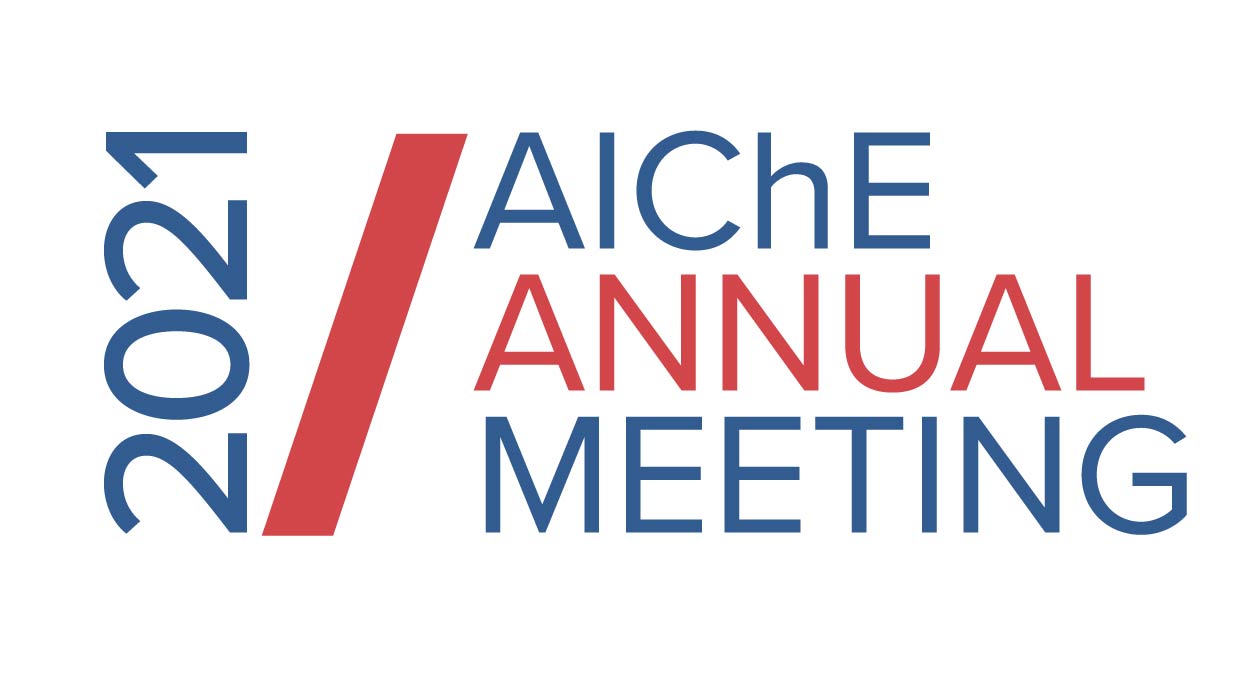
The symmetric diblock copolymer that self-assembles into a lamellar phase can be considered as a prototype for this material class.
The simulation techniques for this system have been perfected over the last decades.
And it is now possible to predict experimental results with these models on short to medium scales accessible.
However, afterward, the morphologies remain stuck in the rugged free-energy landscape.
Evolving in time to overcome the free-energy barriers is even with modern supercomputers untractable.
We present a machine learning model that is trained by medium-time scale simulations of a soft, coarse-grained model to simulate the defect kinetics in the lamellar morphology.
We are exploiting the physical characteristics of overdamped dynamics to formulate the problem of time evolution as a Markov chain.
The trained artificial neural network (ANN) predicts a time-independent transition probably from one time step to the next.
As a result, we obtain a method that can repeatedly apply these mechanisms to obtain very long-time trajectories.
By design, we can choose very large time steps for this evolution.
Predicting the defect kinetics this way with our ANN enables insights into the late-time dynamics.
Unique about this approach is that we can generate the training data fully in silico, which ensures good data quality - the minimum requirement for successful machine learning.
Even as the data generation is computationally costly, we can demonstrate that our approach easily breaks even with the upfront costs.
We specifically design the neural network to be independent of the input size, which enables training on smaller systems, while predictions can be inferred on very large scales.
The combination of being able to predict very large time steps and for large systems enables desktop computers to make predictions on an engineering length scale.
Hence, these techniques open the avenue for fast prototyping in practical applications.