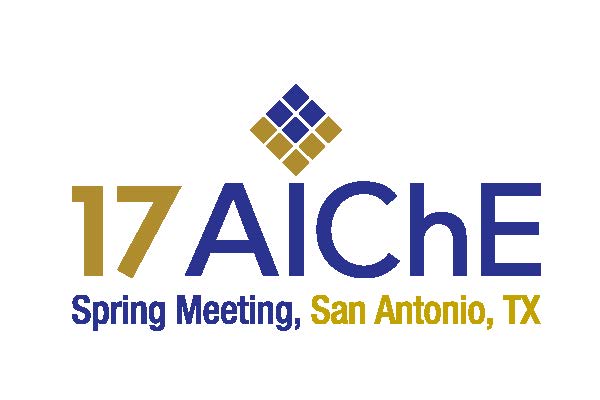
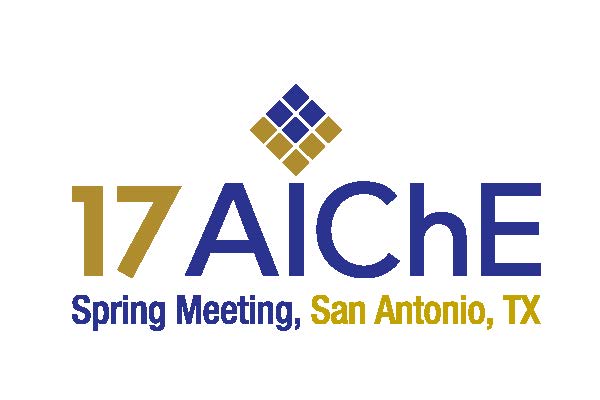
This work presents an approach to perform operational optimisation and retrofit of existing distillation systems. In this approach, the distillation units (CDUs) and HEN are optimised together to obtain cost-effective and acceptable designs. The optimisation approach explores changes in the CDUs and HEN simultaneously, exploiting the synergy within the system.
In this methodology, industrially-relevant variables are manipulated during optimisation; for example, flow rates of distillation products, pump-arounds and stripping steam and split fractions of stream splitters in the HEN. For retrofit, the method allows HEN structural modifications to be constrained to ensure that practicable solutions can be achieved. Variables related to product quality (e.g. ASTM D86 temperatures, flash points, etc.), column flooding or pump capacity can be readily modelled and monitored.
The main feature of this methodology is that artificial neural networks (ANNs), regressed from rigorous process simulation results, are used to represent the distillation process, while Process Integration Limited’s software i-Heat™ is used to represent the HEN. The ANN distillation model and i-Heat™ are linked together to optimise CDUs and HEN simultaneously.
The most recent developments of this approach include a procedure to perform sensitivity analyses to screen undesired operating scenarios (e.g. scenarios where product quality cannot be met), which allows a comprehensive and systematic exploration of the optimisation space. Another development is the implementation of these analysis tools, ANN models and i-Heatâ„¢ into an application software, i-CDUâ„¢, to perform optimisation of the overall distillation system.
This paper presents the application of the methodology to an operational industrial distillation system. This system consists of a flash drum, an atmospheric distillation unit, a vacuum distillation unit and the associated HEN. Operational optimisation for yield improvement is applied to this system considering two types of crude oil feedstock. This methodology is able find solutions that improve product revenue with no capital investment; it is anticipated that these results will be implemented on site.
Presenter(s)
Language
Pricing
Individuals
AIChE Member Credits | 0.5 |
AIChE Pro Members | $19.00 |
Fuels and Petrochemicals Division Members | Free |
AIChE Graduate Student Members | Free |
AIChE Undergraduate Student Members | Free |
AIChE Explorer Members | $29.00 |
Non-Members | $29.00 |