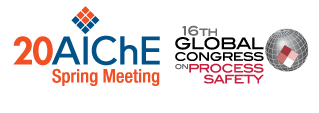
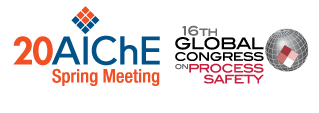
On the other hand, if we review the data in any chemical processes, a commonality can be observed that most of the measurements are pressures, temperatures, flow rates, levels and concentration of different species. Typically, their intrinsic correlations can be summarized by the first principles, such as mass balances, gas laws, energy balances and etc. Although the first principle approach requires a lot of effort to investigate a physical system, while it provides a more general solution for different kinds of systems. Additionally, commonalities also widely exist in process monitoring and fault detection models. Regardless of the number of input variables used in the model, the objective is always to correctly identify the distribution, ~N(μ,Ï2) of normal operating conditions and distinguish the abnormality of test data.
With the recent development of deep learning techniques, it offers the feasibility to build models with better generalization capability. Inspired by the recent breakthroughs in computer vision field, a transfer learning approach was proposed using convolutional neural networks (CNN), in order to extract general features from process data. The proposed framework is more generalized than conventional methods, where features across different processes with different variables can be learned. The proposed CNN classifier was first trained on the famous benchmark, the Tennessee Eastman Process (TEP) [3] to learn the patterns of different kinds of faults. The features learned in the intermediate layers of the CNN classifier can be pooled out for various tasks. In this work, a fault detection method is demonstrated based on the proposed feature extraction tool, which provides a superior generalization ability that can be applied outside the TEP datasets.
References:
[1] L. H. Chiang, E. L. Russell and R. D. Braatz, Fault detection and diagnosis in industrial systems, New York City: Springer Science & Business Media, 2000.
[2] S. W. Choi and e. al., "Fault detection and identification of nonlinear processes based on kernel PCA," Chemometrics and intelligent laboratory systems, vol. 75.1, pp. 55-67, 2005.
[3] J. J. Downs and E. F. Vogel., "A plant-wide industrial process control problem," Computers & chemical engineering, vol. 17.3, pp. 245-255, 1993.
Presenter(s)
Language
Pricing
Individuals
AIChE Member Credits | 0.5 |
AIChE Pro Members | $19.00 |
AIChE Graduate Student Members | Free |
AIChE Undergraduate Student Members | Free |
Computing and Systems Technology Division Members | Free |
AIChE Explorer Members | $29.00 |
Non-Members | $29.00 |