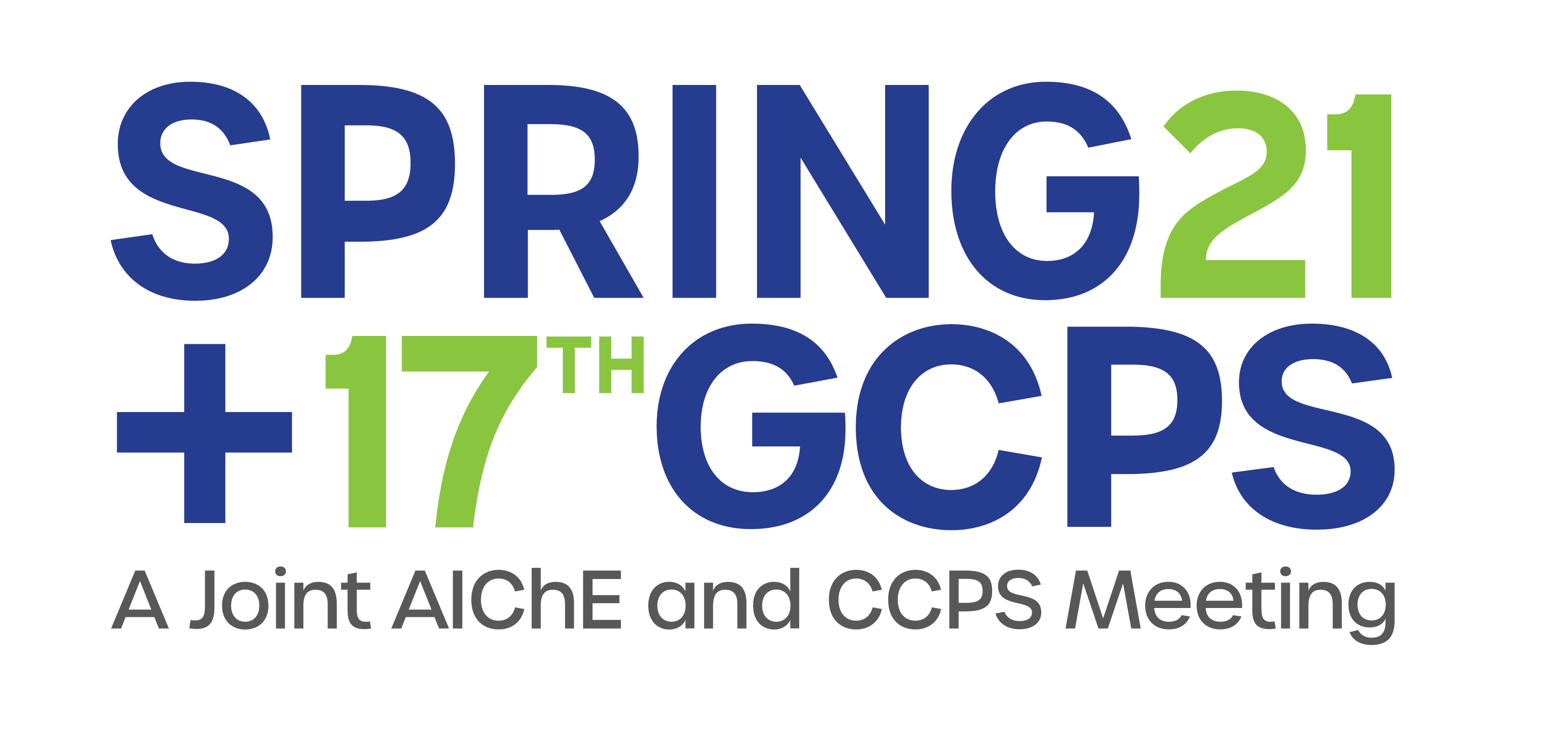
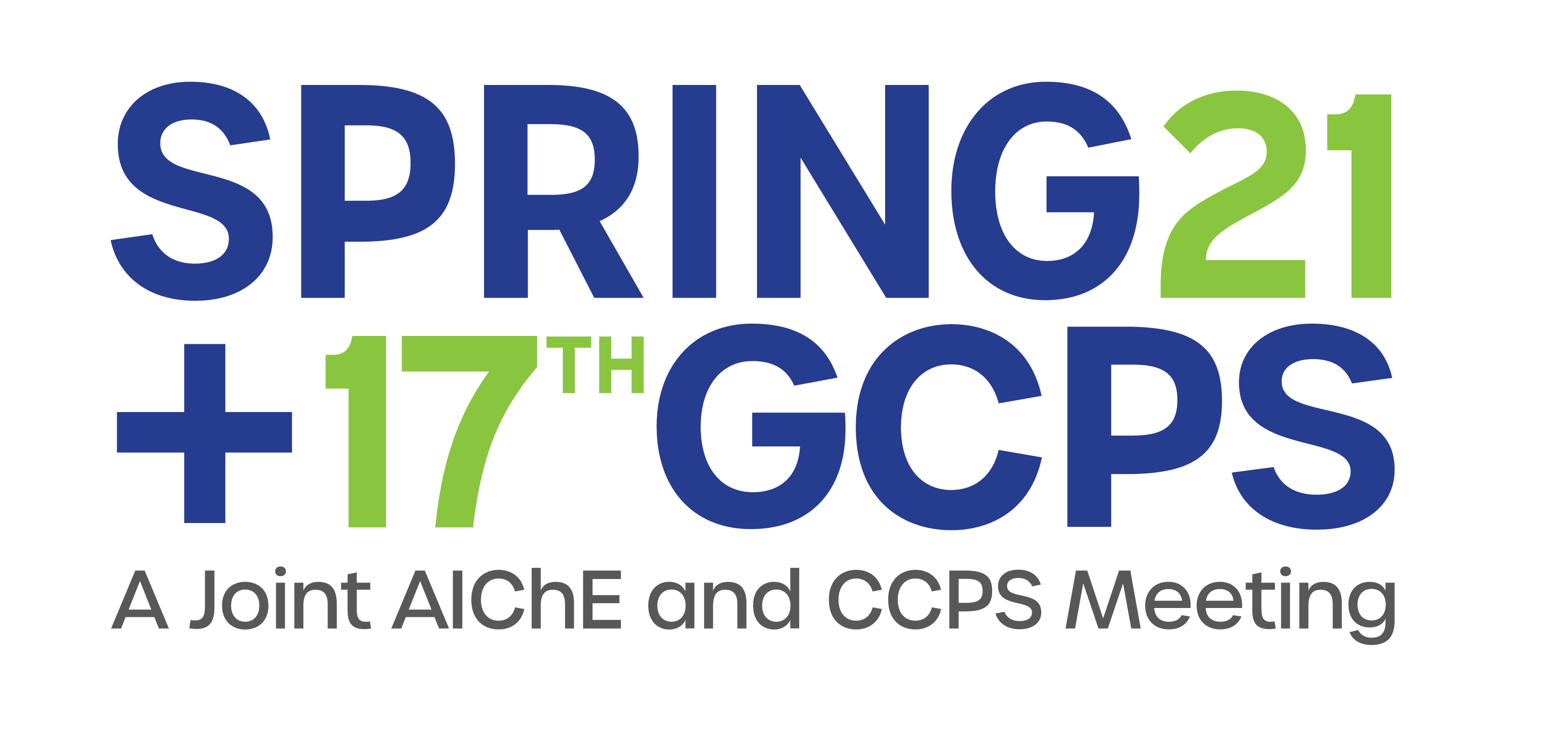
Adrien Spagnol1, Kevin Jaunatre1, Gabriel Ducret2, Pascal Duchene1, Denis Guillaume1, Vinay Jain1 and Benoit Celse1
1 IFP Energies Nouvelles, Rond-Point de lâEchangeur de Solaize, 69360 Solaize, France
2 IFP Energies Nouvelles, 1-4 Avenue du Bois Préau, 92852 Rueil-Malmaison, France
*E-mail: benoit.celse@ifpen.fr
HIGHLIGHTS
- Comparison between different data-driven approaches on industrial dataset.
- Linear regression techniques provide easy-to-explain models.
- Validation of the selected models on chosen cycles, with discussion regarding the possible limitations.
INTRODUCTION
More than 95% of all the chemical products are nowadays produced using a catalyst, which shows the high importance of catalysis for all value chains in the chemical industry, especially in petroleum refineries. In that scope, catalysis deactivation as an interdisciplinary technology field is of strategic socio-economic importance, since it is a considerable and enduring problem when operating industrial catalytic processes. Thus, being able to predict the deactivation of the catalysts is a primary concern.
From the statistical point of view, correctly predicting the deactivation phenomenon in an industrial environment is a challenging task due complex chemical processes and affecting factors such as reactor configuration, quality of feed oils, the catalyst degradation, and greatly varying operating conditions.
Based on collected data from different petrochemical refineries with varying capacities, feedstockâs, and operating conditions, see Table 1 below, the main objective of this work is to build a predictive model of the catalyst deactivation for Vacuum Gas Oil (VGO) Hydrotreatment (HDT) in order to correctly forecast the cycle duration.
Number of refineries |
Number of cycles |
Average length (in days) |
12 |
21 |
830 |
Table 1. Summary of the collected data from petrochemical refineries
MATERIAL & METHODS
Two kind of models are tailored depending on different scenarios. In a first place, the Design configuration, where the goal is to predict the duration of a cycle based on a launch setup, assumed constant over the whole life of the catalyst. In a second place, a What-if configuration, whose goal is to forecast future deactivation,
Prior to any modeling, the preprocessing of the data is detailed, in order to underline the importance of the quality of the used datasets, correct existing caveats from measured information and propose improvements. Different type of models are discussed in this work, either regression-based approaches, which build a relationship between historical data and the catalyst deactivation rate, or time-series strategies, since the catalyst deactivation phenomenon has a time-dependency.
For regression strategies, we explore many available options from the literature, from multilinear regression, possibly including a penalization term [1][2], to nonlinear approaches [3,4]. For time-series techniques, we test simpler approaches [5] and complex models including regressors [6].
RESULTS & DISCUSSIONS
All strategies are discussed, listing the different pros and cons of each. A comparison is done using a calibration-validation process in order to rank each technique based on their quality of prediction, for both scenarios.
Best selected approaches are used on experimental cycles to assess the benefits of being able to predict catalyst deactivation, compared to the classical approach used on refineries based on a straightforward time extrapolation of the weighted average bed temperature (WABT).
Furthermore, building a input-output model allows to optimize the economics of the refineries by offering insights on how to conduct the end of a given cycle, based on the predictions (i.e. showing if it is reasonable to consider different feeds, possibly harder to treat).
KEYWORDS
Catalyst deactivation, regression techniques, time-series approaches, Vacuum Gas Oil Hydrotreatment
BIBLIOGRAPHY
1. Robert Tibshirani, "Regression shrinkage and selection via the lasso," Journal of the Royal Statistical Society: Series B (Methodological), vol. 58, no. 1, pp. 267-288, 1996. |
2. Hui Zou and Trevor Hastie, "Regularization and variable selection via the elastic net," Journal of the Royal Statistical Society: Series B (Statistical Methodology), vol. 67, no. 2, pp. 301-320, 2005. 3. Alex J. Smola and Bernhard Schölkopf, "A tutorial on support vector regression," Statistics and computing, vol. 14, no. 3, pp. 199-222, 2004. 4. Leo Breiman (2001). Random forests. Machine learning, 45(1), 5-32. 5. Everette. S. Gardner, "Exponential smoothing: The state of the art," Journal of Forecasting, vol. 4, no. 1, pp. 1â28, 1985. 6. George Athanasopoulos Rob J Hyndman, Forecasting: Principles and Practice, OTexts, Ed., 2018. |
Presenter(s)
Language
Pricing
Individuals
AIChE Member Credits | 0.5 |
AIChE Pro Members | $19.00 |
AIChE Graduate Student Members | Free |
AIChE Undergraduate Student Members | Free |
Computing and Systems Technology Division Members | Free |
AIChE Explorer Members | $29.00 |
Non-Members | $29.00 |