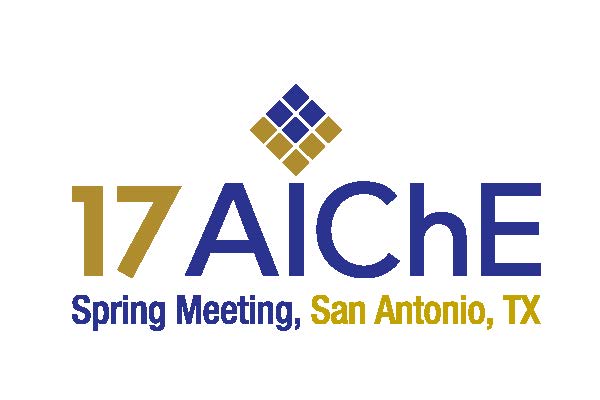
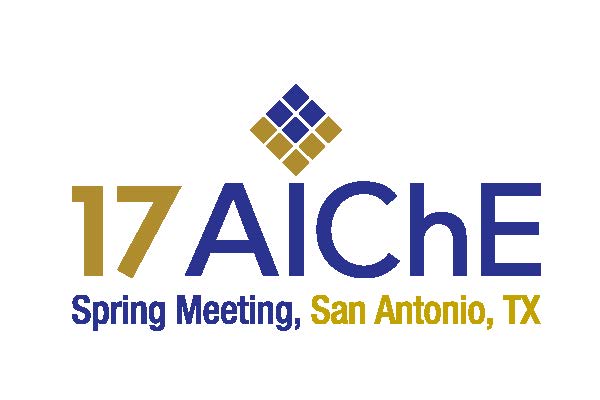
Multiscale representation of improved Multi-Kernel Partial least square technique for process monitoring of the industrial processes
Chiranjivi Botrea, Majdi Mansourib, Mohamed N. Nounouc, Hazem N. Nounoub and M. Nazmul Karima
bElectrical and Computer Engineering Program, Texas A&M University at Qatar, Doha, QATAR,
cChemical Engineering Department, Texas A&M University at Qatar, Doha, QATAR
Abstract
Process monitoring is an important aspect in the chemical industries to ensure safe and proper operation and to maintain process efficiency at the desired level. Data based process monitoring technique have been successfully applied where accurate process model is not available. Process monitoring can be carried out in two phases; fault detection and fault diagnosis. In this work we have proposed Multiscale Multi-Kernel Partial Least Square (MS-MKPLS) based moving window generalized likelihood ratio test (MW-GLRT) for the fault detection and fault diagnosis is be carried out with the contribution plot analysis.
Partial Least Square (PLS) is a popular input output type fault detection model but this technique can be effectively applied mostly to linear processes. Kernel extension of PLS provides an effective technique for fault detection of nonlinear industrial processes. Being an input output model, KPLS can also used as nonlinear regression technique. Selection of kernel function and its parameter have a significant impact on the fault detection performance of the KPLS algorithm, therefore in this work we have proposed optimized multi-kernel PLS to enhance the fault detection performance by performing multi-objective genetic algorithm optimization to minimize missed detection rate, false alarm rate and mean square error of prediction of the output variable. Wavelet function based multi-scale representation further enhances the kernel method due to its ability to effectively separate the deterministic and stochastic features of the data and has the ability to handle the noise, non-normal distribution and auto-correlated data set. Fault detection decision is based on the statistical test that is performed on the residue obtained from the developed model. In our previous work we have showed superior fault detection ability of the composite hypothesis method like generalized likelihood ration test (GLRT) over convention methods like T2 test and Q2 test, in this work we have used moving window based GLRT technique for fault detection.
The proposed MS-MKPLS based MW-GLRT methodology fault detection performance is illustrated through Tennessee Eastman process problem (TEP), which is a continuous process problem based on Eastman chemical company. The fault detection results demonstrate effectiveness of the developed methodology with lower missed detection rate and false alarm rate.
Keywords: KPLS, GLRT, wavelet function, fault detection, Tennessee Eastman process.
References:
1. Botre, Chiranjivi, Majdi Mansouri, Mohamed Nounou, Hazem Nounou, and M. Nazmul Karim. 2016. ÒKernel PLS-Based GLRT Method for Fault Detection of Chemical Processes.Ó Journal of Loss Prevention in the Process Industries 43 (September): 212–24.
2. MacGregor, J.F., and T. Kourti. ÒStatistical Process Control of Multivariate Processes.Ó Control Engineering Practice 3, no. 3 (March 1995): 403–14.
3. Teppola, Pekka, and Pentti Minkkinen. 2000. ÒWavelet–PLS Regression Models for Both Exploratory Data Analysis and Process Monitoring.Ó Journal of Chemometrics 14 (5-6): 383–99.
Presenter(s)
Language
Pricing
Individuals
AIChE Member Credits | 0.5 |
AIChE Pro Members | $19.00 |
Fuels and Petrochemicals Division Members | Free |
AIChE Graduate Student Members | Free |
AIChE Undergraduate Student Members | Free |
AIChE Explorer Members | $29.00 |
Non-Members | $29.00 |