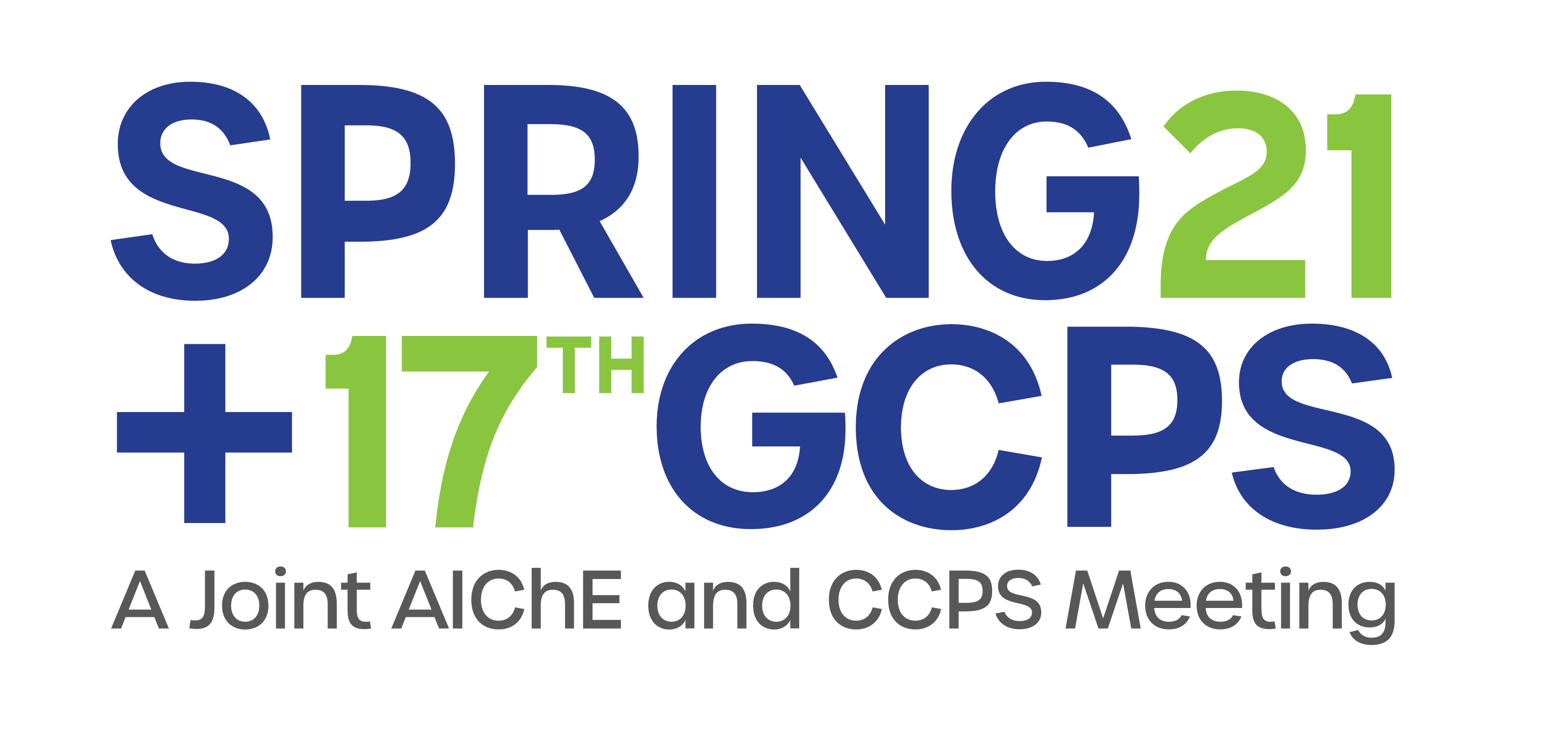
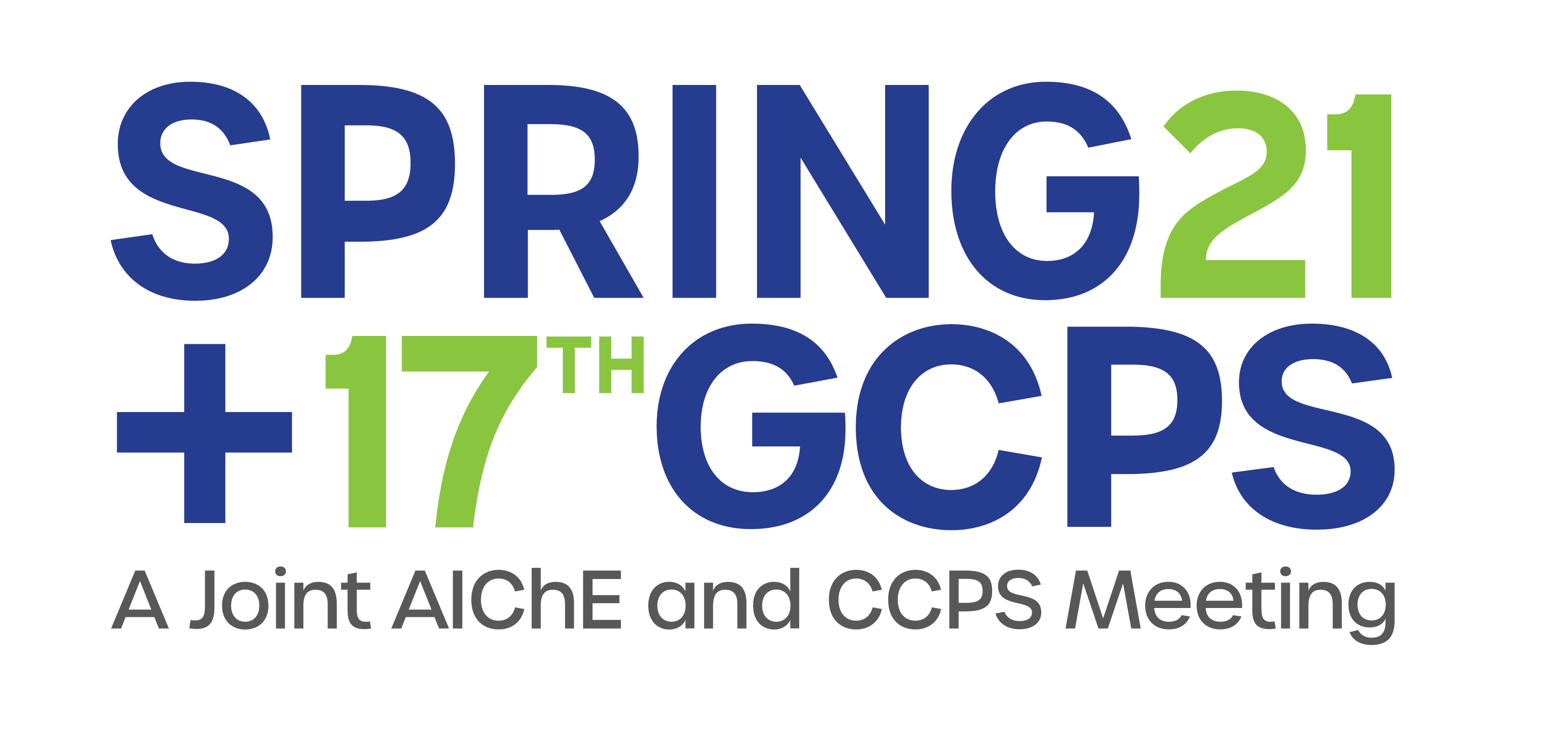
Incidents in process industries still occur globally even though professionals put many efforts into improving process safety over the decades. Although randomness may be an element, incidents are often not chance-events in that these suddenly occur out of nowhere. They do follow incubation periods where weak signals exist a long time before the incidents occur and these signals need to be recognized and resolved as early as possible to prevent the incidents. Weak signals emerge as complex interactions among performance variabilities of human, organization and technological functions, therefore their identification could be intellectually unmanageable. In the study, a framework to identify weak signals was developed and consisted of a system-based hazard identification method (i.e., Functional Resonance Analysis Method) and machine learning models. Its application in a batch polymerization process was implemented for illustration. The FRAM was first applied to understand the interactions in the system and determine what information would be needed for weak signal identification. The information was generated by a digital twin, which was developed based on the FRAM to simulate possible function interactions in the batch process. Finally, Random Forest was developed to predict the probability of unexpected temperature deviation given a set of potential weak signals, and Decision Tree was developed to identify the corresponding combination of weak signals, which can proactively predict the unexpected scenario.
Presenter(s)
Once the content has been viewed and you have attested to it, you will be able to download and print a certificate for PDH credits.
If you have already viewed this content,
please click here
to login.