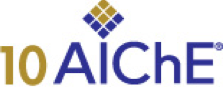
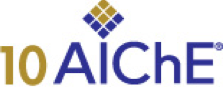
Principal Components Analysis (PCA) is a statistical technique that aims at reducing the problem dimensionality by progressively eliminating redundancy in data. The method has found extensive use in exploratory data analysis as it aids visualization of complex data. Interestingly, the method is data driven which makes it suitable for mechanical learning.
Rotating equipment such as pumps, compressors and turbines are vital equipment in processing plants. Malfunction(s) in the operation of such equipment can have serious and sometimes disastrous consequences. Operation of rotating equipment therefore demands continuous surveillance. However, the current state of affairs is less than satisfactory and would greatly benefit from an easy to implement Early Events Detection (EED) program.
We recently investigated one such a possibility of using PCA for EED; the results are promising and are discussed in this paper.
F&PD Members: Simply click on “click here to buy this archived webcast ” below and your ‘0’ price will appear in your shopping cart.
Language
Pricing
Individuals
AIChE Member Credits | 0.5 |
AIChE Pro Members | $15.00 |
Fuels and Petrochemicals Division Members | Free |
AIChE Graduate Student Members | Free |
AIChE Undergraduate Student Members | Free |
AIChE Explorer Members | $25.00 |
Non-Members | $25.00 |