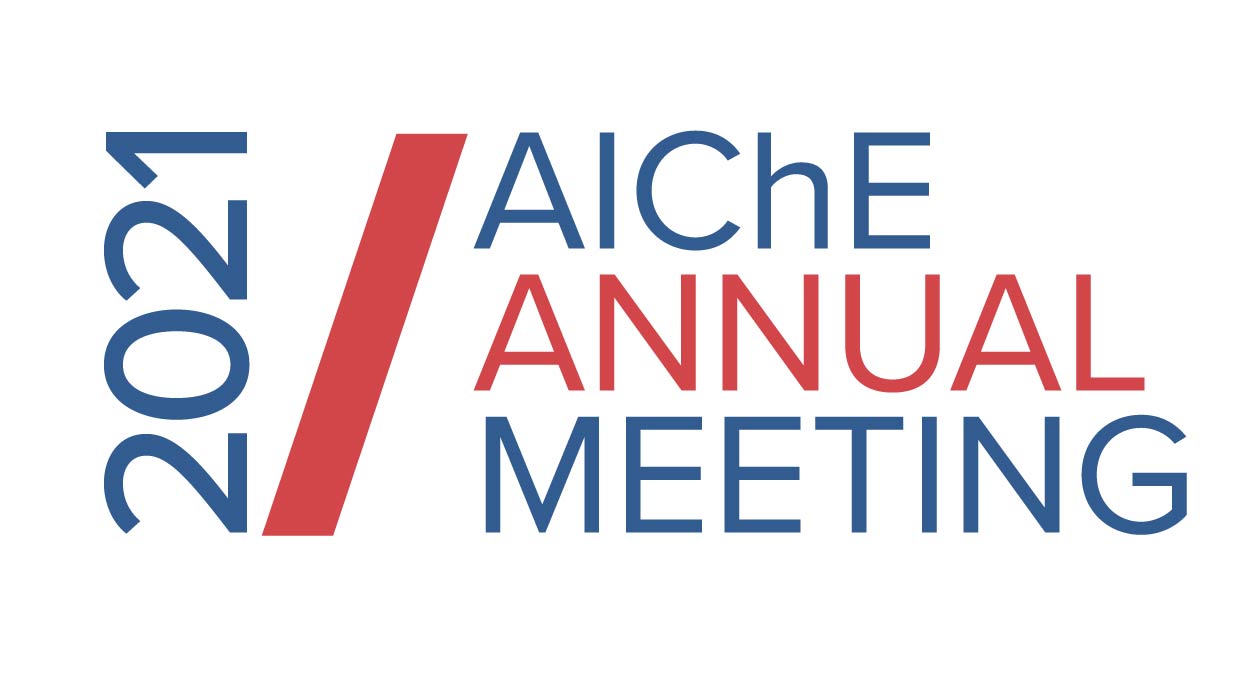
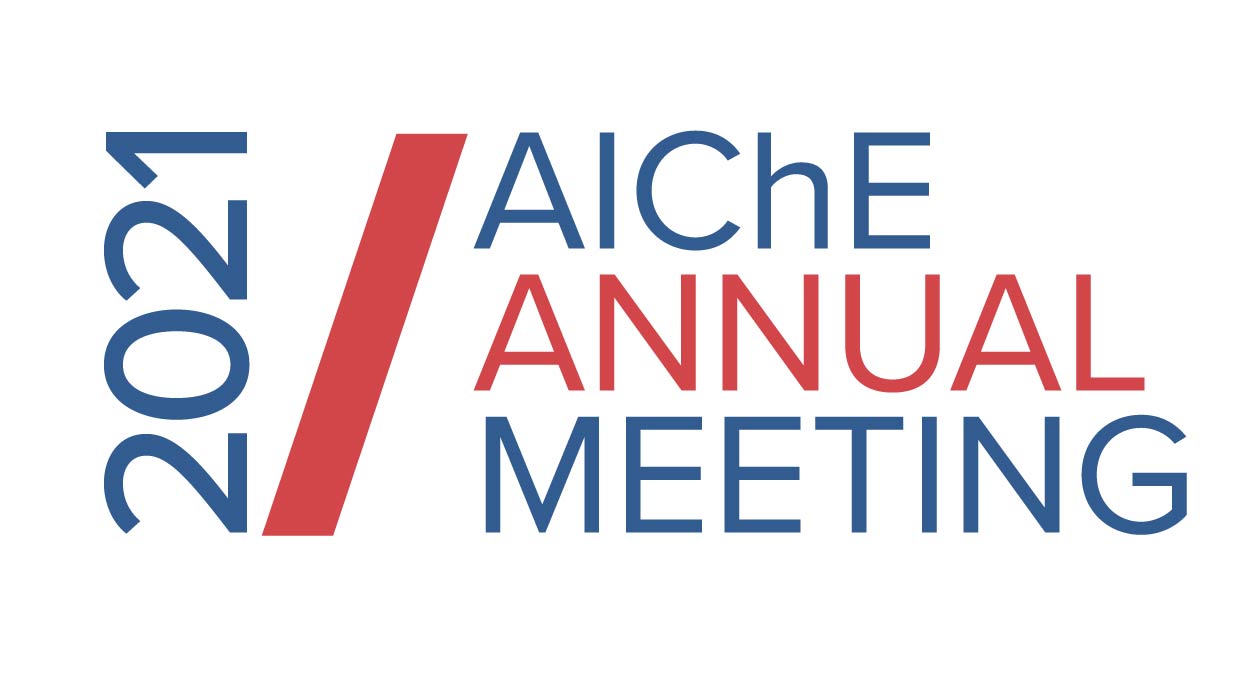
In continuous oral dosage pharmaceutical manufacturing, twin screw wet granulation (TSG) is one process where such a model would be useful. Hindiyeh et al.[1] observed that blending and granulation consume about 40% of the total energy usage in downstream oral dosage pharmaceutical manufacturing. Wet granulation is an energy intensive process since a large amount of shear needs to be applied to the granulating powder blend to obtain good liquid distribution and final granules properties. During lab-scale research, a usual run time of experiments ranges from 10-15 minutes where as manufacturing lines are run longer usually from 8-16 hours. This is when monitoring of energy consumption becomes relevant. Vercruysse et al.[2] in their one-hour long experiments observed that torque required by the system gradually kept increasing till the end of their run. Several studies have optimized the TSG process for obtaining desired granule critical quality attributes (CQAs) [3], [4], but no study has addressed the potential savings in manufacturing costs by optimizing the energy efficiency of the TSG system.
In this work, twin screw granulation experiments were performed and real-time data for process parameters, equipment torque and granule quality attributes were collected using an integrated data infrastructure. This data was then transferred to cloud data lake and was used to train a long-short term memory (LSTM) network to link the real-time process parameters to torque and granule CQAs. To maximize the energy efficiency of the TSG process, the torque applied to the system by the screws needed to be minimized. A physics-constrained supervised autoencoder [5] was used to determine the domain of the process parameters in which the TSG process could be run while still maintaining the granule CQAs. The autoencoder model also predicted the granule growth regime in real-time. After a predetermined time interval, the optimization algorithm was run to determine the new process parameters for the TSG process, which in turn lead to a decrease in the torque levels of the system. This algorithm led to an increase in the energy efficiency of the system by about ~20%, leading to large potential savings in an actual manufacturing scenario.
References
[1] M. Hindiyeh et al., âProcess modification of pharmaceutical tablet manufacturing operations: An eco-efficiency approach,â Processes, vol. 6, no. 2, 2018.
[2] J. Vercruysse et al., âUse of a continuous twin screw granulation and drying system during formulation development and process optimization,â Eur. J. Pharm. Biopharm., vol. 89, pp. 239â247, 2015.
[3] H. Liu et al., âOptimization of critical quality attributes in continuous twin-screw wet granulation via design space validated with pilot scale experimental data,â Int. J. Pharm., vol. 525, no. 1, pp. 249â263, 2017.
[4] H. Liu et al., âDesign space determination and process optimization in at-scale continuous twin screw wet granulation,â Comput. Chem. Eng., vol. 125, pp. 271â286, 2019.
[5] C. Sampat et al., "A physics constrained supervised autoencoder model to predict critical quality attributes of a wet twin screw granulation process" (Under preparation).