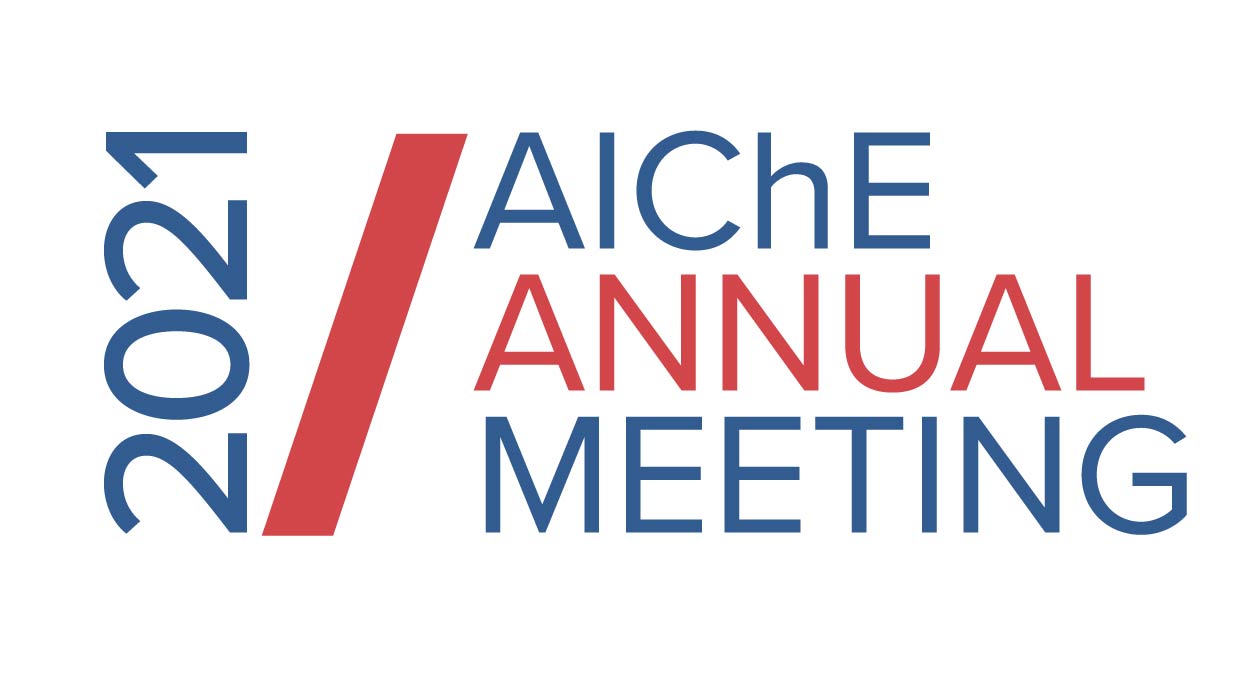
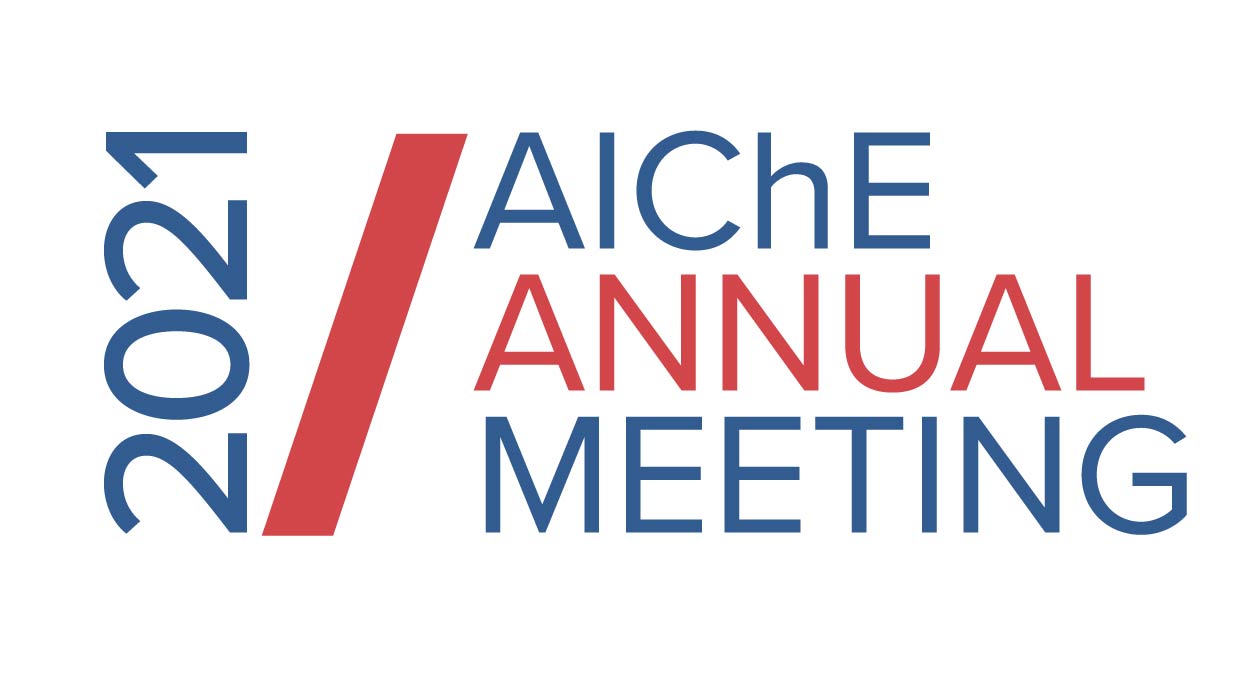
We developed and implemented a data-assisted procedure for parametrizing input-output relations [1]. Our suggested approach aims at parameter reduction in problems where the effective inputs are (possibly nonlinear) combinations of several original model inputs (parameters). Our data-driven approach is based on the manifold learning algorithm Diffusion Maps [2], the extension scheme Geometric Harmonics [3] and is assisted by deep learning (a conformal autoencoder neural network). The relations we considered in this work arise from coupled first order nonlinear differential equations describing the time-evolution of chemical/biochemical reaction networks. More precisely we considered two toy examples with three original inputs each and a model that describes the multisite phosphorylation with 6 original inputs total [4]. In our workflow, for each example, we collect a data set of output behaviors of the system and apply output informed Diffusion Maps. Diffusion Maps achieves parameter reduction and the new discovered machine learning effective parameters are given to us as eigenvectors of the Diffusion Maps algorithm. We establish in a data-driven way the physical interpretability of these ''effective parameters" . These can be used to infer the parameters without optimization: for new, unobserved system behaviors we obtain the values of the data-driven and analytical effective parameters corresponding to those behaviors without running an optimizer. We also illustrate the ability of our scheme to map from new analytical effective parameters to systems' behaviors without the simulations. Utilizing our Y-shaped conformal autoencoder neural network allows us to go beyond discovering the effective paremeters, to parametrizing the level sets of the parameter combinations that do not affect the response. Lastly, we illustrate that Jointly Smooth Functions [5] provide another possibility to construct data-driven effective parameters.
[1] Alexander Holiday, Mahdi Kooshkbaghi, Juan M Bello-Rivas, C William Gear, Antonios Zagaris, and Ioannis G Kevrekidis. Manifold learning for parameter reduction. Journal of Computational Physics, 392:419â431, 2019
[2] Ronald R Coifman and Stephane Lafon. Diffusion maps. Applied and Computational Harmonic Analysis, 21(1):5â30, 2006.
[3] Ronald R Coifman and Stephane Lafon. Geometric harmonics: A novel tool for multiscale out-of-sample extension of empirical functions. Applied and Computational Harmonic Analysis,21(1):31â52, 2006
[4] Eyan Yeung, Sarah McFann, Lewis Marsh, Emilie Dufresne, Sarah Filippi, Heather A Harrington, Stanislav Y Shvartsman, and Martin Wuhr. Inference of multisite phosphorylationrate constants and their modulation by pathogenic mutations. Current Biology, 30(5):877â882,2020.
[5]Or Yair, Felix Dietrich, Rotem Mulayoff, Ronen Talmon, and Ioannis G. Kevrekidis. Spectraldiscovery of jointly smooth features for multimodal data.arXiv pre-print: 2004.04386, April2020

[1] Alexander Holiday, Mahdi Kooshkbaghi, Juan M Bello-Rivas, C William Gear, Antonios Zagaris, and Ioannis G Kevrekidis. Manifold learning for parameter reduction. Journal of Computational Physics, 392:419â431, 2019
[2] Ronald R Coifman and Stephane Lafon. Diffusion maps. Applied and Computational Harmonic Analysis, 21(1):5â30, 2006.
[3] Ronald R Coifman and Stephane Lafon. Geometric harmonics: A novel tool for multiscale out-of-sample extension of empirical functions. Applied and Computational Harmonic Analysis,21(1):31â52, 2006
[4] Eyan Yeung, Sarah McFann, Lewis Marsh, Emilie Dufresne, Sarah Filippi, Heather A Harrington, Stanislav Y Shvartsman, and Martin Wuhr. Inference of multisite phosphorylationrate constants and their modulation by pathogenic mutations. Current Biology, 30(5):877â882,2020.
[5]Or Yair, Felix Dietrich, Rotem Mulayoff, Ronen Talmon, and Ioannis G. Kevrekidis. Spectraldiscovery of jointly smooth features for multimodal data.arXiv pre-print: 2004.04386, April2020

Presenter(s)
Once the content has been viewed and you have attested to it, you will be able to download and print a certificate for PDH credits.
If you have already viewed this content,
please click here
to login.