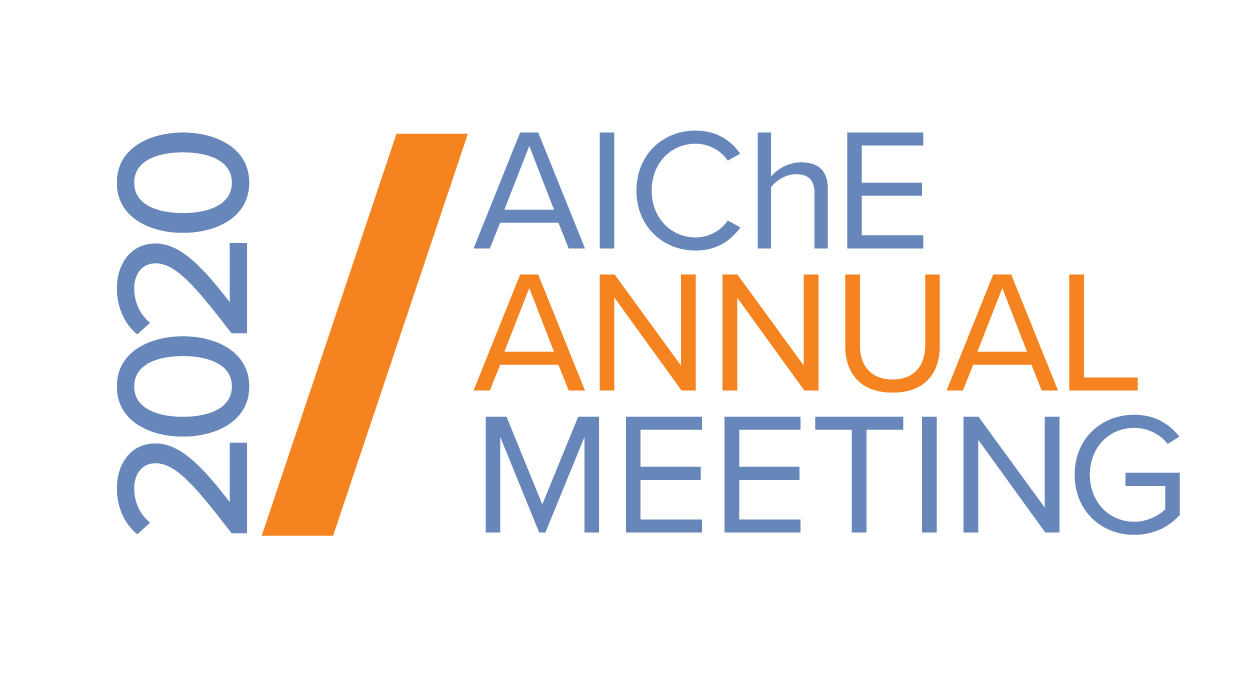
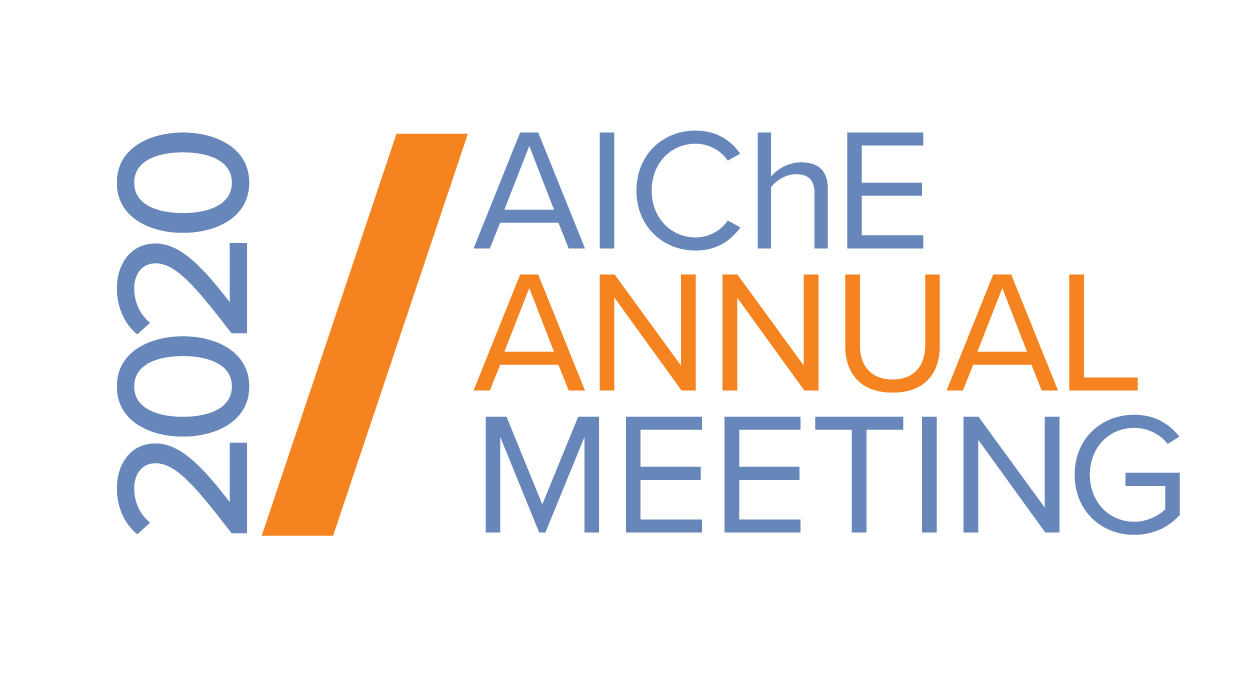
The complex energy landscape is thoroughly analyzed [5-9] to accurately determine the two key factors of this framework: the total demand of the energy products directed to the end-use sectors, and the corresponding price of each product in the form of either a monthly or a spot price [10-14]. We have already presented a rolling horizon forecasting methodology for the demands of energy in the future [4], and here we demonstrate a novel technique to forecast the future prices of energy feedstocks and commodities up to 12 months. The historical prices of each energy product and commodity are studied individually, so as to identify patterns, trends, cycles, outliers, relationships among different variables etc. Then, different forecasting models are fitted in training data sets i.e. Exponential Smoothing, ARIMA, Dynamic Regression, Neural Networks etc. The best forecasting model for each energy product and commodity is selected based on the validation results from testing data sets, and is then utilized to produce the 12 months forecasts. Being able to forecast the future prices of energy has tremendous potential for applications in economics, finance, engineering, law and policy.
References:
- Regnier, E., 2007. Oil and energy price volatility. Energy economics, 29(3), pp.405-427
- I.W. Parry, M. D. Heine, E. Lis, and S. Li, Getting energy prices right: From principle to practice. International Monetary Fund, 2014.
- Barnett, J.; Barron, J.; Oil market volatility is at all-time high. Energy Information Administration (EIA), Today in Energy, March 2020.
- Baratsas, S.; Niziolek, A.; Onel, O.; Matthews, L.; Floudas, C. A.; Hallermann, D.; Sorescu, S.; Pistikopoulos, E. N. What is the price of energy to the end-users? A novel predictive framework and one of its applications to the energy and monetary policies. Nature Communications 2020 (Under Review).
- Elia, J. A.; Baliban, R. C.; Xiao, X.; Floudas, C. A. Optimal energy supply network determination and life cycle analysis for hybrid coal, biomass and natural gas to liquid (CBGTL) plants using carbon-based hydrogen production. Computers and Chemical Engineering 2011, 35, 1399-1430.
- Elia, J. A.; Baliban, R. C.; Floudas, C. A. Nationwide energy supply chain analysis for hybrid feedstock processes with significant CO2 emissions reduction. AIChE Journal 2012, 58 (7), 2142-2154.
- Elia, J. A.; Floudas, C. A. Energy Supply Chain Optimization of Hybrid Feedstock Processes: A Review. Annual Review of Chemical and Biomolecular Engineering 2014, 5 (1), 147-179.
- Onel, O.; Niziolek, A. M.; Floudas, C. A. Integrated biomass and fossil fuel systems towards the production of fuels and chemicals: state of the art approaches and future challenges. Current Opinion in Chemical Engineering 2015, 9, 66-74.
- Floudas, C.A.; Niziolek, A. M.; Onel, O.; Matthews, L.R. Multi-Scale Systems Engineering for Energy and the Environment: Challenges and Opportunities. AIChE Journal 2016, 62 (3), 602-623.
- Energy Information Administration (EIA), Annual Energy Outlook, 2019
- Energy Information Administration (EIA), Monthly Energy Review, 2019
- Lazard, Levelized Cost of Energy Analysis Version 13.0, 2019
- Bureau of Labor Statistics (BLS), Producers Price Index, 2019
- S. Department of Energy (DOE), Alternative Fuels Data Center, 2019