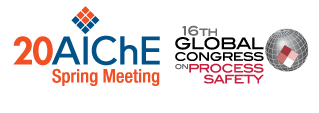
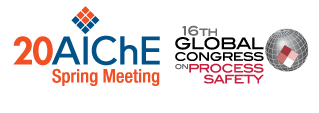
A number of algorithms have been developed in order to improve existing fault detection and diagnosis performance. These algorithms integrate a number of different data-driven driven tools and methods. Multiscale wavelet-based representation of data can be used in order to handle data that is autocorrelated, non-Gaussian, and noisy. Hypothesis testing methods such as the Generalized Likelihood Ratio (GLR) technique can be used in order to provide the best possible detection for a fixed false alarm rate. Moreover, certain model-based methods have been developed in order to monitor process drifts and degradations in the process model, even when a process is operating under control.
This work will discuss the different tools utilized in development of the algorithms, their features, and practical applications, including the benchmark Tennessee Eastman Process (TEP).