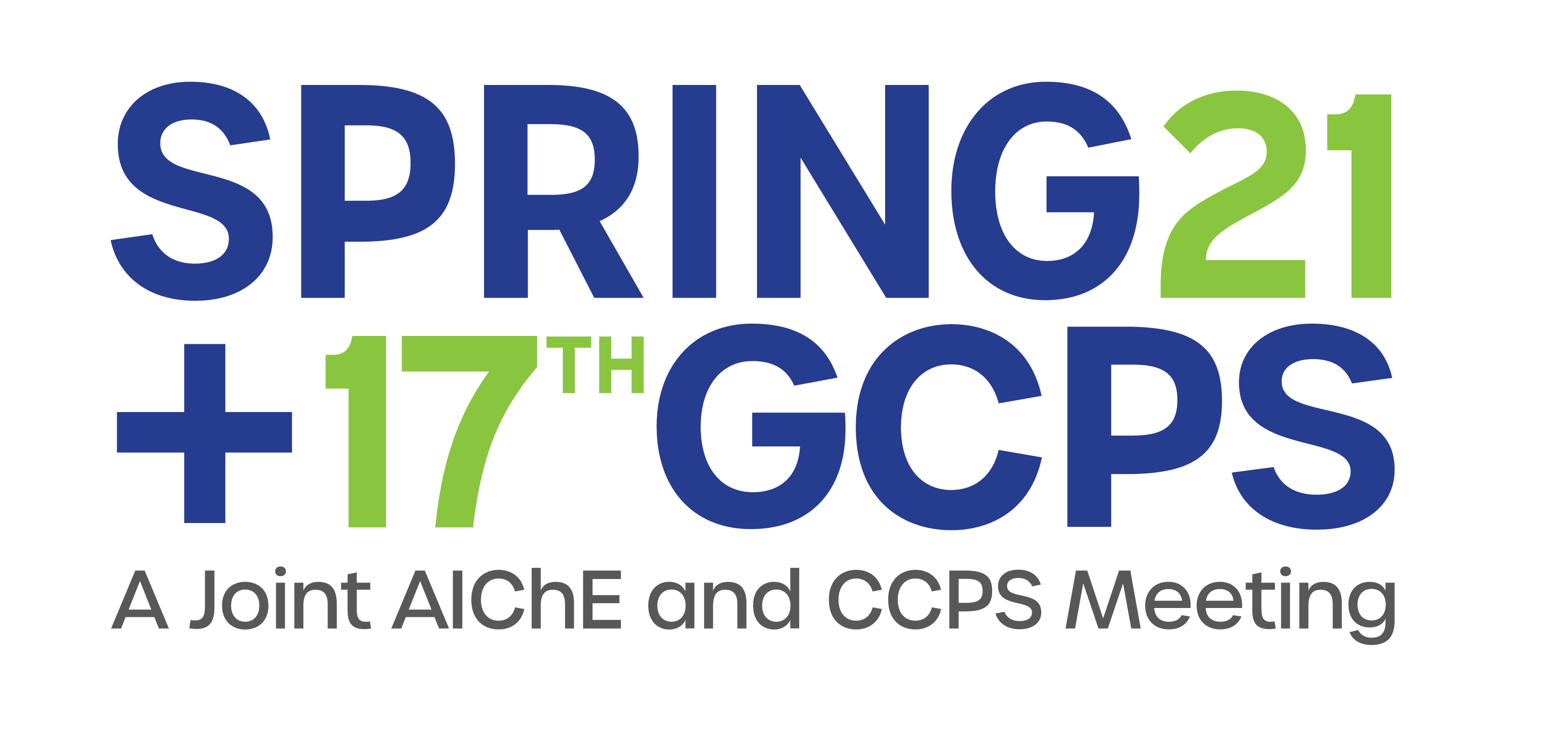
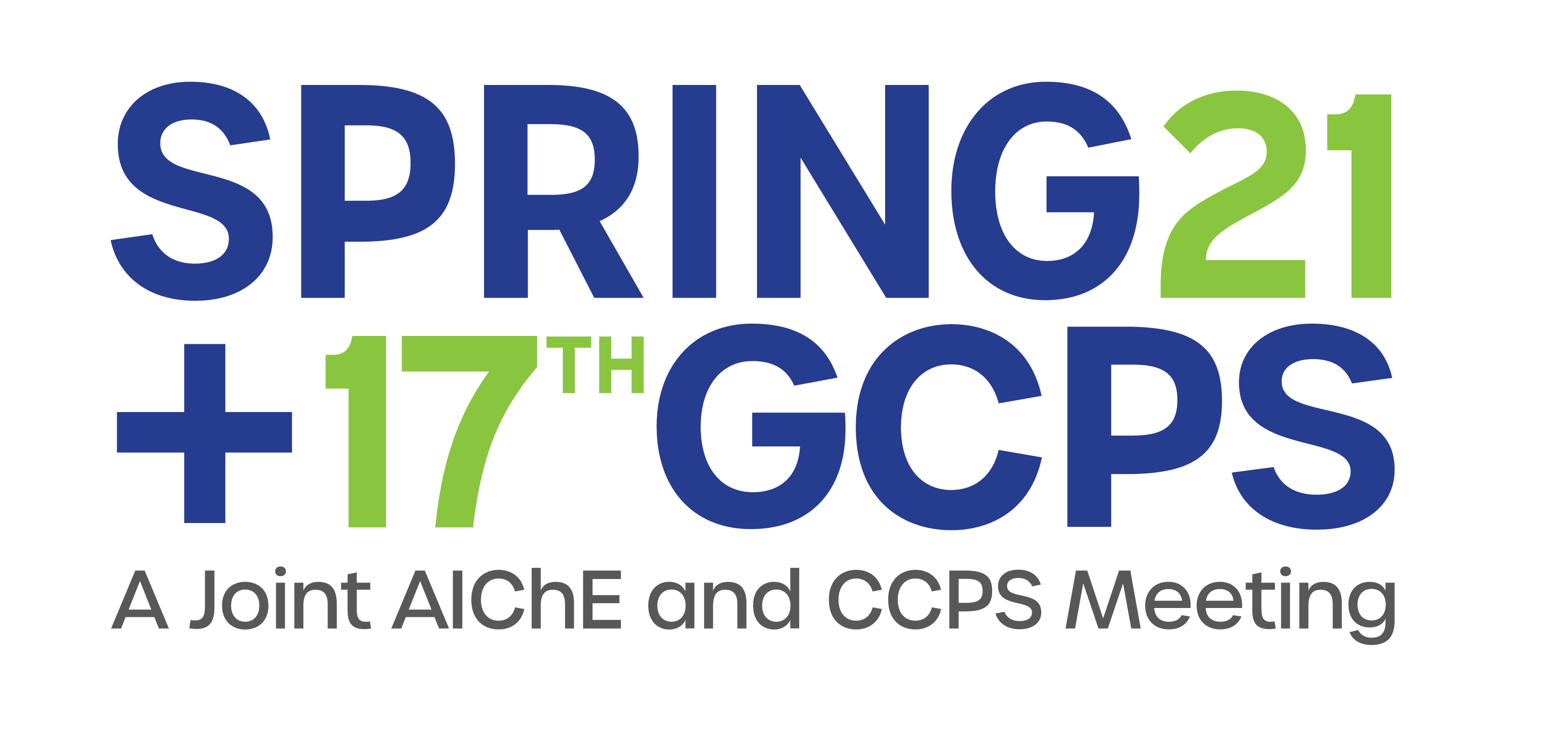
The petroleum facilities
require large amount of water for the cooling, boiler feed, processing,
sanitary services, fire protection, and many other miscellaneous purposes. Both
feed water and resultant water in the refineries must be well treated due to
corrosive effect, quality requirements and environmental issues. Treating the
water via reverse osmosis (RO) membranes is an important industrial process in
terms of sustainability ([1]). This study focuses on the data driven modeling
of an industrial Reverse Osmosis Membrane Unit (ROMU) which includes cartridge
filters, high pressure pump, and RO membrane series. RO membranes are composed
of two stages with 36 and 18 membranes respectively. In more detail, one
membrane module contains 6 spiral wound polyamide membranes as shown in Fig. 1.
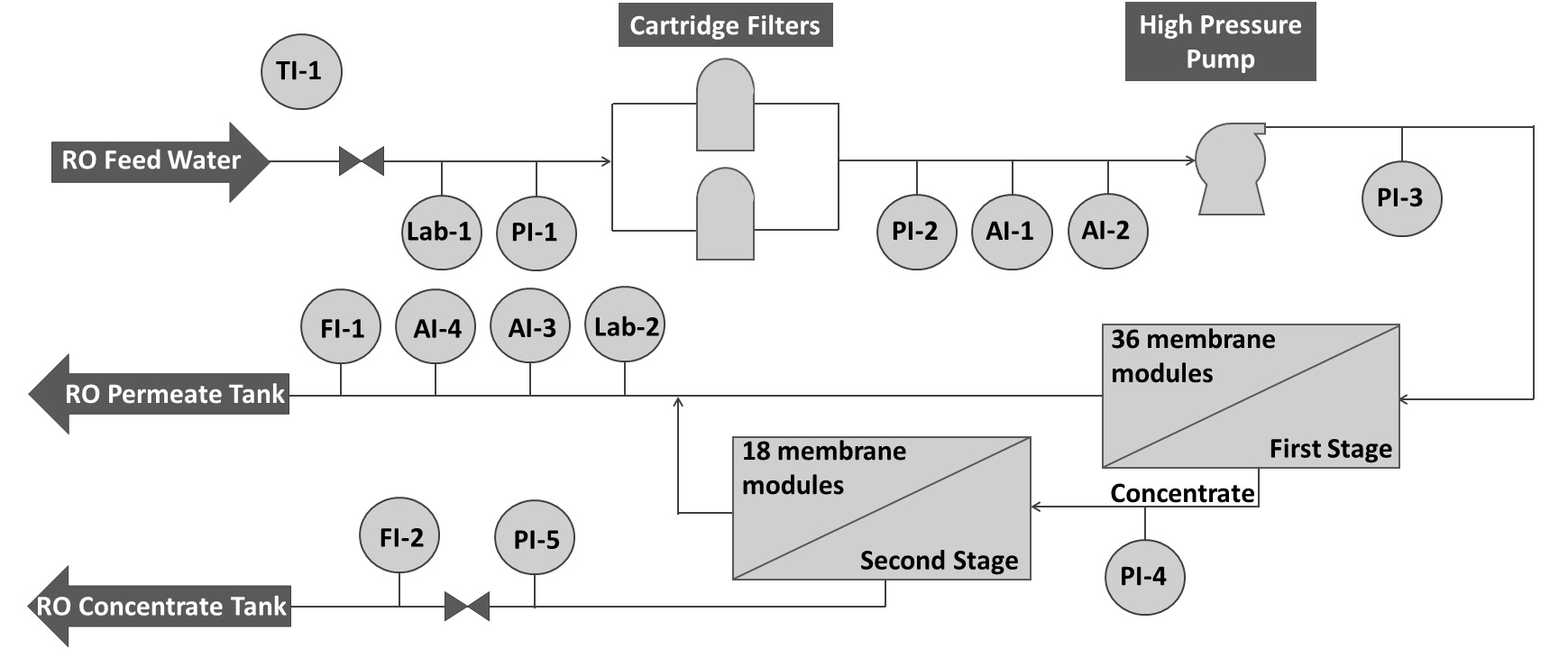
Figure
1.
Simplified ROMU diagram
Despite their wide usage,
RO membranes suffer from permanent fouling once they are not maintained
properly, resulting in higher cost and reduced product amount ([2]). In
addition, the actual nature of the process is complex with high number of
non-linearly coupled process variables. RO membrane fouling modeling and the
determination of the major impacts on the process are the primary objectives of
this study. Artificial Neural Network (ANN) is trained for the identification
of the process to infer the impact of the plant and feed parameters on the
outputs, primarily the product rate. The data for the model development contain
the last 4 years, since the installation of the plant to date. The data include
all sensors related to pressure, flow, temperature, conductivity, and pH. Next, the ANN is evaluated at probable
operating conditions to calculate the operating window of the plant. The
results show the impact of the process parameters on the product rate.
References
[1] Ismail, F., Khulbe, K. C., & Matsuura, T.
(2018). Reverse Osmosis. Elsevier.
[2] Kang, G. D., & Cao, Y. M. (2012). Development
of antifouling reverse osmosis membranes for water treatment: a review. Water
research, 46(3), 584-600.
Presenter(s)
Language
Pricing
Individuals
AIChE Member Credits | 0.5 |
AIChE Pro Members | $19.00 |
AIChE Graduate Student Members | Free |
AIChE Undergraduate Student Members | Free |
Computing and Systems Technology Division Members | Free |
AIChE Explorer Members | $29.00 |
Non-Members | $29.00 |