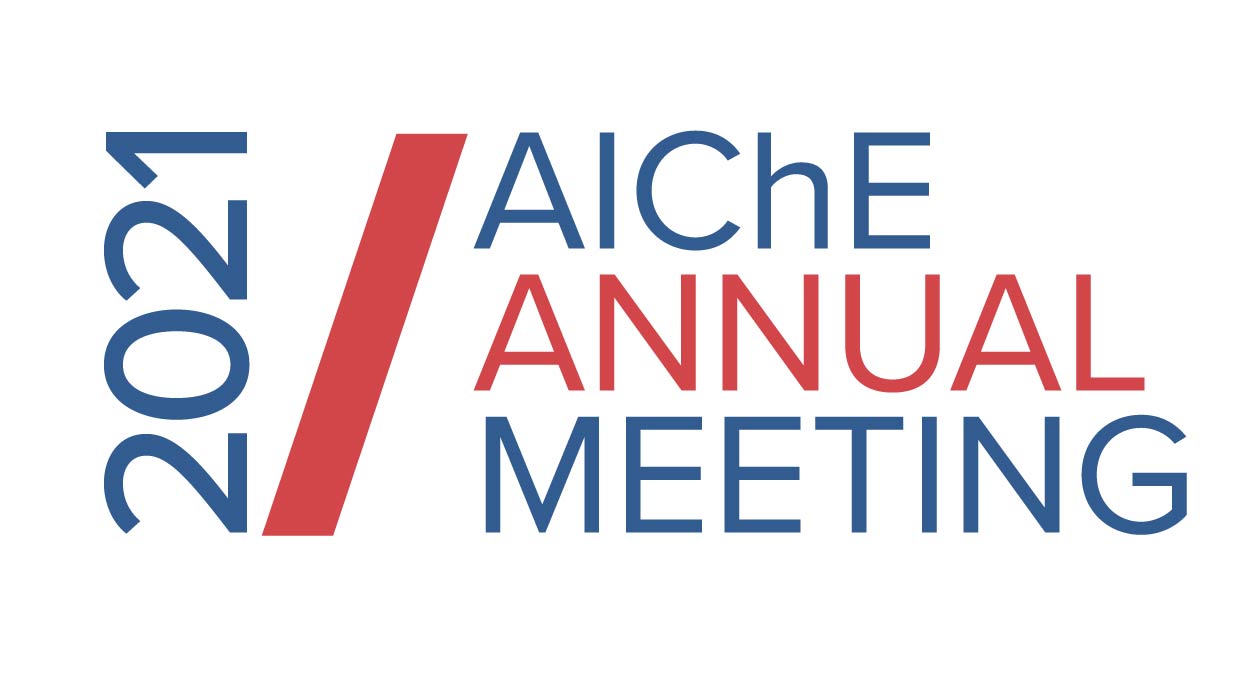
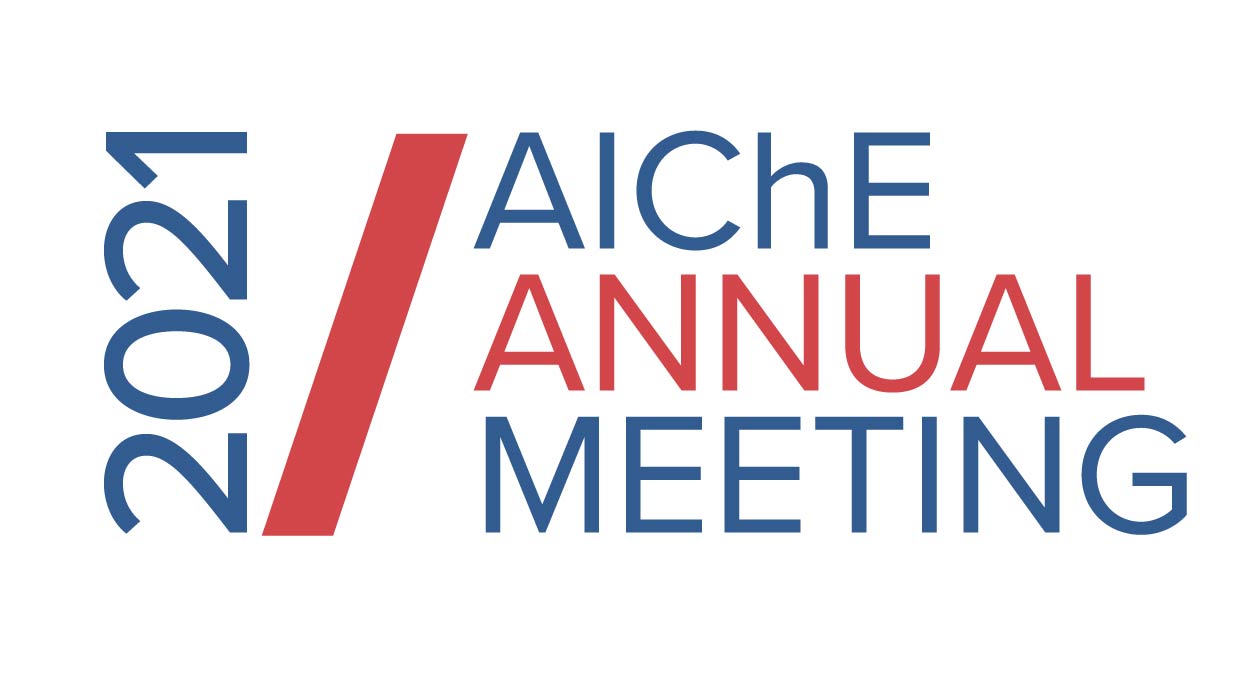
In this work, we conjecture that decision-making processes can be naturally modeled as mathematical optimization problems, and apply the concept of inverse optimization [3] to learn such decision-making models from data. We assume that observed decisions are optimal solutions (possibly with some noise) to a parameterized optimization model. Then, the data-driven inverse optimization problem is to determine the model parameters that minimize the difference between the observations and the model predictions obtained from solving the resulting learned optimization model. We formulate the problem as a bilevel program and apply a KKT-based reformulation to obtain a single-level optimization problem. The resulting nonconvex nonlinear problem is solved with an approximate block coordinate descent-based solution method.
The main advantages of the proposed data-driven inverse optimization method are threefold: (i) flexibility, i.e. the capacity to directly incorporate first-principles knowledge and constraints, (ii) interpretability, since clear cause-effect relationships are directly encoded in the model, and (iii) accuracy, as reflected in a high prediction quality relative to the amount of data required. We demonstrate the effectiveness of the proposed approach in computational experiments and show that it frequently, especially in problems involving more complex constraints, outperforms alternative machine learning methods.
References
1. Chu, W. & Ghahramani, Z. Preference learning with Gaussian processes. ICML 2005 - Proc. 22nd Int. Conf. Mach. Learn. 137â144 (2005).
2. Abbeel, P. & Ng, A. Y. Apprenticeship learning via inverse reinforcement learning. in Proceedings, Twenty-First International Conference on Machine Learning, ICML 2004 (2004).
3. Ahuja, R. K. & Orlin, J. B. Inverse optimization. Oper. Res. 49, 771â783 (2001).