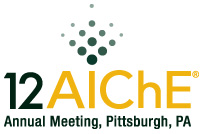
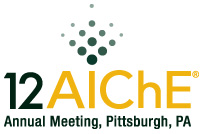
Markov chain Monte Carlo (MCMC) approacheshave provided the computational machinery that has brought Bayesian inference applications to a variety of fields. However, the computational burden that they may require for the inference of complex models involving a considerable number of parameters can be prohibitive. As an alternative, variational approximation methods have been proposed. This family of methods translates the inference problem into an optimization problem by substituting the posterior distribution by a functional form. A decomposition strategy has been developed for the variational approximate inference to deal with models described by differential-algebraic equations (Laínez et al. 2012). In this work we further extend this framework to handle hierarchical models whose number of parameters generally increases with the number of “submodels”. The relationship between the hyperparameters and “sub-model”parameters is exploited in order to apply a Lagrangian based decomposition so as to reduce the computation expense of the optimization problem.
A pharmaceutical reaction kinetic case study is presented to demonstrate the advantages of the proposed approach against MCMC approaches for hierarchical models.
References
Laínez, J.M., L. Mockus, S. Orcun, G. Blau, and G.V. Reklaitis. “A decomposition strategy for the variational inference of complex systems”, submitted to Technometrics (2012).
Acknowledgements
Support from the United States National Science Foundation(Grant NSF-CBET-0941302) is gratefully acknowledged.