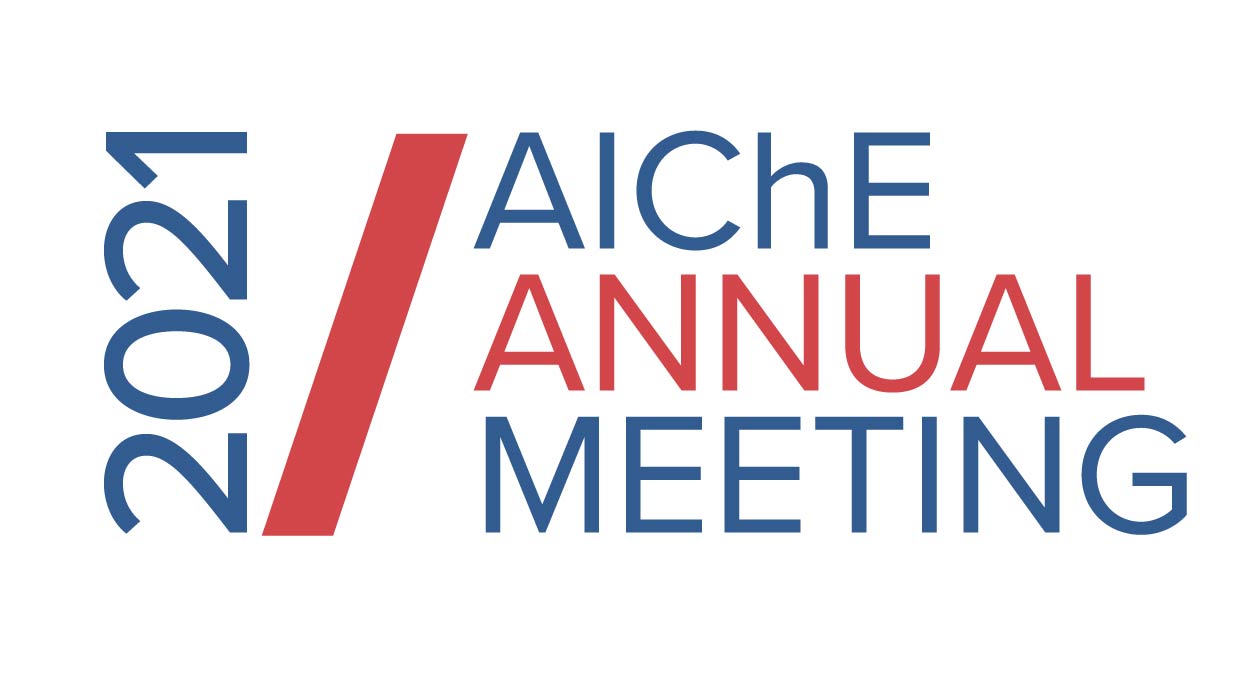
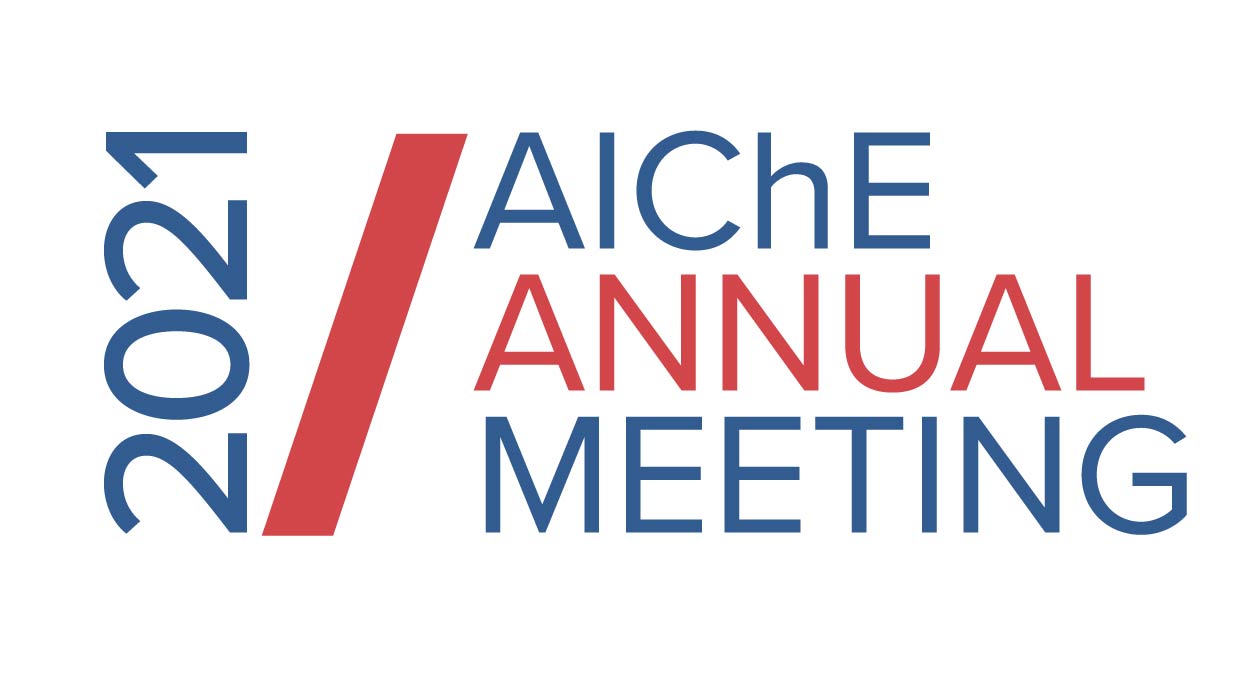
Whether the frontier approach is successful or not is significantly controlled by the reliability and accuracy of input database. It is true that substantial amounts of the data are come by previous literatures and often ab-initio calculations with idealized model systems. The conditions in which the data were generated may be so different from the operando circumstances of the target materials. To secure extreme-level integrity of the database the in-situ measurement of nanoparticle structures should be carried out, from which the reliable correlation of the structure-performance-design principle can be identified.
Using first-principles calculations we studied nanoparticles with adsorbate ligands in liquid solution to establish three-dimensional (3D) structure and property database, which are, then, analyzed through AI-based neural-network approach with high speed and accuracy. The information includes sizes, lattice distortions, and defects with picometer resolution under non-vacuum conditions. The computational outcomes are rigorously validated from the 3D liquid-cell electron microscopy. The approach is indeed âknowledge-basedâ AI, which can be expected to make groundbreaking ways toward the quantum nanoarchitecture for hybrid interface materials.