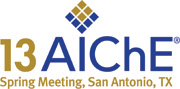
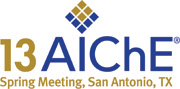
Losses due to unexpected shutdowns and incidents exceed $10 billion every year to the US chemical and petrochemical industry alone. With new advances in information technology, a typical industrial plant operation monitors several hundred parameters continuously, generating on the order of 30M-50M data points every day. Thus, there is a great need of tools that can analyze this “big data” and inform plant personnel of changes in the risk profile of the plant over time with indication of potentially evolving disturbances and help avert unplanned shutdowns and accidents.
This talk will present two new technologies: (a) Dynamic Risk Analysis, and (b) real-time Leading Indicators.
The first part of the talk will discuss Dynamic Risk Analysis technology that assesses risk levels in a plant operation on an on-demand as well as periodic basis. The technique utilizes the entire process (online sensor) and alarm data and extracts crucial risk information by identifying “process near-misses” that serve as leading indicators of potential shutdowns, accidents, incidents, and operational problems. These near-misses serve as early indicators of developing risks and help the plant management to (a) identify deterioration in operations, that is, risk of deviating from normal operation, (b) determine increase in potential of incidents (unplanned shutdowns/accidents) over time, and (c) understand overall health of the process on an on-going basis.
As the risk levels change over time, the technique provides detailed information on formation and/or development of abnormal situations that gets updated on a dynamically. Such a wealth of targeted information helps operating team address issues long before they result in incidents or operational problems. This technology complements the HAZOP based analyses involving scenario-based modeling, quantitative risk analysis, and consequence analysis to determine potential risks.
The second part of the talk will discuss results of real-time Leading Indicators technology that identifies abnormal conditions at their formations stages using process (online sensor) data and sends precise leading alerts when there is a high likelihood of an incident. Post-incident investigations show that there are several near-misses or precursors that occur before these unexpected events that evolve (gradually or often, rapidly) to become abnormal situations. In an alarm-monitored facility, often alarms are too late or too many in number to accurately point out the problems, so that operators can take right corrective actions at the right time. Case studies based on industrial data will be taken to demonstrate how earlier detection of certain abnormal conditions could have avoided the occurrence of undesirable events.