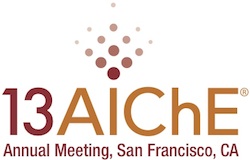
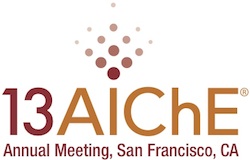
After visiting professor John F. MacGregor in Fall 1997 I got really fascinated by the potential of multivariate latent variable methods for batch process monitoring. Based on the inspiring contributions of John I went on studying new methods to ease and improve batch process monitoring.In this talk I will present some of our novel contributions in this field , based on previous John´s work. In particular I will review two issues: missing data and real-time batch synchronization. In process chemometrics missing data can appear for a number of reasons: failures in sensors or in the communication between the instrumentation and the digital control system (DCS) , sensors taken offline for routine maintenance , manual samples not collected at the required times , data discarded by gross measurement errors , and sensors with different sampling periods. In real-time-batch process monitoring and control , at each time t the future behaviour of the process trajectories is unknown , and then can be treated as missing values. Out of the proposed methods , Trimmed Scores Regression (TSR) [1] turns out to be a simple an efficient method to estimate missing data. Usually batch pace evolution is different batch-to-batch yielding misaligned key process events. Real-time batch synchronization is a critical issue for real-time batch process monitoring. For this purpose the Relaxed-Greedy Dynamic Time Warping (RGTW) algorithm [2] will be introduced. [1] Arteaga F. , Ferrer A. Dealing with missing data in MSPC: several methods , different interpretations , some examples. J. Chemometrics 2002; 16: 408–418. [2] González-Martínez J. , Ferrer A. , Westerhuis J. Real-time synchronization of batch trajectories for on-line multivariate statistical process control using dynamic time warping , Chemometrics and Intelligent Laboratory Systems 2011; 105: 195-206.