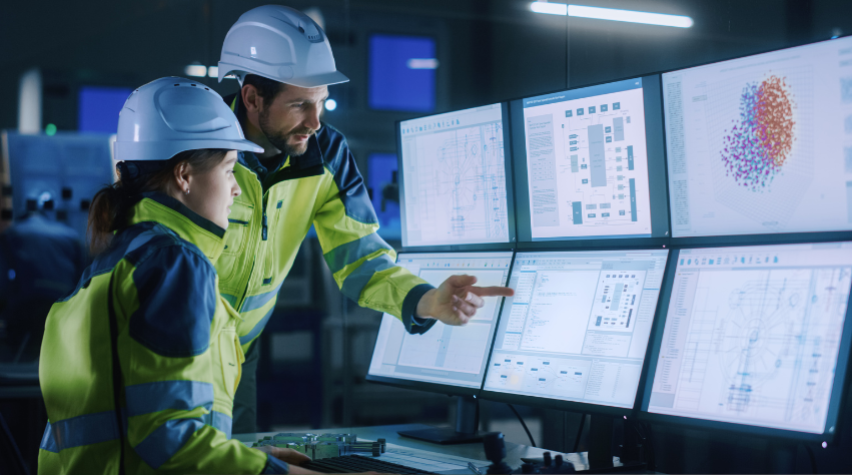
Co-authored by Luke Achenie and Dr. Phillip R. Westmoreland
A previous ChE in Context column (CEP, July 2024, pp. 24–25) and CEP's August 2024 special section on AI and digitization described how the field of artificial intelligence (AI) and machine learning (ML) is rapidly evolving. The speed of development and AI/ML’s wide range of applications lead to many practical issues, and this column briefly considers some of them. Data is key to successful AI/ML modeling, so it is no wonder that data is central to many of these issues.
Regulatory and safety compliance
“Black-box” ML models that are employed in applications where regulations and safety are key can be difficult to trust because they do not provide the requisite level of transparency required for compliance. “Grey” or “white box” ML models such as physics-informed and explainable-AI models are potential aids to building trustworthiness.
Ethical concerns
Model bias can lead to potentially significant ethical issues. AI/ML models learn from the data they are being “fed.” As such, if the dataset is outdated or incomplete, the models will likely predict biased results. If the ML model developer is not aware of the potentially dangerous bias problem, that is an issue; if they are aware but do not account for it, that is an ethical issue. For example, in pharmaceutical drug discovery, bias is a difficult problem to solve. Clinical trials aim to recruit statistically appropriate patients with regard to sex, race, age, and other characteristics to avoid biased ML models.
Data privacy
Companies must comply with privacy laws in collection, storage, and processing of individuals’ data, whether of employees, clients, or marketing contacts. Therefore, data-privacy concerns can lead to complicated legal issues when AI/ML models are involved. Although high-end security protocols keep evolving, the activity is costly and time-consuming.
Intellectual property
Determining ownership of data is relatively straightforward; for example, refinery data belongs to the refinery. However, determining ownership of AI-generated discoveries can be complex. If an off-the-shelf large language model (LLM) such as ChatGPT or Copilot is used to generate solutions, who owns the intellectual property (IP) associated with the solution space? The legal department has to answer such questions, which are non-trivial. The legal underpinnings of AI/ML IP are still nascent.
Changes in organizational culture and processes
The culture, decision-making, and work processes in large organizations are often slow to adapt to new technologies. Resistance to change is not necessarily a bad thing in the short term. However, in the long term, such resistance to change can be crippling. Companies that are resistant to change can fail — think of Blockbuster, Kodak, Nokia, Sun Microsystems, and Polaroid. Admittedly, most of these companies were focused on technological products, unlike gas/oil/energy companies whose technological advancements typically focus on efficiency improvements and environmental sustainability. However, such companies must continue to embrace AI/ML.
Skilled workforce availability
A workforce capable of steering AI/ML development will be needed, and these workers will rely on data science and computer programming skills; this might include programming languages such as Python, Julia, R, and Java. In the short term, engineers and scientists can take paid certificate courses and free online courses (e.g., Coursera) to build necessary skillsets. In the long term, the process and pharmaceutical industries will need to hire chemical engineers who have data-science backgrounds. Retaining talent with the necessary AI skills is not easy because there is a high demand for such skills across industries. Other employees may fear losing their relevance when they cannot quickly acquire proficiency in a new technology such as AI/ML. Such fear could provoke pushback against AI/ML and increase anxiety or decrease motivation within an organization.
A practical alternative is to subcontract AI/ML services to specialized data-science companies. However, these companies might lack necessary domain knowledge and miss crucial insights. From experience, it is usually easier to educate a chemical engineer in data science than to educate a data scientist in chemical engineering.
Interdisciplinary collaboration
Engineers and scientists with different STEM backgrounds often work together in teams to solve problems. This interplay of different domain-specific knowledge has worked well. Now those teams must incorporate employees with AI/ML domain knowledge, possibly creating barriers to interdisciplinary collaboration. If new teams are built from the ground up with such collaborations, such barriers can be minimized.
Economic costs
Managing costs associated with AI/ML could be a deal-breaker, involving investments both in AI infrastructure and periodic maintenance. Costs can be substantial and potentially will escalate given AI/ML’s fast-paced evolution. The fact that the AI/ML landscape may look different in three to five years further complicates the cost scenarios.
In summary
In summary, chemical engineers must learn about the technology of AI/ML, but we also must acknowledge and take into account its associated challenges.
This article is also featured in the ChE in Context column of the October 2024 issue of CEP . Members have online access to complete issues, including a vast, searchable archive of back-issues found at www.aiche.org/cep.