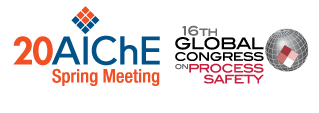
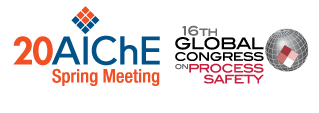
This study used lower flammability limit (LFL) data and ten calculated molecular descriptors data of 78 pure chemical compounds to construct Quantitative structure-property relationship (QSPR) models. Four machine learning methods, k-nearest neighbors (k-NN), support vector machine (SVM), random forest (RF) and boosting, are applied to QSPR models to improve prediction accuracy. Prediction errors and accuracy are compared with traditional multiple linear regression (MLR) models. A novel cross validation method, 10-fold cross validation method, is also used to increase the data usage and prediction reliability. Result shows that models generated by machine learning methods have a significantly lower root mean square error (RMSE) than traditional MLR method in the test dataset. Machine learning based models can be used as substitution methods to improve UFLs predictability of chemical compounds
Presenter(s)
Once the content has been viewed and you have attested to it, you will be able to download and print a certificate for PDH credits.
If you have already viewed this content,
please click here
to login.
Language
Pricing
Individuals
AIChE Member Credits | 0.5 |
AIChE Pro Members | $19.00 |
Employees of CCPS Member Companies | Free |
AIChE Graduate Student Members | Free |
AIChE Undergraduate Student Members | Free |
AIChE Explorer Members | $29.00 |
Non-Members | $29.00 |