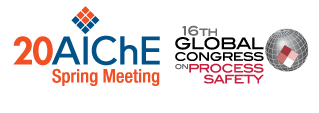
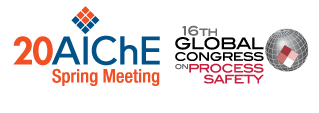
Predicting the expected magnitude of the damage in advance determines the success or failure of the initial response strategy in the event of an accident. This data analytics study is based on the fire accident and response data of the National Fire Service of Korea, accumulated over the last 11 years (2008-2018). We train a deep neural network that inputs about 40 values including common recorded information for all accidents and outputs expected property damage and casualties. Principal component analysis (PCA) also derives the major factors that contribute significantly to the prediction of damage scale among 40 input values. In particular, various machine learning methods are explored to develop the possibility of developing predictive models among the major factors in the investigation report, such as occurrence date, place, casualty, human injury, property damage, duration of suppression, ignition heat source, ignition factor, suppression equipment, and weather conditions. In the Industry 4.0 era, the result of this predictive analytics study suggests the preemptive intelligent use of the collective cause investigations beyond the standardized damage investigation and the findings reporting, obtained after nationwide expending of high cost for analysis of fire incidents.
Presenter(s)
Once the content has been viewed and you have attested to it, you will be able to download and print a certificate for PDH credits.
If you have already viewed this content,
please click here
to login.
Language
Pricing
Individuals
AIChE Member Credits | 0.5 |
AIChE Pro Members | $19.00 |
AIChE Graduate Student Members | Free |
AIChE Undergraduate Student Members | Free |
Computing and Systems Technology Division Members | Free |
AIChE Explorer Members | $29.00 |
Non-Members | $29.00 |