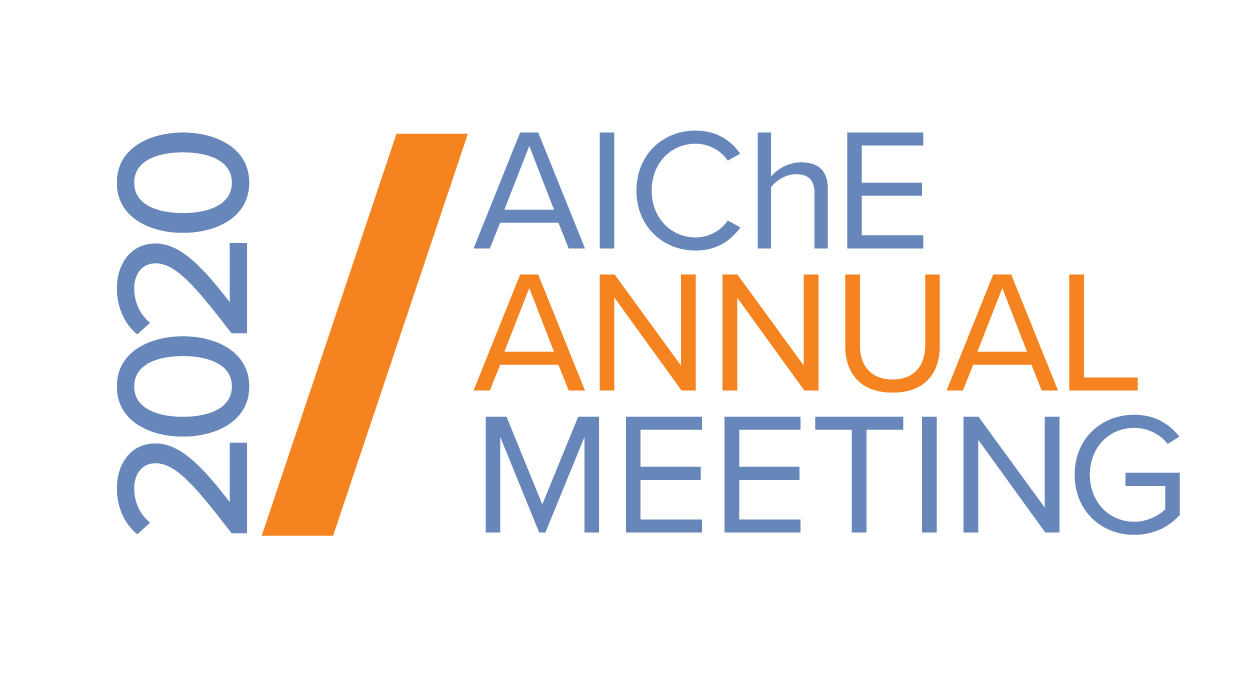
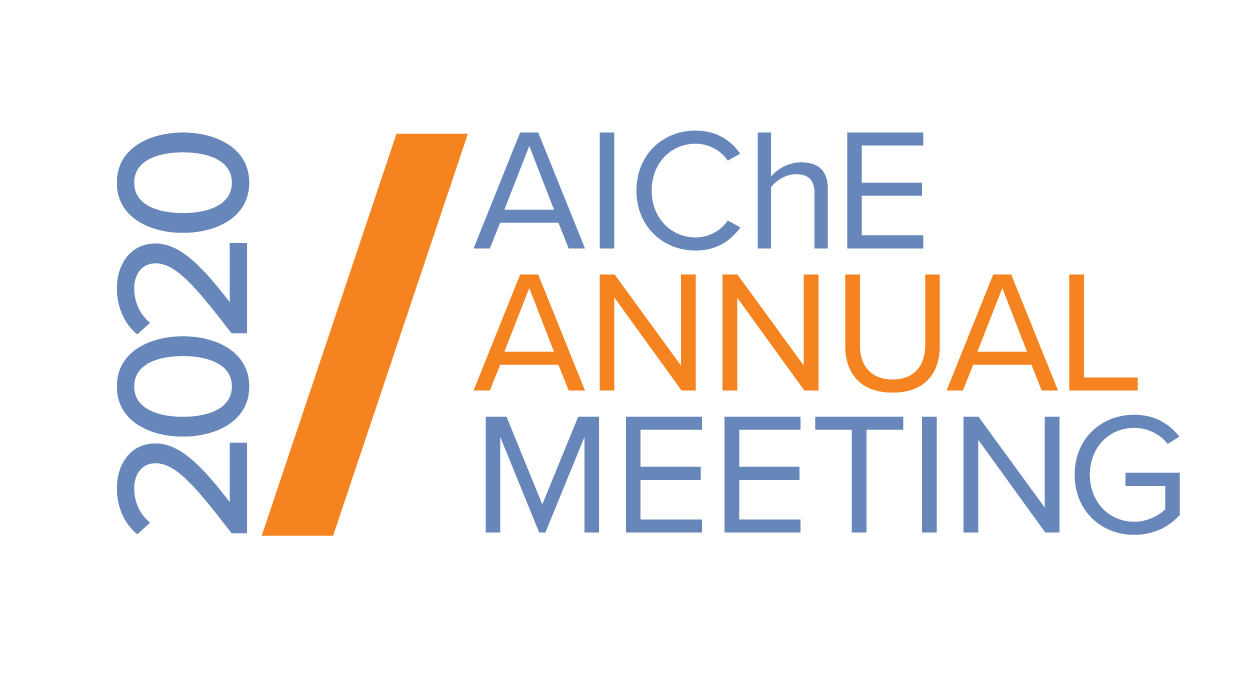
Using conventional reaction rate equations and parameters, the reaction kinetics can be modeled with enough accuracy [2]. However, the mechanisms that determine the growth of the worm-like structures are currently not well understood. Given this currently-inadequate chemical and physical understanding, an alternative to first-principles modeling to relate product (polymer) properties such as contact angle to iCVD processing conditions is to use an empirical modeling approach such as neural networks. A typical neural network model can contain a number of fitting parameters, many times greater than the number of observations available to fit the model with. This relatively large number of fitting parameters provides a lot of flexibility for shaping the response surface of the target (output) variable(s).
In this work, we model the contact angle of heptane on PPFDA as a function of iCVD operating conditions using a neural network architecture. We then use the developed model to determine the optimal iCVD operating conditions that maximizes the heptane contact angle on the worm-like surface. To verify model predictions, we independently perform iCVD laboratory experiments with the model-predicted optimal operating conditions, perform microscopy to elucidate the polymer structure, and measure the resulting heptane contact angles.
References
- Lau, K.K.S.; Chen, Z.; Nguyen, T. Directed Vapor Deposition and Assembly of Polymer Micro- and Nanostructures. S. Patent Application 62941086; November 27, 2019.
- Lau, K.K.S. Growth Mechanism, Kinetics, and Molecular Weight. In CVD Polymers: Fabrication of Organic Surfaces and Devices (ed. Gleason, K.K.); Wiley 2015.