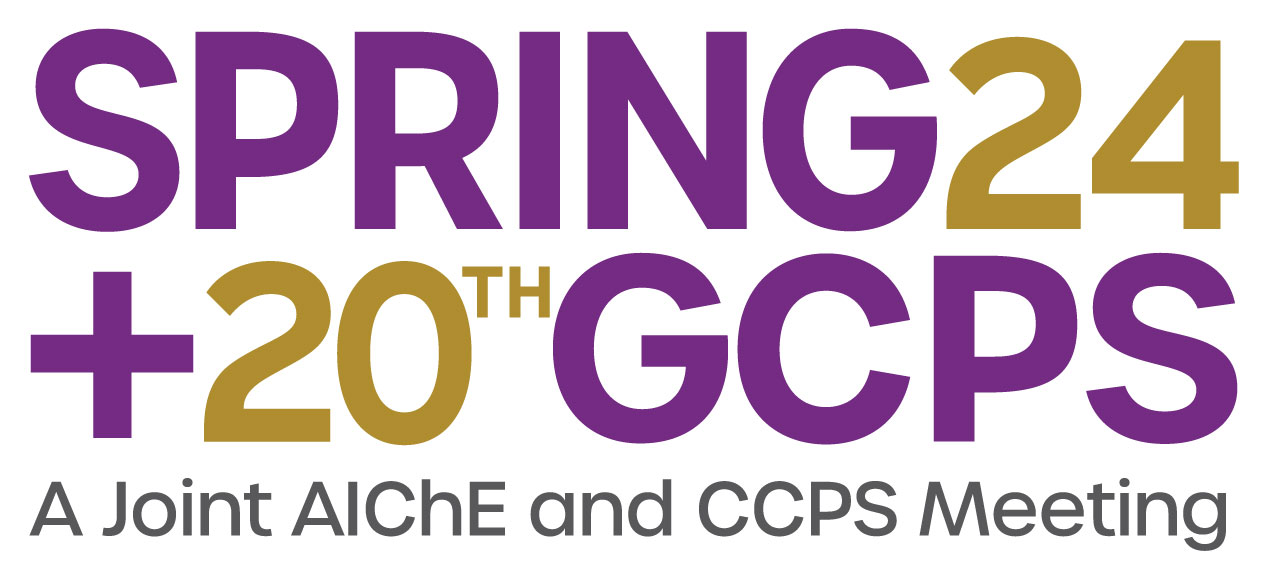
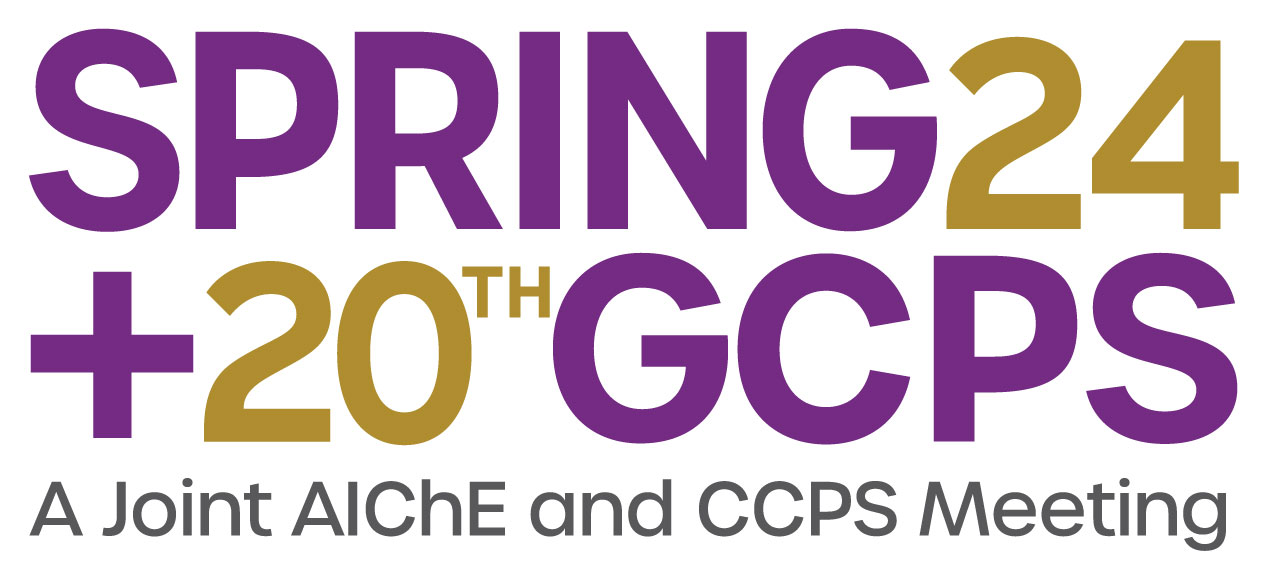
Effectively using AI/ML and APC in Ethylene
Jim Brigman, PE
Application of data analytics and simulation to address problems in petrochemical and refining operations has been an option for over 40 years. Practically all chemical engineers graduating after 1980 were exposed to first principles simulation while getting their degree. Use of steady state simulation to understand the theoretical capabilities of the processing unit where they are working, the operation of distillation columns, or the sizing of heat exchangers has been a common application of simulation for engineers for the last 40 years and continues to be. Likewise the application of statistical analysis such as using statistical process control techniques to optimize unit performance or applying statistical analysis to equipment reliability to increase overall unit availability has been common practice over this period of time and continues to be. However despite the ready availability of these tools, use for day to day use by engineers for the sake of fine tuning operations and recognizing operational opportunities is limited.
Simulation software vendors have continued to improve their products to make them easier to utilize with significantly better visualization and processing speed. With the increase in processing speed and the ease of accessing years of operational data, data analytics tools such as artificial intelligence and machine learning techniques can be applied. The insights from this analysis can be impressive. However still the use of these tools remains limited despite the fanfare of digitalization and the wealth of tools developed.
Advanced Process Control represents the most successful application of data analytics that is widely used. APC applies a broad range of techniques to provide multi-variable control. Within a set of related process variables, relationships are established to automate the task of pushing performance of certain variables to defined optimal points within the defined constraints of the system. This is common practice. However it should be noted that rarely are a companyâs engineers supposed to build the APC system for the plant. Typically an internal group of company engineers specializing on the particular APC system provide the upkeep on these systems and do so often with the support of that specific APC vendor.
Digitalization and the application of AI/ML tools are in vogue and where properly applied are generating enormous value. Getting the right solutions in place is seen as necessary to compete. Yet despite this belief, successful implementation of AI/ML tools is estimated to be occurring less than 30% of the time and even then not performing up to expectations. Based on looking at the use simulation and other statistical tools, this should not be surprising. Attempting to treat digitalization, its implementation, and its use in the same manner as simulation will result in the same limited use and applicability despite its tremendous potential. Treating AI/ML digitalization implementation and use more similarly to APC implementation can significantly increase successful application and use.
This paper will go into the details of how successful solutions were built for Ethylene operations that address the major issues found in Ethylene plant operation. These solutions are not competitive to APC but significantly additive to APC in terms of opportunity identification for profitability, reliability and sustainability. The comparison to APC is solely for showing how the application and implementation of these solutions should mirror the application and implementation of APC systems in order to drive the successful use to capture value.
Examples will be given of AI/ML tools implemented in Ethylene facilities as solutions that are able to effectively address:
- Optimizing furnace runlength, yield, and coil life for increased production
- Improving the reliability and performance of key equipment including the quench tower, cracked gas compressor, and various distillation columns
- Equipment and instrumentation reliability
- Establishing awareness of current operational performance in terms of profitability and sustainability versus theoretical or best ever performance
The ongoing use of these solutions to capture tens of millions in increased production and these solutionâs ongoing maintenance will be described.
While Ingenero does provide an AI/ML tool set, the paper is not concentrating on the tools but the technology implementation and support process necessary to deliver solutions that creates ongoing value in Ethylene facilities that are applicable regardless of selected vendors technology.
Presenter(s)
Language
Pricing
Individuals
AIChE Member Credits | 0.5 |
AIChE Pro Members | $19.00 |
Fuels and Petrochemicals Division Members | Free |
AIChE Graduate Student Members | Free |
AIChE Undergraduate Student Members | Free |
AIChE Explorer Members | $29.00 |
Non-Members | $29.00 |