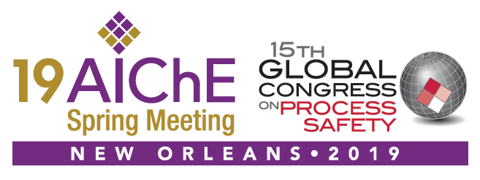
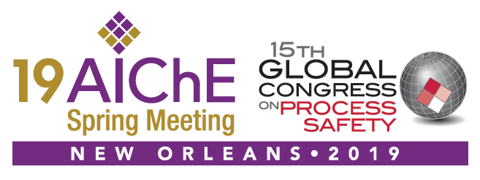
The symptomatic indicators reside in the large volumes of a variety of data that is measured on a continuous basis from the manufacturing operation and archived. However, identifying such symptoms is difficult and time consuming for the unaided human mind, unless it shows up obviously on a commonly monitored parameter. Such identification would entail sifting through thousands of a wide variety of data points, collected at minute wise or higher frequencies. Several of these parameters measured are non-linearly related to one another, further complicated by different types of errors in the collected data.
Big Data Analytics techniques can be utilized to help effectively analyze thousands of minute wise tag data for an Ethylene plant, in real time, to help âfind problems before they find youâ. This paper describes how Machine Learning Techniques have been applied to Ethylene Manufacturing to provide useful, non-intuitive insights that are usually hidden in the large volumes of data collected. When carefully integrated with fundamental process models and combined with domain knowledge, it helps identify symptoms well in advance and allows for Root Cause Analysis to be done before it precipitates as a Reliability issue. The integrated approach is essential to minimize alerts on âfalse positivesâ, which is important for the sustained utilization of such an application.
The multivariate nature of these techniques makes this approach different and more effective than the traditional Statistical Process Control (SPC). Sixteen Unit operations have been identified as common areas prone to unforeseen reliability issues in the Ethylene Plant. These techniques have been effectively hooked to live plant data for effective Reliability monitoring and automatic outlier identification, with focus on the sixteen identified areas. Also, coupling this with vendor equipment performance curves and fundamental predictive models, allows for live comparison of the equipment performance with its design. Commonly occurring failures can be predicted early based on pattern recognition using failure maps and signatures. Not so commonly occurring failures are also identified with indicators that allow basic engineering analysis to predict possible reliability issues.
Case examples in Ethylene plants where these techniques have enabled early identification of possible anomalies; causes of possible failures and increased equipment effectiveness are described in this paper.
Presenter(s)
Language
Pricing
Individuals
AIChE Member Credits | 0.5 |
AIChE Pro Members | $19.00 |
Employees of CCPS Member Companies | Free |
AIChE Graduate Student Members | Free |
AIChE Undergraduate Student Members | Free |
AIChE Explorer Members | $29.00 |
Non-Members | $29.00 |