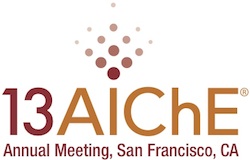
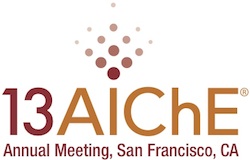
Abstract Quantitative Structure Retention Relationships (QSRR) can play an important role to enhance the speed and quality of chromatographic method development. This paper presents a novel (compound classification based) QSRR modeling strategy which simultaneously accounts for analyte properties , mobile phase conditions and stationary phase properties. It involves the adoption of two models: (A) Partial Least Square - Discriminate Analysis (PLS-DA) to classify compounds into sub-classes having similar interactive relationships between the mobile phase conditions and stationary phase; (B) L- Partial Least Squares (L-PLS) to predict the compound’s retention time based on the mobile phase conditions , stationary phase , and the compound properties. For the retention time of a compound to be modeled , the most favorable compound class is identified in an optimization framework which simultaneously minimizes both the compounds misclassification rate (based on PLS-DA) and the retention time prediction error (based on L-PLS) through a Mixed Integer Optimization. The proposed QSRR model (L-PLS with compound classification) significantly improves the retention time predictability compared with traditional QSRR models or L-PLS models without compound classification. When combined with the Linear Solvation Energy Relationship (LSER) parameters (using Abraham coefficients) as the column properties , the approach allows: (1) prediction of (new , never analyzed) compound retention times under chromatographic conditions (columns and mobile phase conditions) used to train the model; (2) prediction of (previously analyzed under training conditions) compound retention times under chromatographic conditions that have not been previously evaluated; (3) optimization of the chromatographic conditions (mobile phase and column selection) to maximize critical pair resolution , including new compounds; (4) enhanced mechanistic understanding of the interactive retention relationship between compounds , mobile phase and column (e.g. compound retention mechanism). The effectiveness of proposed modeling strategy will be demonstrated through two practical pharmaceutical applications in Supercritical Fluid Chromatography (SFC) and Reversed Phase Liquid Chromatography (RPLC).