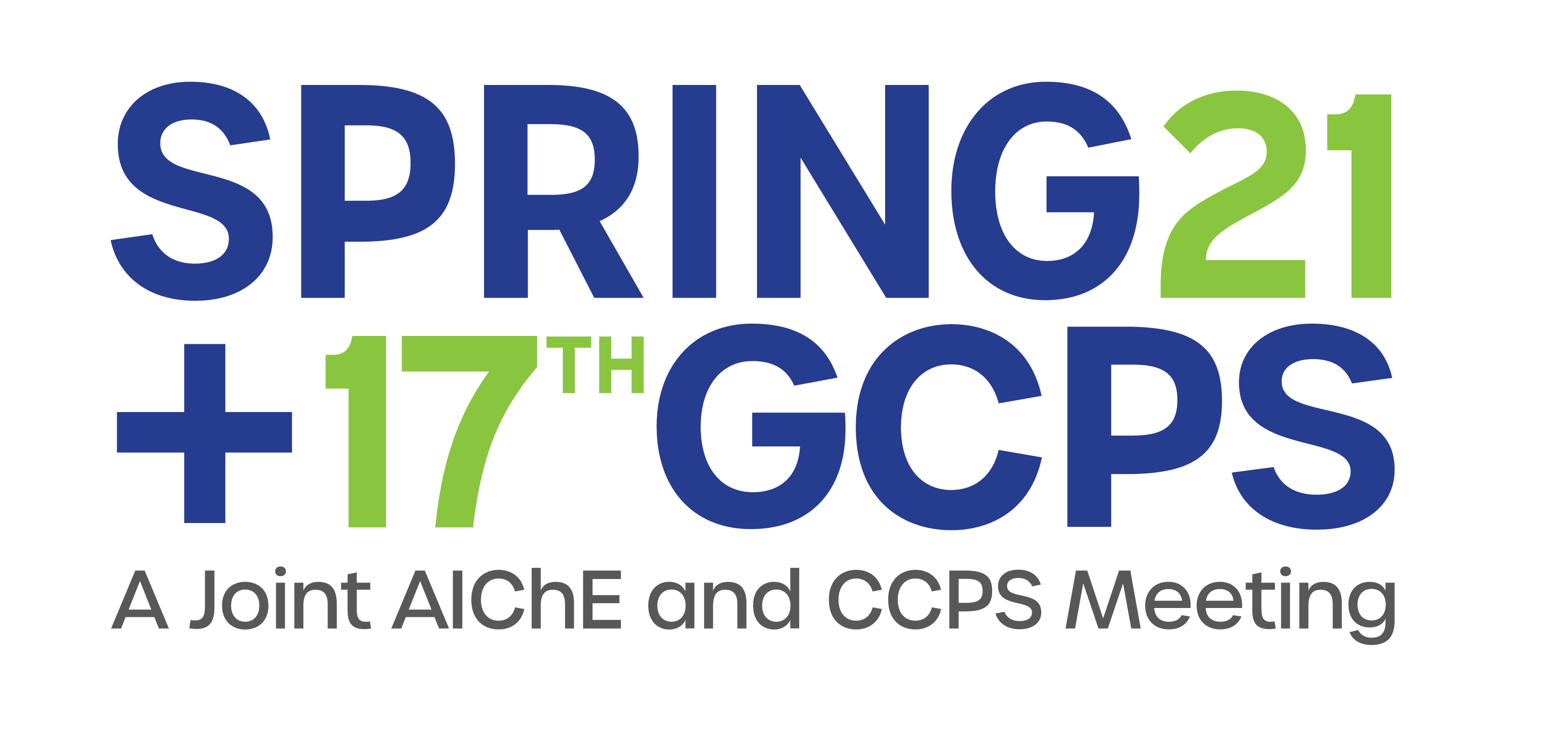
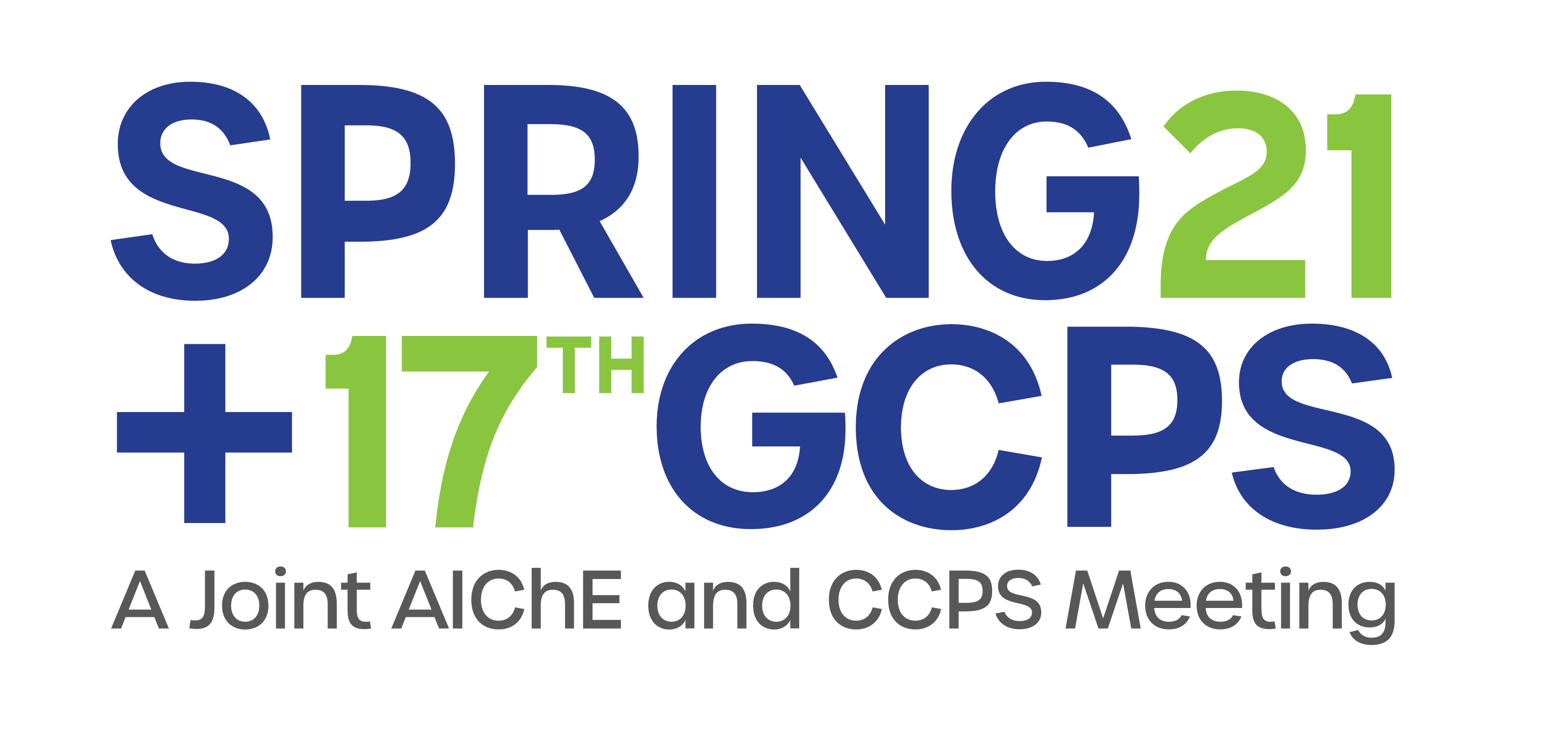
This study is aimed at identifying the contributors of liquid aerosol formulation and solving their data deficiencies by developing the corresponding quantitative structureâproperty relationship models. 1215 liquid chemical substances and 14 predictors have been input to train the machine learning models via k-fold cross validation with the consideration of principal component analysis. Three rounds of comparisons were executed to find the final optimal models for liquid dynamic viscosity (LDV), surface tension (ST) and liquid vapor pressure (LVP). The most persuasive model for LDV is obtained by exponential Gaussian process regression (GPR) approach with seven principal components while the Matern 5/2 GPR algorithm is the most robust one for ST and LVP to formulate the optimal models. Since the reasonably good interpretation and prediction performance provided by the developed models, they can be served as effective tools to expand the database for the liquid aerosol formulation properties of organic compounds.