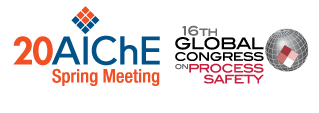
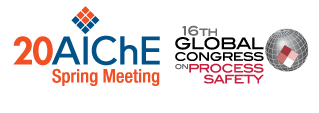
Large volumes of industrial data in conjunction with machine learning provide new opportunities for better decision making and fault prediction in the process industry. We will present a systematic approach to process and synthesize data to build reliable models for fault prediction. We will address questions such as How are the data pre-processed? How do we identify the best model? What are the relevant modern machine learning tools? We will illustrate our approach using a year's worth of data from a real industrial Electric Arc Furnace (EAF) which is widely used for steelmaking and refining particulate ores into base metals. Specifically, we will show how the industrial raw data was pre-processed into a structured form that is amenable to representation learning. We will also illustrate the application of a wide-ranging set of machine learning tools to predict loss of plasma arc in EAF.
Presenter(s)
Once the content has been viewed and you have attested to it, you will be able to download and print a certificate for PDH credits.
If you have already viewed this content,
please click here
to login.
Language
Pricing
Individuals
AIChE Member Credits | 0.5 |
AIChE Pro Members | $19.00 |
AIChE Graduate Student Members | Free |
AIChE Undergraduate Student Members | Free |
Computing and Systems Technology Division Members | Free |
AIChE Explorer Members | $29.00 |
Non-Members | $29.00 |