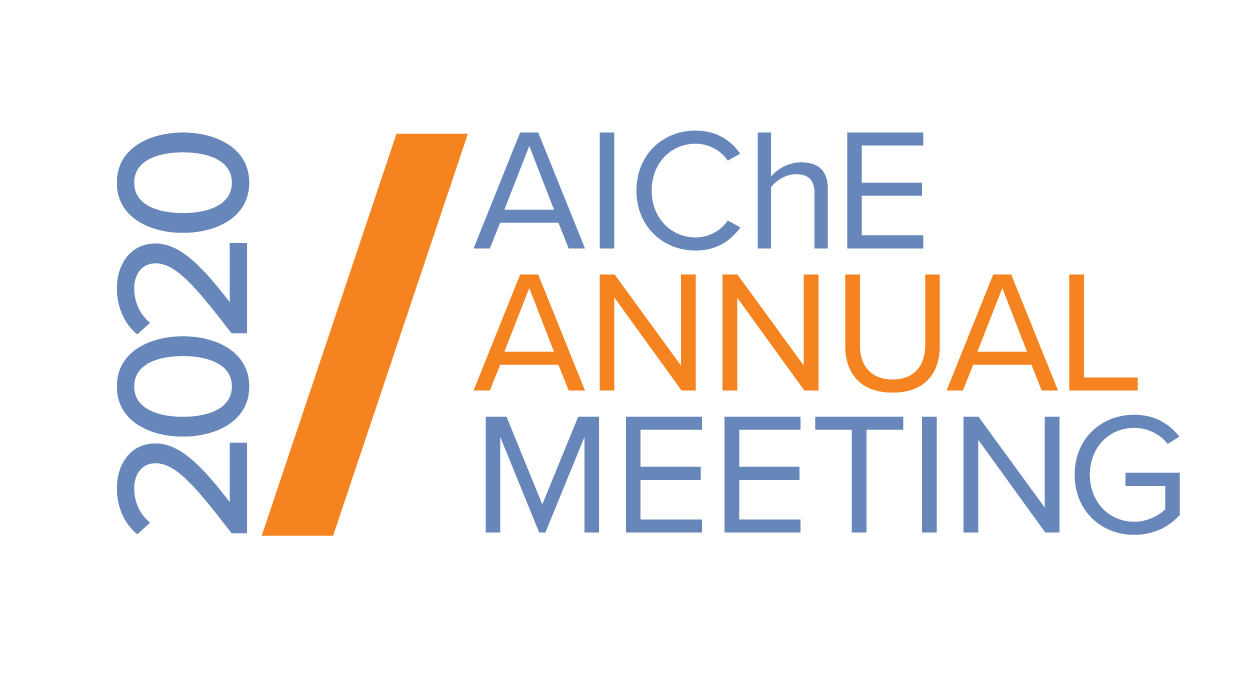
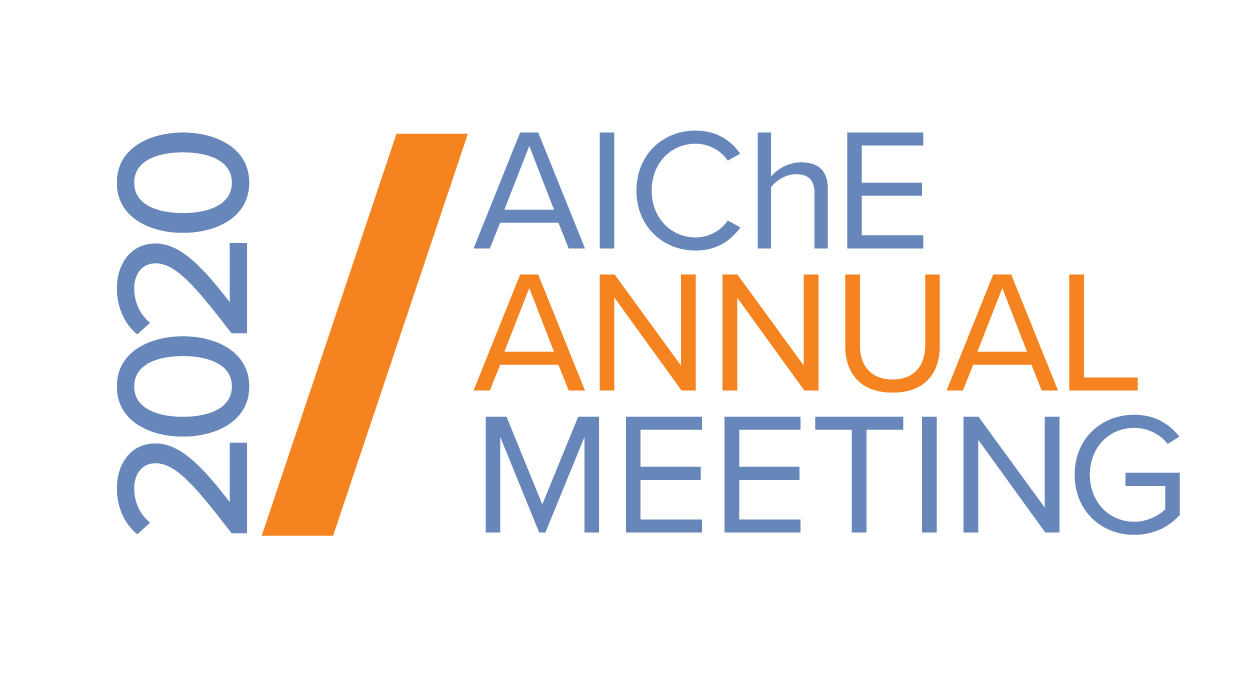
Graph neural networks (GNNs) are models that take graphs as input. GNNs are useful for modeling molecules as graphs because they are permutation invariant, meaning they do not change output if atom order is rearranged. GNNs are revolutionizing modeling of physical systems like the convolutional neural network did for imaging. In this video, we will give an overview of the principles of GNNs. After that, we will discuss their applications in coarse-grained mapping prediction and chemical shifts prediction where in both works, GNNs show good performance. GNNs should be a drop-in replacement for previous molecular feature selection and will grow in popularity.
Presenter(s)
Once the content has been viewed and you have attested to it, you will be able to download and print a certificate for PDH credits.
If you have already viewed this content,
please click here
to login.