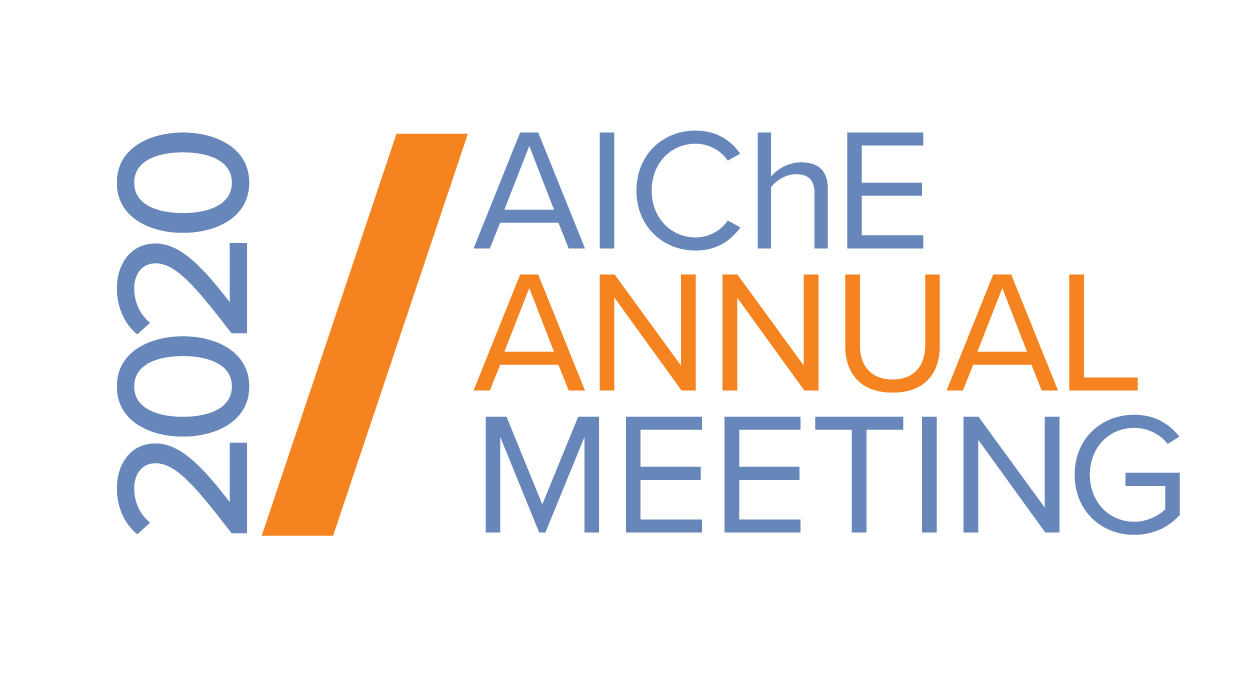
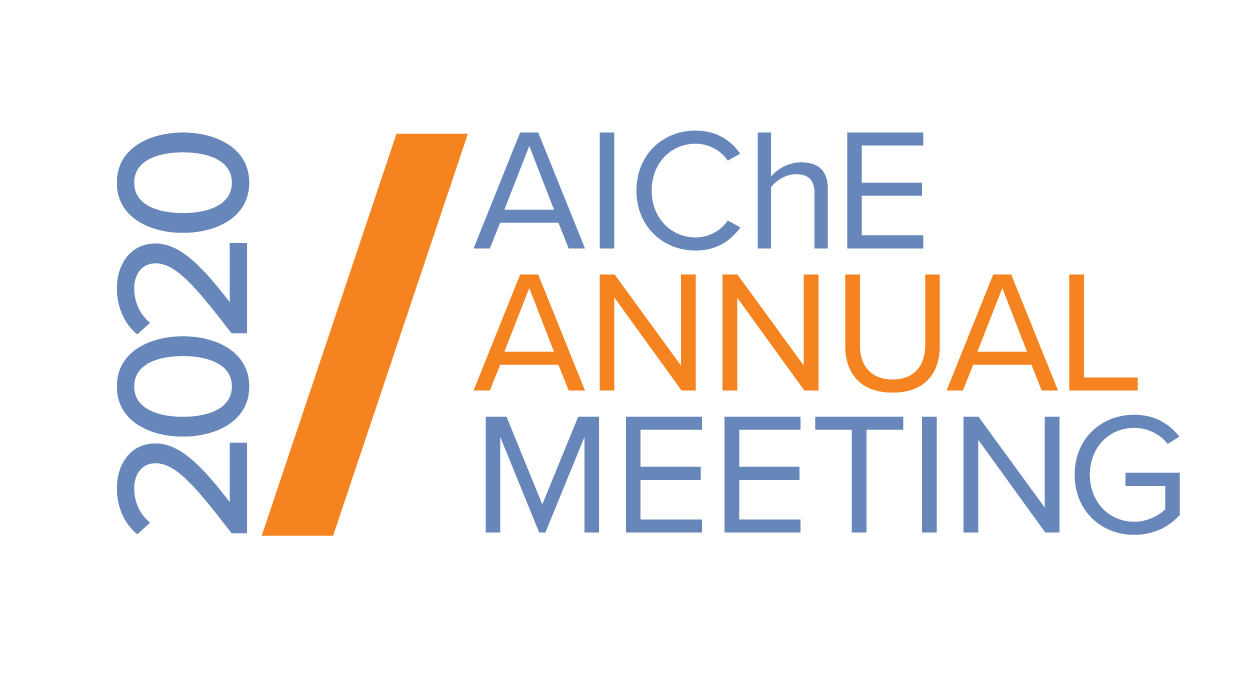
In this paper, we address the problem of large-scale robust state-estimate prediction; that is, the prediction of future values of state estimates robustly at every time instant in large-scale processes. To this end, we decompose a large-scale state-estimate prediction problem into a set of smaller-scale state-estimate prediction problems so that the resulting set of the smaller-scale state-estimate predictors are more robust, easier to design and implement, and more computationally efficient. We also study tuning of the smaller-scale state-estimate predictors to maximize the robustness of the predictors set while ensuring the stability of the error dynamics of the entire set. In addition, we address the problem of implementing the smaller-scale state-estimate predictors in parallel (using a computer with parallel processors) to improve computational efficiency of the state estimate prediction while preserving the accuracy and robustness of state-estimate predictions.
References
[1] J.M. Ali, N.H. Hoang, M.A. Hussain, D. Dochain, Review and classification of recent observers applied in chemical process systems, Computers & Chemical Engineering, 76 (2015) 27-41.
[2] A.K. Jana, A nonlinear exponential observer for a batch distillation, in: 2010 11th International Conference on Control Automation Robotics & Vision, IEEE, 2010, pp. 1393-1396.
[3] M. Soroush, State and parameter estimations and their applications in process control, Computers & Chemical Engineering, 23 (1998) 229-245.
[4] S. Tatiraju, M. Soroush, Nonlinear state estimation in a polymerization reactor, Industrial & engineering chemistry research, 36 (1997) 2679-2690.
[5] N. Zambare, M. Soroush, B.A. Ogunnaike, Robustness improvement in multi-rate state estimation, in: Proceedings of the 2001 American Control Conference.(Cat. No. 01CH37148), IEEE, 2001, pp. 993-998.
[6] N. Abdel-Jabbar, C. Kravaris, B. Carnahan, A partially decentralized state observer and its parallel computer implementation, Industrial & engineering chemistry research, 37 (1998) 2741-2760.
[7] N. Abdel-Jabbar, C. Kravaris, B. Carnahan, Structural analysis and partitioning of dynamic process models for parallel state estimation, in: Proceedings of the 1998 American Control Conference. ACC (IEEE Cat. No. 98CH36207), IEEE, 1998, pp. 3170-3176.
[8] J.B. Carvalho, F.M. Barbosa, Parallel and distributed processing in state estimation of power system energy, in: MELECON'98. 9th Mediterranean Electrotechnical Conference. Proceedings (Cat. No. 98CH36056), IEEE, 1998, pp. 969-973.
[9] R. Ebrahimian, R. Baldick, State estimation distributed processing [for power systems], IEEE Transactions on Power Systems, 15 (2000) 1240-1246.
[10] D.M. Falcao, F.F. Wu, L. Murphy, Parallel and distributed state estimation, IEEE Transactions on Power Systems, 10 (1995) 724-730.
[11] D.B. Pourkargar, M. Moharir, A. Almansoori, P. Daoutidis, Distributed estimation and nonlinear model predictive control using community detection, Industrial & Engineering Chemistry Research, 58 (2019) 13495-13507.