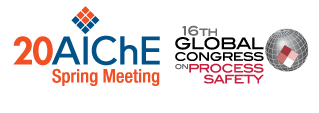
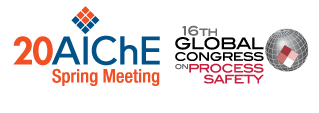
In this work, we explore the application of a deep reinforcement learning controller to the batch crystallization of the sodium chloride, water, ethanol anti-solvent system. The ultimate objective of the controller is to obtain a target distribution of crystal size at the end of the batch by manipulating the temperature of the system and flow rate of the anti-solvent. To do this, we implement a deep neural-network based controller with an actor-critic structure that is trained to maximize the long term reward at each sampling time. The network is trained on a Fokker-Planck model whose parameters are estimated by dynamic optimization, and the network learns in this environment by exploration. We evaluate the performance of the controller against baselines established by classical control techniques and finally analyze the advantages and disadvantages of this framework.
Presenter(s)
Language
Pricing
Individuals
AIChE Member Credits | 0.5 |
AIChE Pro Members | $19.00 |
AIChE Graduate Student Members | Free |
AIChE Undergraduate Student Members | Free |
Computing and Systems Technology Division Members | Free |
AIChE Explorer Members | $29.00 |
Non-Members | $29.00 |