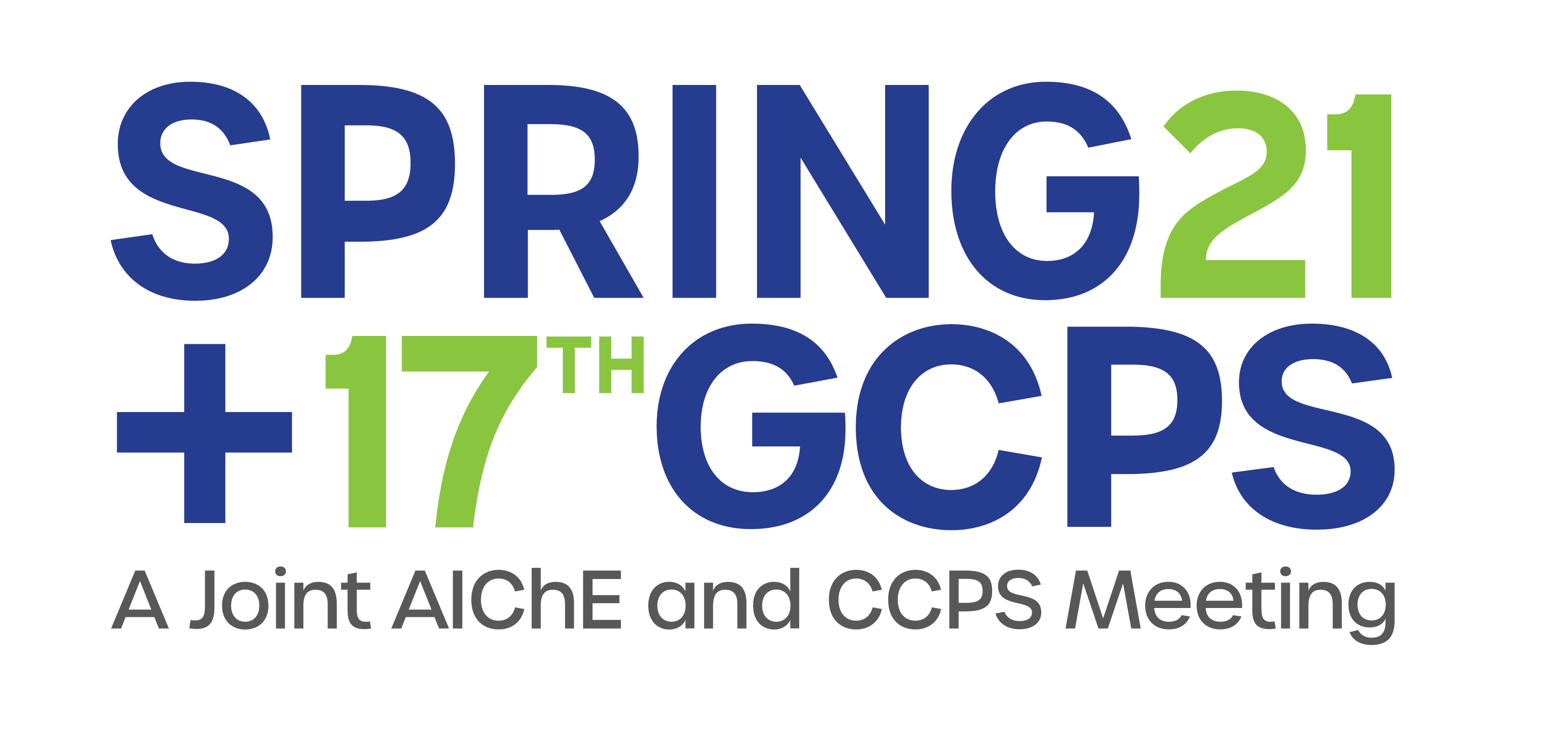
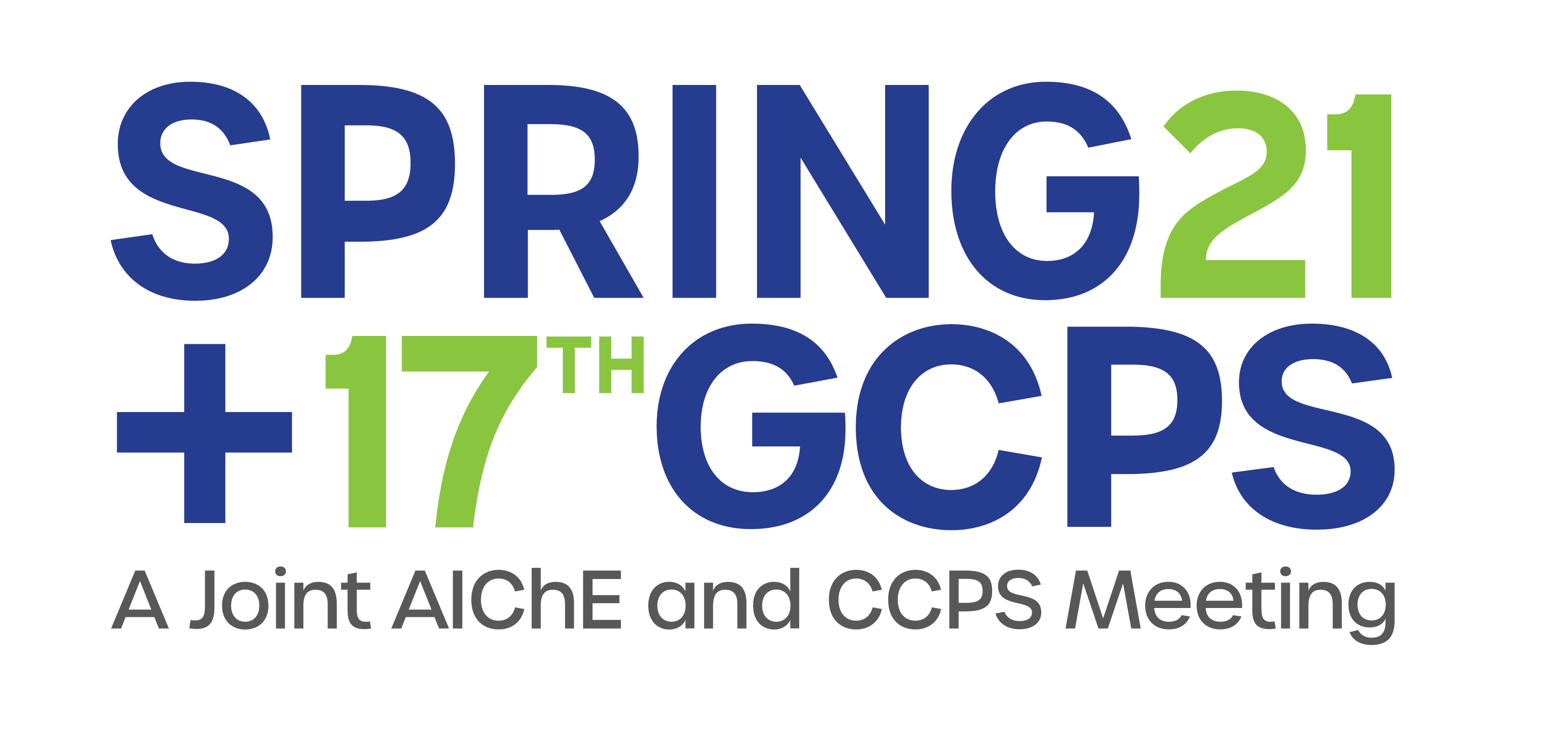
An alternative approach which has the potential to reduce these costs is to automate the review of IRs through the use of data analytics and machine learning (DA/ML). Advances over the past several decades in computational capabilities and computer programming techniques / algorithms now permit application of DA/ML techniques to analyze the large historical data sets that contain information on the performance of SSCs over years of plant operation. Unfortunately, these techniques have not been widely adopted within the nuclear industry due to its highly regulated nature. A consequence of this structure is that any DA/ML models used in applications subject to regulatory oversight must provide a high degree of confidence in the predictions due to potential regulatory impacts associated with an incorrect determination.
The MRFF Analyzer is a DA/ML application which significantly improves the efficiency of the maintenance rule screening process. The model uses a combination of natural language processing (NLP), Bayesian statistics, and machine learning to identify the likelihood that the incident described by an IR represents a MRFF. The software was trained, tested, and validated on four years of historic incident reports (2015-2019) generated across Exelon Generationâs fleet of operating NPPs. This data set consisted of approximately 650,000 IRs. Approximately 420,000 IRs from 2015 through the summer of 2018 were used to train the model, and 80,000 IRs from summer-winter 2018 were used to test the model. Model performance in the field was validated during 2019 with each IR generated by the sites analyzed by the software with review performed by on-site subject matter experts as specified in the plant program implementing procedures. Once the performance of the MRFF Analyzer software was verified as adequate to meet the requirements of the Maintenance Rule, the parallel use of review by human experts was discontinued.
This paper presents the development, training, and deployment of the MRFF Analyzer software across Exelon Generationâs fleet of operating NPPs. The algorithms in use in the software are discussed in detail. The challenges dealing with highly skewed data are presented and the approaches used to address these challenges discussed. The results of a detailed sensitivity assessment are presented and used to estimate the expected statistical performance of the software. The overall performance of MRFF Analyzer since its deployment at Exelon Generation is discussed and estimates of the cost savings are provided.