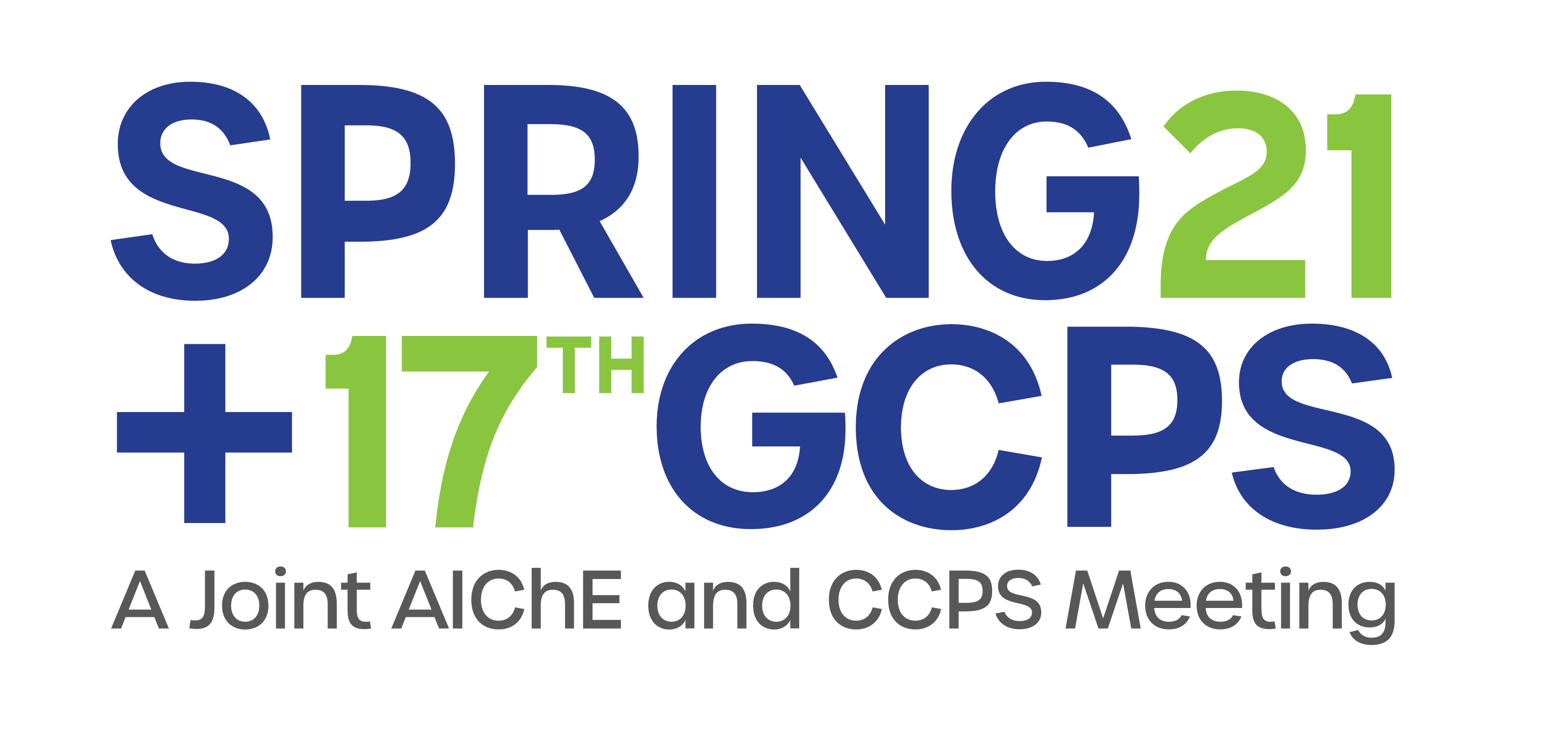
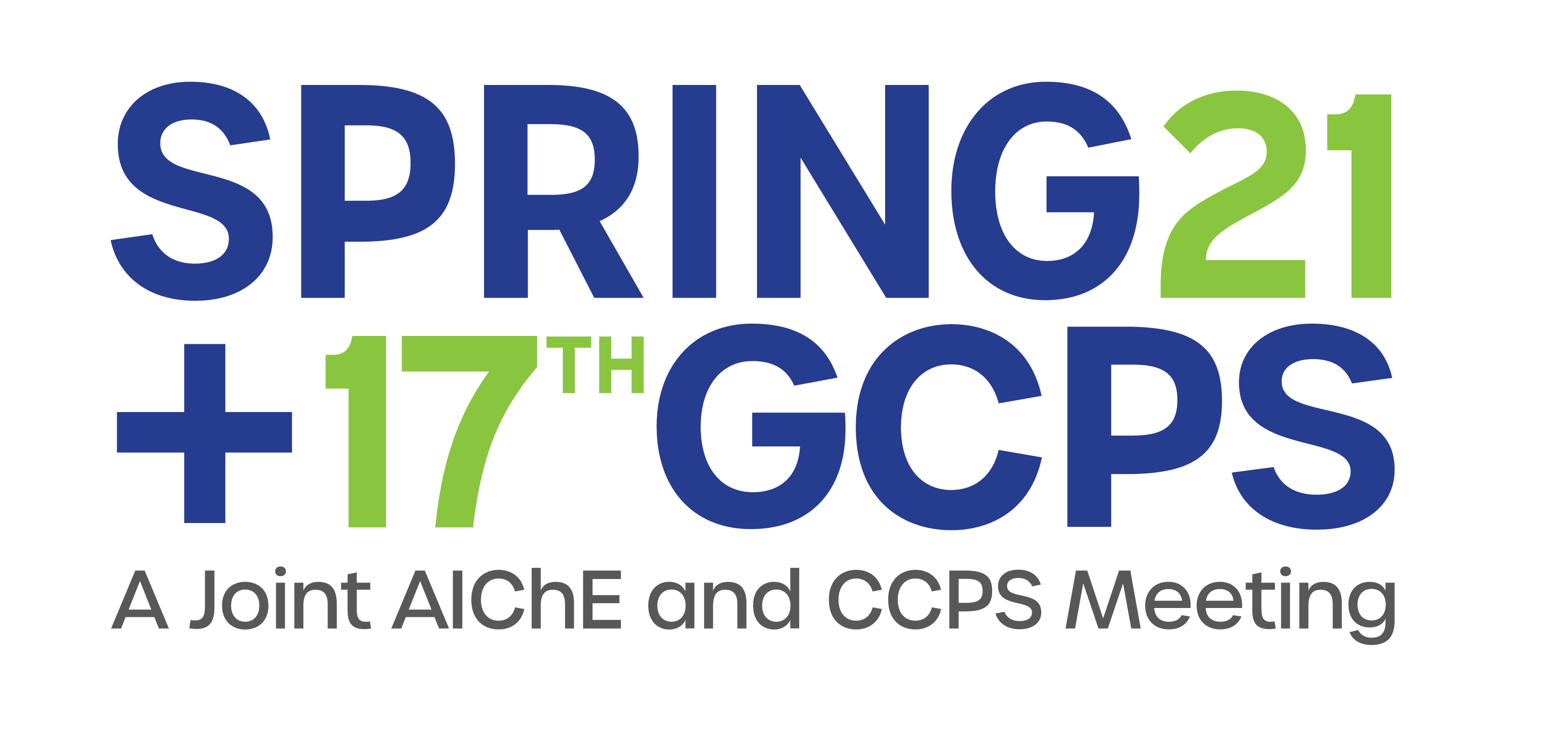
Often the tasks of Big Data Analysis and/or Discovery are impeded by the size of data sets. Moreover, when data sets are large and the desired information content in the data is quite small, the ability to discover and/or analysis this content is extremely challenged. This is akin to a real mining effort when most of the solids and elements are not the precious âgemsâ being sought. A powerful multivariate statistical approach that is often applied in such situations is Principal Component Analysis (PCA). However, the common application of PCA is to keep the top most latent variables that explain a certain threshold of the variation and discard all the rest. This approach can miss rare âgemsâ that show up in the extreme low percentages of the variation. The talk presents a PCA methodology that can find critical behavior (i.e., effects) in very large data sets when the number of experiments are very small in comparison to the number features for each experiment. An illustration of this approach will be given for a micro-array Covid-19 study of three groups types: smokers, never smokers and smokers that quit. This deep analysis will identify subgroups in these three groups with as many as one subject out of more than seventy subjects in the study.
Presenter(s)
Once the content has been viewed and you have attested to it, you will be able to download and print a certificate for PDH credits.
If you have already viewed this content,
please click here
to login.