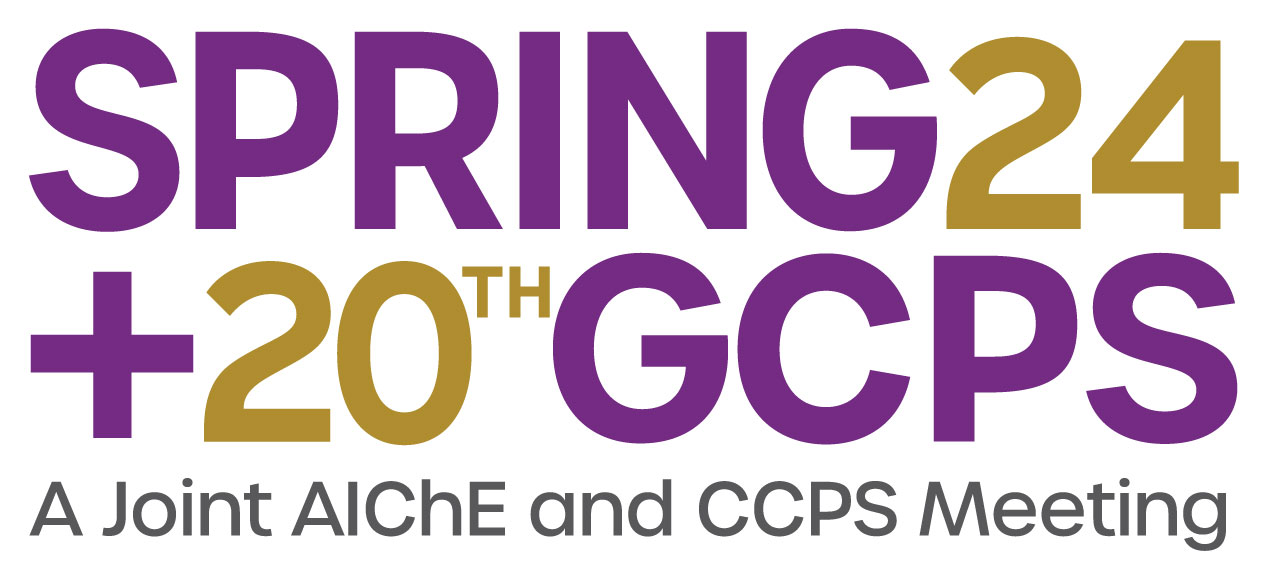
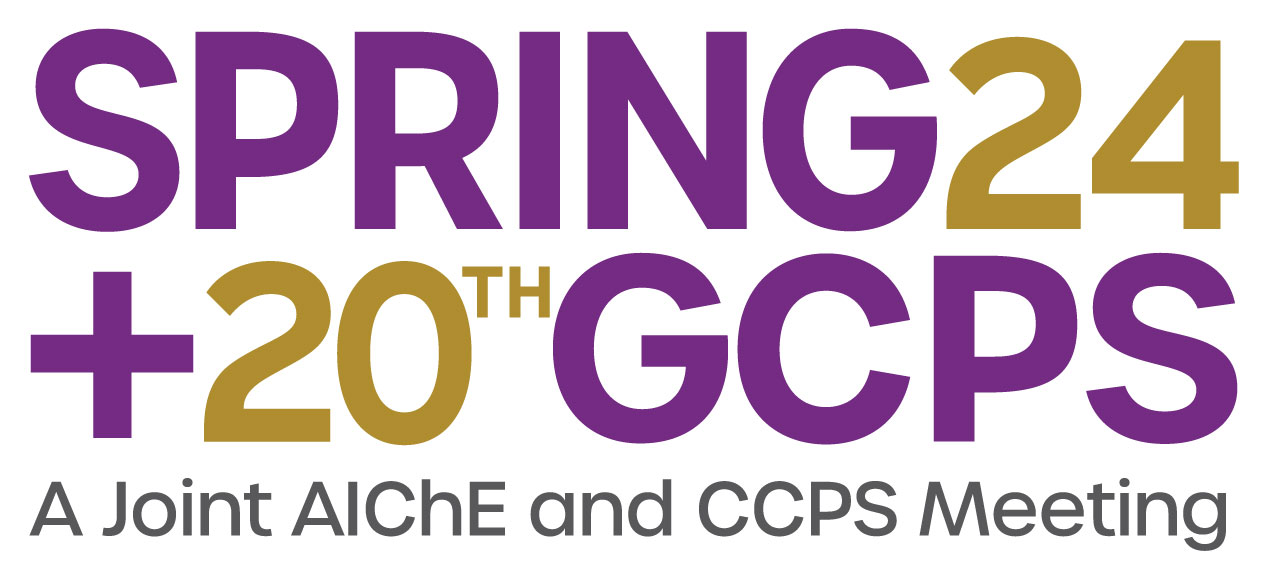
Big data analytics and IIOT can enhance plant performance and reliability by using multi-model techniques and systematic analysis of plant operation. Multi-modeling is essential, but it also needs to be integrated with data collection, model-based information and knowledge generation, visualization and dashboards for interpretation and early detection, and implementation and tracking for benefit realization.
Successful application of a good overall asset effectiveness (OAE) solution considerably helps in improving the asset effectiveness resulting in,
- Longer mean time between machine overhauls/ replacement à extending T/A intervals
- Improving efficiency and overall asset effectiveness à increasing plant production capacity depending on the severity of operating constraints
- Operating plant to optimal best levels while ensuring reliability and safety
Using a combination of first principle, empirical models, and predictive analytics, smart solutions can detect equipment constraints and deterioration in real time. They also provide useful information for making timely and appropriate decisions to optimize asset effectiveness and ensure operational safety. Industry specific advanced analytics of historic and real-time data enable improvements that are challenging to achieve with traditional monitoring approaches.
First principle models are based on established scientific principles, while empirical models capture complex relationships that may not be easily described mathematically. By combining them, we can improve the accuracy and robustness of our predictions and simulations. This approach is called hybrid modeling or data-driven modeling, and it is widely used in engineering, economics, and environmental science. However, it is important to understand the problem, the available data, and the physical processes when first principle and empirical models are applied together.
Hybrid modeling enables continuous evaluation of measured and derived parameters against established engineering limits. This can reveal opportunities and potential reliability issues. The performance is tracked using suitable techniques, tools and equipment models, and the deviation from normal is quantified. Moreover, predictive and prescriptive analytics can help assess the impact of deviation, the need for corrective action and the assistance in finding challenging solutions.
Data analytics can enhance the plant operating strategy and lead to substantial economic gains in the ethylene process industry. This paper will discuss how an effective predictive analytics solution driven by different techniques, tools and models is effectively utilized to optimize production and asset performance of an ethylene plant.
Presenter(s)
Language
Pricing
Individuals
AIChE Member Credits | 0.5 |
AIChE Pro Members | $19.00 |
Fuels and Petrochemicals Division Members | Free |
AIChE Graduate Student Members | Free |
AIChE Undergraduate Student Members | Free |
AIChE Explorer Members | $29.00 |
Non-Members | $29.00 |