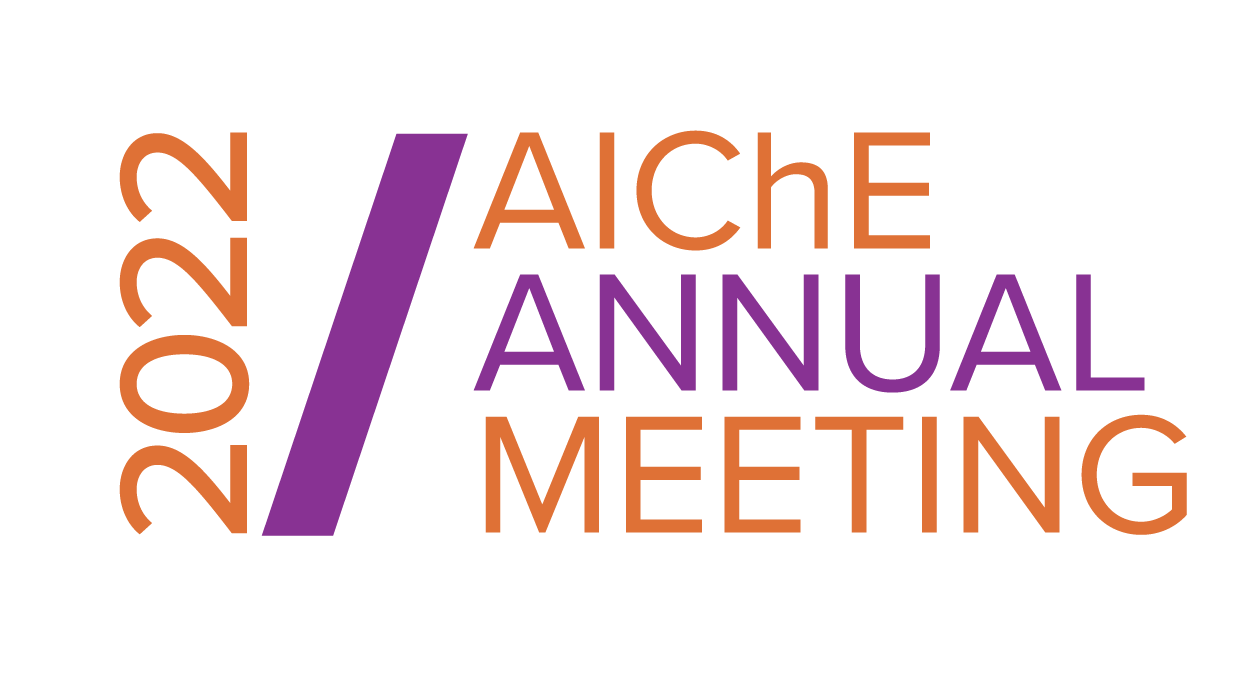
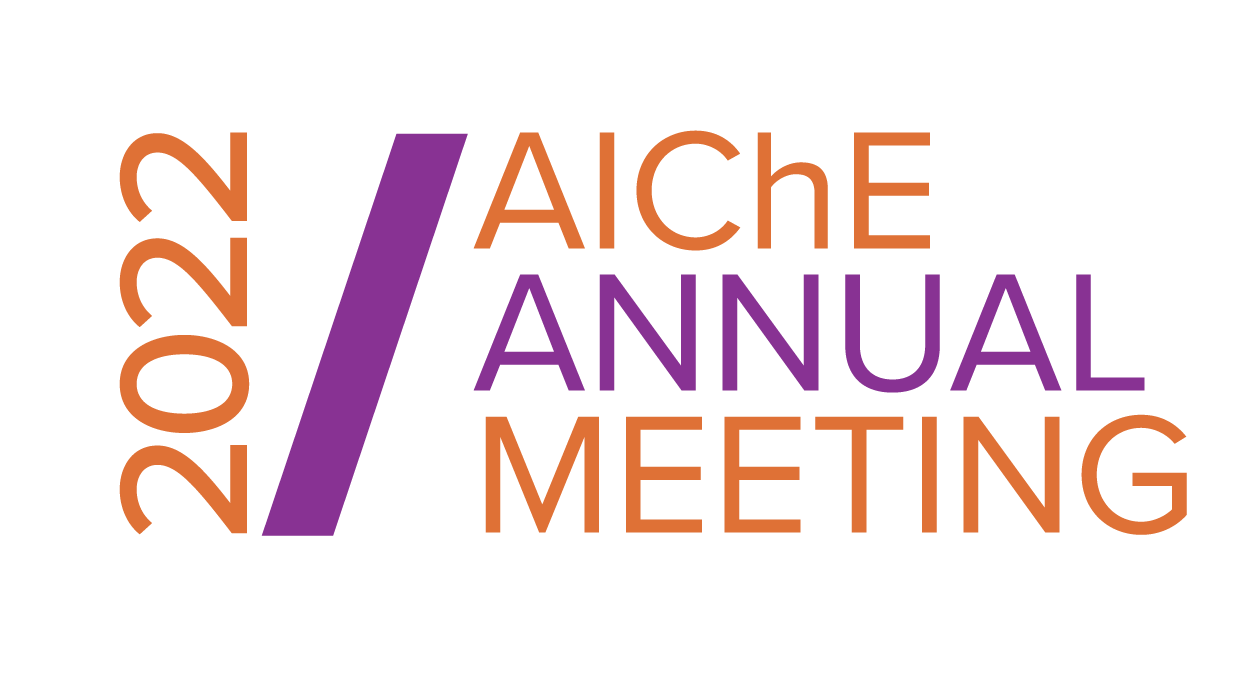
Part 1: Motivated by the urgent need for new Li-ion battery recycling technologies, we explore molecular-to-systems engineering of diafiltration membrane separations. We show how superstructure optimization using science-based mathematical models facilitates systematic evaluation of trade-offs in membrane system design and sets quantitative materials property targets. But this analysis is predicated on science-based models for membrane transport. With this motivation, we then share how synergies between data analytics (e.g., dynamic modeling, nonlinear parameter) and transport experiments (e.g., sensor design for time-series measurements) led to the new Diafiltration Apparatus for high-Throughput Analysis (DATA) technique for higher throughput membrane characterization. We show how Fisher Information-based metrics quantify the information gain for additional measurements and facilitate the optimization of experimentation conditions.
Part 2: Science-based (model-based) design of experiments (SBDOE) seeks to maximize the information gained in proposed experiments conditioned on an assumed model by manipulating experimental degrees of freedom. Despite the rich history of SBDOE applications and algorithmic advances for chemical engineering, these methods are often underutilized due to the need to integrate expertise in domain modeling, applied statistics, and computational optimization. To help reduce these barriers, we introduce Pyomo.DOE, a new open-source Python package, for SBDOE. We highlight important model formulations to improve the numeric robustness of SBDOE optimization. In a case study, we apply SBDOE (using Pyomo.DOE) to optimize fixed-bed breakthrough experiments to calibrate to a partial differential-algebraic equation
(PDAE) model for CO2 adsorption on next-generation metal-organic framework (MOF) materials.
References:
Elvis A. Eugene, William A. Phillip, Alexander W. Dowling (2019). “Data Science-Enabled Molecular-to-Systems Engineering for Sustainable Water Treatmentâ€, Current Opinion in Chemical Engineering, 26, p. 122-130. doi: 10.1016/j.coche.2019.10.002
Jonathan A. Ouimet, Xinhong Liu, David J. Brown, Elvis A. Eugene, Tylar Popps, Zachary W. Muetzel, Alexander W. Dowling, William A. Phillip (2022). “DATA: Diafiltration Apparatus for high-Throughput Analysisâ€, Journal of Membrane Science, 641(1), p. 119743. doi: 10.1016/j.memsci.2021.119743
Jialu Wang, Alexander Dowling (2022), “Pyomo.DOE: An Open-Source Package for Model-Based Design of Experiments in Pythonâ€, AIChE Journal (Early View). doi: 10.1002/aic.17813