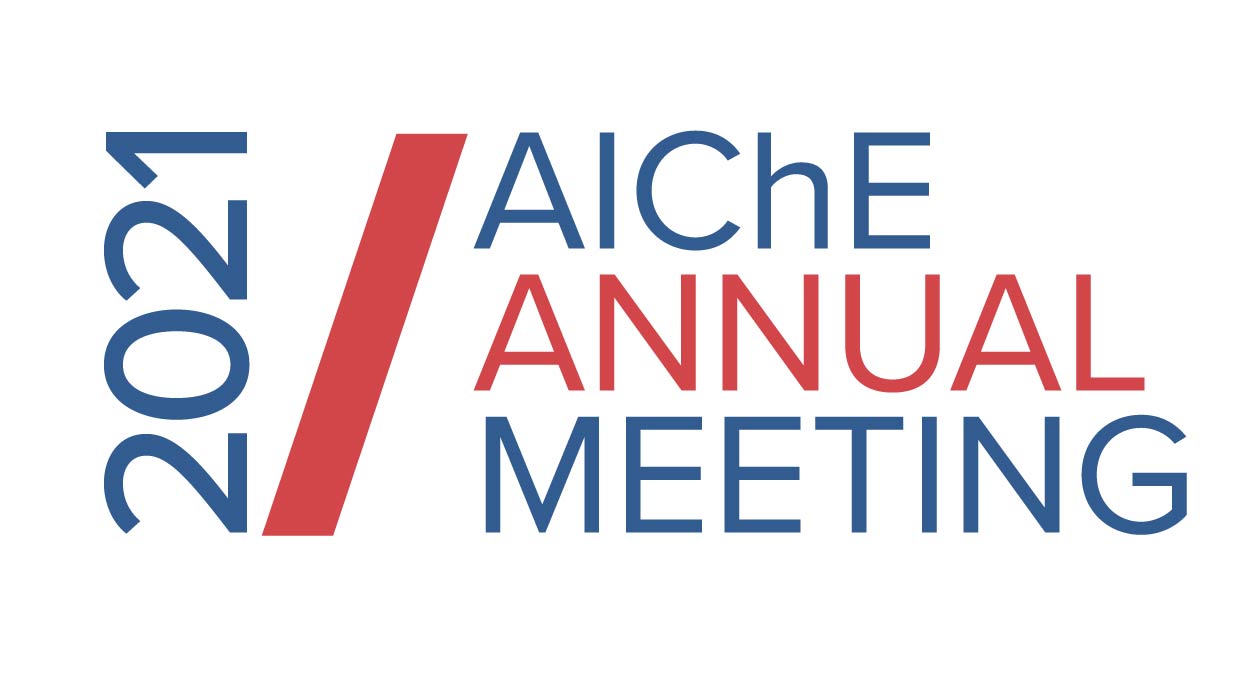
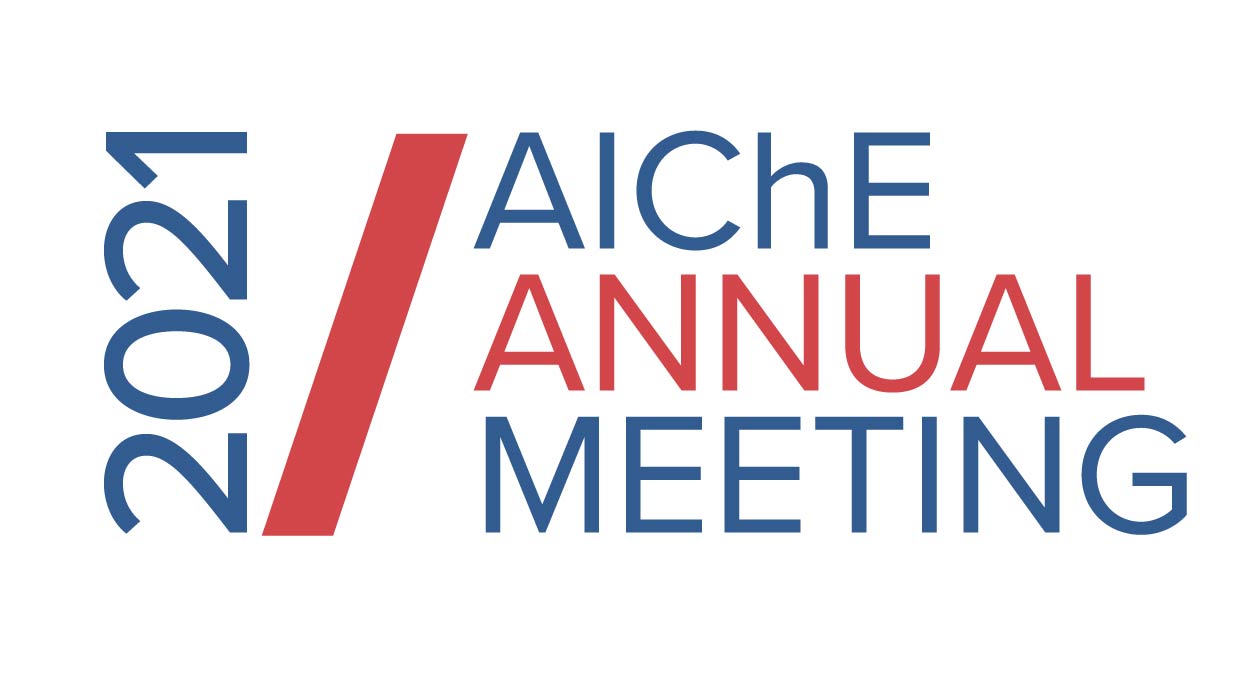
In general, there are two approaches for membrane system design: sequential and simultaneous. In the sequential approaches, the permeator configuration is fixed prior to the determination of operating conditions. However, these approaches may yield suboptimal final designs due to the restricted search space. On the other hand, in simultaneous approaches, both structural and operating decisions are determined simultaneously. This is usually done by postulating a superstructure which contains many candidates for the optimal process configuration [4]. To represent more process configurations, some studies use a larger superstructure which could represent each separation stage as several permeators in parallel or in series. The network representations in these studies, however, consider only one inlet and two outlets. However, more efficient membrane-based separations can be designed if a more generalized representation is adopted where inputs and outputs are not too restricted. For example, one could envision combined reactor-separation systems where the feed into the separation (membrane) system is unknown or can be represented by two candidate feeds. Furthermore, describing the permeation of multicomponent mixtures mathematically presents a major challenge. Due to the governing transport equations, the resulting unit model comprises differential algebraic equations which are mathematically complex and computationally demanding when used in an optimization framework. Most studies in this area focus on obtaining local optima[4,5], whereas those which achieve global optimality [7] are too restrictive (e.g., consider only binary feed mixtures or fixed configurations).
Accordingly, the aim of this work is to develop a global optimization framework to design membrane networks for multicomponent gas separations. We present a mixed integer nonlinear programming (MINLP) model to find a configuration and operating conditions which result in minimum cost for a single feed stream and two product streams (i.e., retentate and permeate final product streams). We employ a rich superstructure with structural decisions for interconnections between stages (i.e., bypasses and recycles), the feed stage, and stage-to-retentate/permeate product streams. Since the concentrations of retentate and permeate of a stage can vary greatly depending on the stage cut, we allow the retentate to go to the next stages as bypass stream and the permeate to be sent back to the previous stages as recycle stream to add all feasible connections. All structural decisions are represented by binary variables. To retain computational tractability, we use an approximate unit model to describe the membrane permeators.
The proposed optimization model also allows pressure ratio at each stage to be a design variable. Furthermore, we introduce feed variability in our model to represent cases where there are different options of feed streams because, in integrated systems, we may have trade-offs between the difficulty/cost of the reactor network vs. the separation network. Finally, we showcase the applicability of the proposed model through several illustrative examples.
References
[1] R. W. Baker and B. T. Low, âGas separation membrane materials: A perspective,â Macromolecules, vol. 47, no. 20, pp. 6999â7013, 2014.
[2] L. M. Robeson, âThe upper bound revisited,â J. Memb. Sci., vol. 320, no. 1â2, pp. 390â400, 2008.
[3] R. Qi and M. A. Henson, âOptimal design of spiral-wound membrane networks for gas separations,â J. Memb. Sci., vol. 148, no. 1, pp. 71â89, 1998.
[4] I. E. Grossmann, âMixed-Integer Optimization Techniques for Algorithmic Process Synthesis,â Adv. Chem. Eng., 1996.
[5] R. Qi and M. A. Henson, âMembrane system design for multicomponent gas mixtures via mixed-integer nonlinear programming,â Comput. Chem. Eng., vol. 24, no. 12, pp. 2719â2737, 2000.
[6] A. M. Arias, M. C. Mussati, P. L. Mores, N. J. Scenna, J. A. Caballero, and S. F. Mussati, âOptimization of multi-stage membrane systems for CO2 capture from flue gas,â Int. J. Greenh. Gas Control, vol. 53, pp. 371â390, 2016.
[7] B. Ohs, J. Lohaus, and M. Wessling, âOptimization of membrane based nitrogen removal from natural gas,â J. Memb. Sci., vol. 498, pp. 291â301, 2016.