Editor’s Note: A slightly different version of this article appears as the Perspective article in the April 2016 AIChE Journal (pp. 966–974).
Nanoparticles for drug delivery can be better designed by modeling the body as unit processes connected by the vascular system. Each organ and organ function can be represented as a unit process on organ-on-a-chip devices.
Nanoparticles have emerged as promising vehicles to deliver drugs in the body. Many nanoparticles are intravenously injected and must outsmart several underlying processes taking place within the body — such as filtration in the kidneys, active phagocyte-mediated clearance in the liver, physical entrapment in the lungs, and advection in tumor tissues — before reaching the target site. These processes are linked by the vascular system. Nanoparticles that can navigate through this highly complex biological network have a better chance of delivering drugs to the target tissue.
Intravenous nanoparticle-based drug delivery can be better understood by focusing on the interplay between individual cells, individual organs, and the overall connectivity between each of the organ processes and the cells contained within the organ. It is currently not possible to connect these length scales — i.e., nanoscale (cellular-scale), micro-scale (organ-scale), and macro-scale (organism-scale) — to predict nanoparticle fate and function. However, we can work toward this goal by viewing and analyzing intravenous nanoparticle delivery similar to that of a chemical plant by leveraging the unit process strategy successfully developed and popularized by chemical engineers.
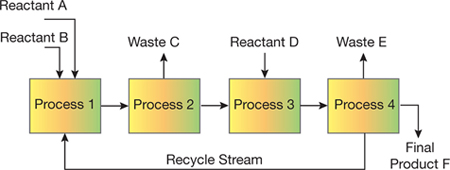
▲Figure 1. The unit process approach successfully used by chemical engineers can be shown as a flowchart with reactants, products, waste, individual unit processes, and a recycle stream.
The unit process approach has allowed chemical engineers to routinely design and analyze complex systems that consist of individual processes (e.g., separators, filters, purifiers, reactors, heat exchangers, refrigerators, dryers) connected via a larger network (Figure 1). The physical principles governing widely used unit processes are well-known, so individual unit processes to manufacture products ranging from petrochemicals to food products can be designed in a predictable manner. An important attribute of the unit process approach is the integration of information across various length and time scales through theoretical modeling, simulations, and semi-empirical correlations. The ability to jump between and relate scales is crucial to ensuring connectivity between the macroscopic and microscopic details.
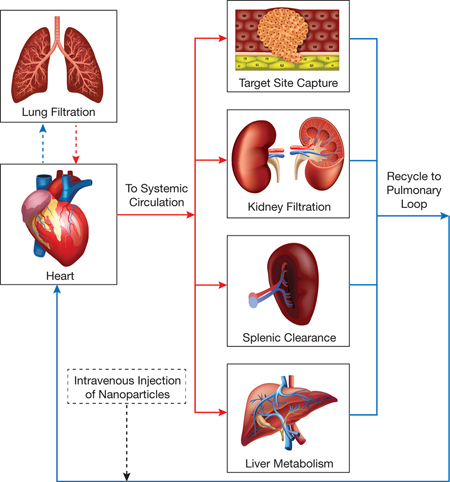
▲Figure 2. Nanoparticle drug delivery can be viewed as a unit process flowchart, with an injection port for the nanoparticles, oxygenated blood flow (red lines), deoxygenated blood flow (blue lines), and individual unit processes connected via vascular circulation.
In principle, each physiological process can be modeled as a unit process (Figure 2), and a system of connected unit processes can be used to determine the fate of nanoparticles traveling through the body. A limitation is that, unlike a true unit process, which can be designed, studied, and modeled in isolation, most physiological processes cannot be analyzed independently. Thus, researchers frequently study nanoparticle movement at the organism level, which often overlooks individual drug-delivery processes and cell-particle interactions. To complement the empirical in vivo results, they may use additional experimental techniques (e.g., static cell culture, perfused organ models), but those methods typically lack the structural complexity of organs and the results cannot be directly related to empirical in vivo results.
Currently, the most relevant method to study organs and organ processes individually is an organ-on-a-chip system. Other methods, such as intravital microscopy, can provide complementary information. However, methods that can connect results from different techniques that involve diverse conditions and different length scales are lacking. Theoretical modeling and computational methods can help address this, especially in relating data between different length scales that are often collected via disjointed experiments. Despite some success of both organ-on-a-chip devices and various computational and modeling methods, investigating organs as individual units to consistently predict nanoparticle performance in a connected system has not been demonstrated.
This article provides an overview of organ-on-a-chip technology and discusses how such devices can be used in a unit process approach to design better drug-delivery nanoparticles. It discusses the most important physiological processes that influence the fate and function of nanoparticles in the body and identifies notable breakthroughs in each area. Finally, it identifies challenges and future directions in using a unit process strategy to design better nanoparticle-based drug-delivery vehicles.
Organ-on-a-chip
Organ-on-a-chip devices (1, 2) can be used to study isolated organ-scale (micro-scale) interactions between nanoparticles and individual organs or organ processes. Although they can reproduce many essential organ functions, these devices are not currently able to predict nanoparticle performance.
One major issue is that it is difficult to replicate on a chip the complexity of each individual organ. While existing organ-on-a-chip systems have been able to mimic many of the essential organ functions, they do not depict several important features — local vascular dynamics, inclusion of relevant cell types (e.g., endothelial cells or circulating blood cells), and physiologically relevant mechanical properties of organs (e.g., elasticity).
Another issue has to do with the way multiple organ-on-a-chip systems are connected, especially since the interplay between the organs and the connecting vascular system is essential to predicting nanoparticle performance. A working synthetic system that connects several organ-on-a-chip systems in a way that mimics in vivo conditions has not been demonstrated.
These issues must be be addressed if a unit process approach to predicting the fate and function of nanoparticles in the body is to be realized.
The organ processes that are most relevant to nanoparticle drug delivery are: vascular circulation, lung and kidney filtration, spleen and liver clearance, and target site capture and distribution. Organ-on-a-chip devices that mimic these functions are important in developing a unit process approach to drug delivery.
Vascular circulation
The vascular system connecting all organ processes (Figure 2) is comprised of two primary segments: the pulmonary loop and the systemic circulatory system. In the pulmonary loop, deoxygenated blood travels from the heart to the lungs for oxygenation; the oxygenated blood travels back to the heart and is then pumped into the systemic circulatory system. Systemic circulation is essentially parallel blood flow through the individual organs, which ensures oxygenated blood can reach all other areas of the body before returning to the pulmonary loop for the cycle to begin again. The vascular system controls the transit and residence times of nanoparticles in each organ, and thus regulates nanoparticle flow, binding, and distribution throughout the whole body.
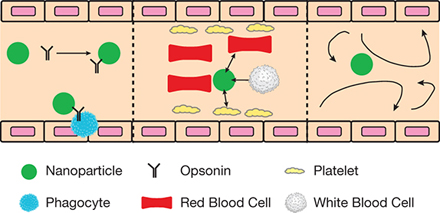
▲Figure 3. Nanoparticles must bypass several features of the various unit processes within the body. Left: Nanoparticles contact opsonins (black), which are antibodies that bind to foreign particles, tagging them to be removed by phagocytes (blue). Center: Interactions with circulatory cells impact the positioning and bulk flow margination of nanoparticles. Right: Nanoparticles encounter complex and unpredictable flow patterns during vascular circulation.
Some of the obstacles that nanoparticles encounter before reaching, binding, and entering the target site involve:
- opsonins, which are proteins in the blood that bind to the nanoparticles and flag them for removal by the immune system (Figure 3, left)
- other particulates in the blood, such as circulatory cells, that can exclude nanoparticles from bulk flow and influence nanoparticle position in the blood vessels, which significantly impacts nanoparticle binding (Figure 3, center)
- complex flow profiles, notably in the heart and pathological tissues, which impact particle margination (i.e., the movement of particles toward the walls of a channel), binding, and subsequently treatment efficacy (Figure 3, right).
Microfluidic organ-on-a-chip systems have been developed to depict the essential flow properties of micro-circulation under several conditions. For example, studies have reproduced the geometry of the vascular system to investigate the role that nanoparticle parameters play in modulating adhesion and binding as a result of geometrical constraints (3). More recently, biological features have been included in vascular chips, including vessel layout from actual tissues (4), endothelial cells lining vessels (5), permeable endothelia structures (6), opsonins and other plasma proteins (7), and circulatory cells (e.g., red blood cells and white blood cells) (8).
Lung and kidney filtration
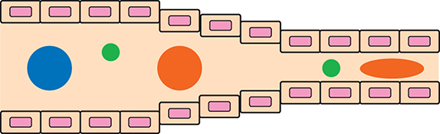
▲Figure 4. The lungs filter particles by size. Large particles (blue) become trapped, whereas smaller particles (green) and flexible particles (orange) can freely travel through the lungs.
The lungs act as a natural filtration unit in the body. This filtration is size-dependent and is facilitated by two unique characteristics of the lungs: tiny capillaries that can be as small as 500 nm in diameter, and the large endothelial surface area. Nanoparticles should be designed to avoid lung filtration in order to prevent the uptake of drugs in healthy cells and capillary clogging, which will impair blood flow, reduce the efficiency of oxygen delivery, and instigate the formation of microthrombi. Thus, smaller-diameter and flexible particles are more desirable to avoid passive (and likely non-desirable) uptake and persistence in lung tissue (Figure 4).
The kidneys act as an ultrafiltration system that separates blood flow and Bowman’s capsules, which are waste streams that empty into the urine. Hydrostatic pressure in the capillaries drives the ultrafiltration, while the pores located between the endothelial cells in the capillaries and the podocytes in the Bowman’s capsules influence the flow of filtrate and retentate. There are advantages (e.g., removal of nondegradable particulates) and disadvantages (e.g., premature removal of drug) to renal filtration and clearance of nanoparticles, and these must be carefully considered when designing nanopaticles for drug delivery.
The most basic organ-on-a-chip systems that incorporate filtration features use channels that are smaller in diameter than the nanoparticles being investigated to mimic the capillary geometry typically encountered in the lungs (9). More advanced systems include pressure differential effects arising in the lung’s capillary cells (10).
Organ-on-a-chip filtration systems have incorporated additional physiological features of the lungs, such as the air-blood interface. For example, one device included an alveolar-endothelial cell barrier on a porous membrane capable of mimicking breathing motions (11). That chip was able to accurately portray organ responses to bacteria, nanoparticles, and inflammatory insults. Kidney-on-a-chip systems mimic the interface separating blood flow and excreted waste, and include features that simulate the effects of renal tubular cells in regulating the water-ion balance (12) and transepithelial transport (13).
Spleen and liver clearance
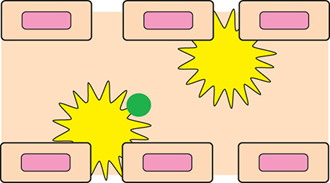
▲Figure 5. The sinusoids in the spleen and liver are lined with macrophages (yellow), which clear the bloodstream of toxins, including foreign nanoparticles (green).
Of all the organs, the spleen and liver (Figure 5) have the most significant impact on removing particles from circulation. Thus, it is essential to include these organs in organ-on-a-chip devices. Both organs receive blood flow through sinusoids — open-ended blood vessels that take the place of capillaries in the liver and spleen. The sinusoids are lined with macrophages, which clear the bloodstream of toxins, including foreign nanoparticles. Once the macrophages have sequestered the foreign particles in either the liver or the spleen, the particles are unlikely to return to systemic circulation; therefore, it is highly desirable for nanoparticles to avoid direct interactions with macrophages.
One active area of nanoparticle research focuses on determining the impact of particle parameters (e.g., size, shape, surface charge) (14, 15) on uptake and subsequent clearance of nanoparticles by phagocytic cells in the liver and spleen.
To mimic the structure of the liver, scientists have used a decellularized whole liver to harvest intact vascular trees, which served as a template for the synthesis of a bio-mimetic liver scaffold that was seeded with human liver and endothelial cells (16). Recently, scientists developed a liver-on-a-chip that mimicked functional characteristics of the liver, including the production of vital biological molecules (17). A spleen-on-a-chip was developed to recreate the hydrodynamic effects in the spleen (18).
Target site capture and distribution
Target sites include tumors, wounds, atherosclerotic plaques, and tissues residing beyond a difficult-to-breach barrier (e.g., the blood-brain barrier). Each target site has unique features that should be considered when designing nanoparticles to ensure specific and efficient targeting and uptake. For example, tumors can have porous endothelial barriers that nanoparticles are likely to diffuse through to reach the tumor. Other target sites can overexpress certain biomarkers that provide a ligand-receptor-mediated means to target diseased tissues.
Microfluidic technologies have been used to mimic target tissues and their individual characteristics. For example, devices mimicking pathological tissues ranging from tumors to wound sites to the blood-brain barrier have been designed and extensively reviewed (19–21).
Organ-on-a-chip devices predict in vivo results
Several technologies portraying individual drug-delivery unit processes on a chip have successfully predicted or described in vivo results.
An organ-on-a-chip depicting a microfluidic vascular model capable of modulating permeability at an endothelial interface demonstrated that nanoparticle translocation increases with increasing permeability.
A tumor-on-a-chip utilizing tumor spheroids was developed to analyze and describe the real-time accumulation of nanoparticles in tumor cells under physiological flow conditions. The device showed, in vitro, that nanoparticle penetration and peripheral accumulation is mediated by both nanoparticle size and receptor-mediated interactions, and that smaller antibody-functionalized particles are better able to accumulate in a tumor’s interstitial spaces. These findings were strongly supported by in vivo studies. Furthermore, scientists found that increased flowrate corresponded to an increase in drug accumulation in the tumor. Such an observation cannot be captured with an in vivo mouse model (22).
Nanoparticles are subjected to complex flow, shear, and other forces and interactions in the vasculature. Studies have investigated these parameters in microfluidic devices in attempts to modulate nanoparticle interactions with diseased, or even healthy, tissues.
An in vitro microfluidic chip of an endothelial wound was designed to simulate relevant biochemical and bio-physical characteristics, as well as interactions stemming from competitive binding by circulatory cells. In this device, a surface coated with collagen and a von Willebrand factor (a blood glycoprotein involved in hemostasis) designed to mimic an endothelial wound was exposed to a mixture of targeted hemostatic nanoparticles and activated platelets, both of which readily bind to the wound-mimicking surface and to each other. As expected, because these particles target both the wound and each other, they caused more clot formation than nanoparticles that only target the wound. The results were validated with an in vivo tail-amputation model (23).
A recently reported organ-on-a-chip mimicked the shear effects of pathological sites and vessel constrictions to investigate shear-responsive nanoparticles. Shear-responsive, clot-dissolving nanoparticles introduced as a large aggregate dispersed into numerous individual particles when exposed to high shear forces in the microfluidic organ-on-a-chip device. This effect was confirmed by in vivo results (24).
Other organ-on-a-chip devices have elucidated fundamental particle design parameters. For example, a device that included filtration slits highlighted how particle elasticity can modulate passage through the slits. This result was reproduced in vivo, where harder particles were passively trapped in lung capillaries. Overall, these examples emphasize the ability of existing organ-on-a-chip devices to depict complex biological phenomena and also predict and explain a nanoparticle’s effectiveness.
Theory and simulation: Organ-scale interactions
While organ-on-a-chip devices provide valuable information at the micro (organ) scale, they are not as useful for obtaining information at the nano (cellular) scale and the macro (organism) scale. Scientists have developed theoretical and computational models to describe, connect, and predict drug-delivery processes at both the nano and macro scales (25, 26). Of particular interest are: pharmacokinetic modeling, which involves experiments that provide physiologically relevant quantifiable values (e.g., half-lives, compartment volumes, clearance rates, etc.) to describe the circulation and distribution of nanoparticles throughout the body; and theoretical and computational methods, which use thermodynamic or atomistic and molecular models to describe individual nanoparticle-cell interactions.
One of the main limitations of pharmacokinetic models and computational methods for studying nanoparticle interactions with single cells is that they are based on several assumptions. Pharmacokinetic modeling, for example, simplifies the body structure by grouping similar tissues and organs into compartments. Because of this simplification, pharmacokinetic models can yield inconsistent results regarding the distribution of nanoparticles in tissues. Computational modeling, on the other hand, typically involves assumptions about the physical and/or chemical features of both nanoparticles and cells. Such assumptions can mistakenly place emphasis on less-relevant cell or particle parameters.
Efforts are moving forward to address these issues and it is likely that a unit process view of nanoparticle drug delivery, with the ultimate goal of providing predictive abilities for the design of future nanoparticles, will be possible by leveraging organ-on-a-chip devices in tandem with theoretical and computational modeling.
Theory and simulation: Cellular scale and cell-nanoparticle interactions
In nanoparticle drug delivery, individual interactions between cells and nanoparticles occur at the nanoscale and are ultimately responsible for the delivery of therapeutics to diseased cells. These interactions can encompass essential processes, such as cellular uptake, endosomal escape, drug dissociation from the nanoparticle, cytoplasmic transport, and target-organelle interactions (e.g., nuclear entry). While these individual processes can be studied in vitro, both qualitatively and quantitatively, it is quite challenging to determine the parameters responsible for the performance differences observed for different nanoparticle formulations.
Theoretical studies are best suited to describe these phenomena. Theoretical methods require key features of the nanoparticle (e.g., size, shape, elasticity, charge, hydrophobicity) and the interacting cell membrane (e.g., lipid molecules, proteins, elasticity, thickness) to be defined, as well as a modeling method (e.g., atomistic, unified-atom force fields, coarse-graining) that describes cell-nanoparticle interactions to be chosen.
By defining these parameters and simulating the interactions between nanoparticles and cell membranes, it is possible to draw general conclusions and extract specific details regarding cell-particle interactions at the nanoscale. For example, computational methods have been used to define minimum particle sizes and minimum ligand densities that prevent endocytosis (27). Other studies have leveraged computational methods to define internalization rates as a function of ligand coatings in receptor-mediated endocytosis and to provide quantitative insights into receptor-ligand binding strengths (28). Nanoscale phenomena captured by theoretical modeling of cellular internalization include the total number of particles internalized in an individual cell, optimal particle parameters based on energetics, shape effects, elasticity (both cell and nanoparticle) effects, surface property effects, and environmental effects, to name a few. Other nanoscale processes worth including in a unit process approach, such as endosomal escape, microtubule trafficking, and nuclear entry, would benefit from a theoretical and computational approach. However, few studies focusing on these processes have been conducted.
Theory and simulation: Organism scale and physiological interactions
The organism scale encompasses processes such as circulation and distribution of nanoparticles in the body. While quantitative values for time-dependent tissue distribution or circulation persistence can be directly analyzed, gaps between time points or missing information at long times cannot be exactly determined. Likewise, semi-empirical results often lack a fundamental basis. In general, animal experiments can be combined with pharmacokinetic modeling to fill in many of the blanks that often arise from unknown phenomena, which cannot be recreated in an organ-on-a-chip. Pharmacokinetic modeling has been used to describe the distribution and circulation properties of small-molecule drugs, and this approach has been extended to nanoparticle drug-delivery systems to determine parameters such as half-lives and clearance rates (29).
The pharmacokinetic modeling method considers each organ or group of organs as a single compartment, and the flow between compartments is governed by mass transport laws — similar to a unit process flowchart. An advantage of pharmacokinetic modeling is that it allows for direct comparisons of nanoparticle formulations with regard to their circulation rates and distribution. More sophisticated models have included transient vascularization of tumors and the specific accumulation of nanoparticles in tumor vasculature (30). Other pharmacokinetic models have been used to investigate the role of nanoparticle physical properties in modulating biodistribution or circulation times. In addition, scientists have combined results from multivariate regression with pharmacokinetic models to predict the biological performance of nanoparticles, and their predictions agreed with experimental data.
Pharmacokinetic models can be used to assign quantifiable values to empirical in vivo results, compare nanoparticle formulations, and in some cases predict the in vivo performance of nanoparticles prior to in vivo testing, which is essential in correlating organism-scale in vivo results with connected organ-on-a-chip systems.
Future outlook
Microfluidic technologies are capable of recreating essential physiological features to mimic organs, organ functions, and diseased tissues. Researchers have leveraged these devices to extract fundamental information regarding nanoparticle interactions with drug-delivery unit processes, and in some cases to predict in vivo performance. Applying a unit process approach to successfully predict the fate and function of nanoparticles, however, will require more advanced organ-on-a-chip systems.
Advanced chips will include multiple processes connected with synthetic vascular channels in a body-on-a-chip system. Results from these chips can be combined with theoretical and simulation results that describe individual cell-particle interactions at the cellular-scale or with information obtained from empirical animal studies at the organism-scale. A unit process approach that collectively includes these strategies will prove capable of not only accurately predicting the biological fate and performance of nanoparticles, but also describing empirical results from in vivo experiments.
As technological advances allow the synthesis and implementation of a connected model, the emphasis of nanoparticle drug-delivery research and development will shift from circulation times and tissue targeting to theoretical modeling of the physical, chemical, and molecular details to optimize nanoparticle delivery for specific diseases. This is similar to chemical process optimization software leveraging knowledge from all relevant length scales to enable the design of an industrially relevant plant of connected unit processes.
Literature Cited
- Bhise, N. S., et al., “Organ-on-a-Chip Platforms for Studying Drug Delivery Systems,” Journal of Controlled Release, 190, pp. 82–93 (Sept. 2014).
- Björnmalm, M., et al., “Engineering and Evaluating Drug Delivery Particles in Microfluidic Devices,” Journal of Controlled Release, 190, pp. 139–149 (Sept. 2014).
- Doshi, N., et al., “Flow and Adhesion of Drug Carriers in Blood Vessels Depend on their Shape: A Study Using Model Synthetic Microvascular Networks,” Journal of Controlled Release, 146 (2), pp. 196–200 (Sept. 2010).
- Kolhar, P., et al., “Using Shape Effects to Target Antibody-Coated Nanoparticles to Lung and Brain Endothelium,” Proceedings of the National Academy of Sciences, 110 (26), pp. 10753–10758 (June 25, 2013).
- Thompson, A. J., and O. Eniola-Adefeso, “Dense Nanoparticles Exhibit Enhanced Vascular Wall Targeting over Neutrally Buoyant Nanoparticles in Human Blood Flow,” Acta Biomaterialia, 21, pp. 99–108 (July 15, 2015).
- Kim, Y., et al., “Probing Nanoparticle Translocation Across the Permeable Endothelium in Experimental Atherosclerosis,” Proceedings of the National Academy of Sciences of the United States of America, 111 (3), pp. 1078–1083 (Dec. 6, 2013).
- Namdee, K., et al., “Differential Impact of Plasma Proteins on the Adhesion Efficiency of Vascular-Targeted Carriers (VTCs) in Blood of Common Laboratory Animals,” Bioconjugate Chemistry, 26 (12), pp. 2419–2428 (Dec. 15, 2015).
- Thomas, A., et al., “Characterization of Nanoparticle Delivery in Microcirculation Using a Microfluidic Device,” Microvascular Research, 94, pp. 17–27 (July 2014).
- Merkel, T. J., et al., “Using Mechanobiological Mimicry of Red Blood Cells to Extend Circulation Times of Hydrogel Microparticles,” Proceedings of the National Academy of Sciences of the United States of America, 108 (2), pp. 586–591 (Jan. 11, 2011).
- Cui, J., et al., “Super-Soft Hydrogel Particles with Tunable Elasticity in a Microfluidic Blood Capillary Model,” Advanced Materials, 26 (43), pp. 7295–7299 (Sept. 10, 2014).
- Huh, D., et al., “Microengineered Physiological Biomimicry: Organs-on-Chips,” Lab on a Chip, 12 (12), pp. 2156–2164 (May 3, 2012).
- Jang, K. J., and K. Y. Suh, “A Multi-Layer Microfluidic Device for Efficient Culture and Analysis of Renal Tubular Cells,” Lab on a Chip, 10 (1), pp. 36–42 (Jan. 7, 2010).
- Jang, K. J., et al., “Fluid-Shear-Stress-Induced Translocation of Aquaporin-2 and Reorganization of Actin Cytoskeleton in Renal Tubular Epithelial Cells,” Integrative Biology: Quantitative Biosciences from Nano to Macro, 3 (2), pp. 134–141 (Feb. 6, 2011).
- Walkey, C. D., et al., “Nanoparticle Size and Surface Chemistry Determine Serum Protein Adsorption and Macrophage Uptake,” Journal of the American Chemical Society, 134 (4), pp. 2139–2147 (Feb. 1, 2012).
- Champion, J., and S. Mitragotri, “Role of Target Geometry in Phagocytosis,” Proceedings of the National Academy of Sciences,” 103 (13), pp. 4930–4934 (Feb. 6, 2006).
- Baptista, P. M., et al., “The Use of Whole Organ Decellularization for the Generation of a Vascularized Liver Organoid,” Hepatology, 53 (2), pp. 604–617 (Feb. 2011).
- Rigat-Brugarolas, L. G., et al., “A Functional Microengineered Model of the Human Splenon-on-a-Chip,” Lab on a Chip, 14 (10), pp. 1715–1724 (May 21, 2014).
- Rennert, K., et al., “A Microfluidically Perfused Three Dimensional Human Liver Model,” Biomaterials, 71, pp. 119–131 (Dec. 2015).
- Prabhakarpandian, B., et al., “Synthetic Tumor Networks for Screening Drug Delivery Systems,” Journal of Controlled Release, 201, pp. 49–55 (Mar. 10, 2015).
- Ravikumar, M., et al., “Mimicking Adhesive Functionalities of Blood Platelets using Ligand-Decorated Liposomes,” Bioconjugate Chemistry, 23 (6), pp. 1266–1275 (June 20, 2012).
- Prabhakarpandian, B., et al., “SyM-BBB: A Microfluidic Blood Brain Barrier Model,” Lab on a Chip, 13 (6), pp. 1093–1101 (Mar. 21, 2013).
- Albanese, A., et al., “Tumour-on-a-Chip Provides an Optical Window into Nanoparticle Tissue Transport,” Nature Communications, 4 (2718), (Oct. 21, 2013).
- Anselmo, A. C., et al., “Platelet-Like Nanoparticles: Mimicking Shape, Flexibility, and Surface Biology of Platelets to Target Vascular Injuries,” ACS Nano, 8 (11), pp. 11243–11253 (2014).
- Korin, N., et al., “Shear-Activated Nanotherapeutics for Drug Targeting to Obstructed Blood Vessels,” Science, 337 (6095), pp. 738–742 (Sept. 21, 2012).
- Nestorov, I., “Whole Body Pharmacokinetic Models,” Clinical Pharmacokinetics, 42 (10), pp. 883–908 (2003).
- Ding, H. M., and Y. Q. Ma, “Theoretical and Computational Investigations of Nanoparticle-Biomembrane Interactions in Cellular Delivery,” Small, 11 (9–10), pp. 1055–1071 (2015).
- Yuan, H., and S. Zhang, “Effects of Particle Size and Ligand Density on the Kinetics of Receptor-Mediated Endocytosis of Nanoparticles,” Applied Physics Letters, 96 (3), p. 033704 (2010).
- Ding, H. M., and Y. Q. Ma, “Role of Physicochemical Properties of Coating Ligands in Receptor-Mediated Endocytosis of Nanoparticles,” Biomaterials, 33 (23), pp. 5798–5802 (Aug. 2012).
- Li, M., et al., “Physiologically-Based Pharmacokinetic Modeling of Nanoparticles,” ACS Nano, 4 (11), pp. 6303–6317 (2010).
- Frieboes, H. B., et al., “A Computational Model for Predicting Nanoparticle Accumulation in Tumor Vasculature,” PLOS One, 8 (2), e56876 (Feb. 28, 2013).
Copyright Permissions
Would you like to reuse content from CEP Magazine? It’s easy to request permission to reuse content. Simply click here to connect instantly to licensing services, where you can choose from a list of options regarding how you would like to reuse the desired content and complete the transaction.