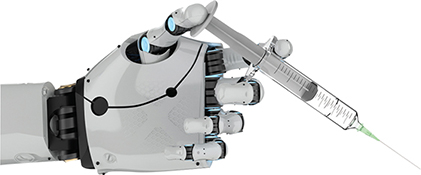
A wave of change is spreading across many industries due to technological advances enabled by artificial intelligence (AI) and automation. The pharmaceutical industry is no exception and is harnessing AI tools for new drug discovery and development.
AI is a machine process that makes predictions or takes actions based on data, and often attempts to imitate human behavior. However, computers and machines are unable to interpret why specific data is important and they lack the emotional intelligence to determine which result is most relevant. Most experts agree that AI in its current form should be used for tasks that are repetitive and lack efficiency, as opposed to those that require human understanding. Within the pharmaceutical industry, these tasks are usually associated with big data.
Donna Conroy, founder of SciMar One — a consultant group that utilizes data analytics in pharmaceutical development — explains that in the pharmaceutical industry, AI tasks should be low-risk, be incapable of causing harm to patients, and reduce overall costs to make downstream products more affordable. One such AI task might be predicting the efficacy and safety of a new molecule within a biological system by referencing a genomic database. Historically, molecule identification requires both large datasets and lengthy time frames — areas where AI systems could prove beneficial.
Pharmaceutical experts report that many companies are using AI for drug discovery. These organizations are harnessing machine learning (ML) to identify the molecular structures of new drugs, for example, or to determine the best cellular genomics to produce a certain active pharmaceutical ingredient (API). ML is a branch of AI that gives computers the ability to “learn” from input data without being explicitly programmed. Companies can either construct their own ML models, or use existing models. (For more on ML, check out the two-part series that appeared in the June and October 2018 issues of CEP.)
The drug development process requires emotional intelligence, experience, intuition, and rapid decision-making. This phase of the drug lifecycle is marked by routine process specializations (such as toxicity and usability testing). These tasks can require empathetic insights to evaluate the varying treatment- and disease-related datasets to extract the relevant data for the stakeholders (e.g., physicians, patients, or investors). The ability to pivot quickly during this development phase is crucial, as learning happens in real time. AI lacks the capacity to interpret, assess, and pivot due its dearth of human intelligence, and, as a result, it is unable to fully evaluate the data that it processes.
Although the efficiency intrinsic to AI is expected to surpass human capabilities in the future, at present, a human still needs to observe and validate the necessity and utility of a molecular substance before it nears testing and clinical trials. Humans are still best-suited to most drug development tasks. However, opportunities abound for AI in the microtasks that assist human decision-making, which can help expedite the process. For example, AI has been used to identify key patients for clinical trials. Being first to market in clinical-trial enrollment is crucial, and using AI to pinpoint the best patients to enroll can help the clinical trial kick-off sooner. Novartis used AI to combine clinical trial data from a variety of internal sources to predict and monitor trial enrollment, cost, and quality.
In general, once a drug or therapy gets past the development stage, investors are willing to spend more money to promote it. Some AI development efforts moving toward commercialization involve the personalization of medicine — for example, delivering personalized, targeted therapies to specific patients, or determining a new therapy’s efficacy and side-effects profile for a specific patient or patient group. CardioDiagnostics, for instance, provides tools for wireless heart monitoring; AiCure is a smartphone app that ensures users take their medication at the right time; and Eularis transforms pharmaceutical companies’ big data into actionable insights. Novo Nordisk’s AI assistant Sofia uses ML and natural language processing to field questions from diabetes patients and provide answers, learning from each interaction to improve responses for patients that were once handled by nurses at a call center.
The Pharmaceutical Discovery, Development and Manufacturing (PD2M) Forum’s Future of Pharmaceutical Manufacturing Conference and the PD2M Process Control Conference, both planned for later this year, will explore current and future implementation of ML and AI in the pharmaceutical lifecycle. We hope to see you this fall at the upcoming conferences.
Copyright Permissions
Would you like to reuse content from CEP Magazine? It’s easy to request permission to reuse content. Simply click here to connect instantly to licensing services, where you can choose from a list of options regarding how you would like to reuse the desired content and complete the transaction.