In-process measurement provides continuous information about particle behavior under changing process conditions, enabling engineers to optimize difficult processes.
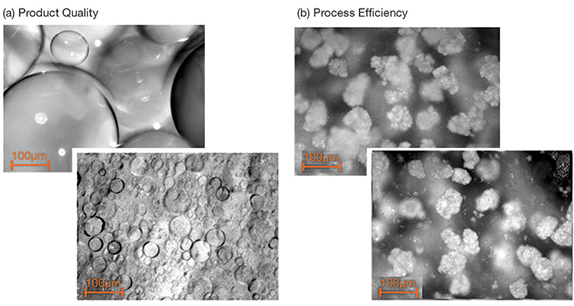
▲Figure 1. Particle size and count can influence product quality, as well as process efficiency. (a) Small droplets (front) deliver medicine in an ointment faster than larger droplets (back). (b) Separation times are longer when small crystals (front) block filters.
Particles, crystals, and droplets are present in many manufacturing processes and products, and often pose challenges for the engineers who must optimize product quality and process efficiency. The proper control of particle size, shape, and count is often a critical factor in final product quality, and can greatly influence process efficiency (Figure 1).
For example, the effectiveness of medicines used to treat lung diseases depends heavily on particle size; small particles penetrate into the airways faster than large particles (1). Particulate processes take longer to optimize and scale up than other unit operations and require continuous troubleshooting to operate effectively (2).
The optimization of process parameters is critical to consistently obtain the desired particle attributes. For instance, in crystallization, the cooling rate directly influences final crystal size — faster cooling rates typically produce smaller crystals (3). In emulsification processes, mixing intensity must be controlled to obtain the desired droplet size distribution (4). In the flocculation of fiber cement particles, high-molecular-weight polymer flocculants increase aggregation and produce larger and stronger flocs (5).
Multiphase systems involving combinations of particles, droplets, and bubbles add complexity to the challenge of understanding, optimizing, and controlling the processes used to produce, modify, or separate them.
Understanding how process parameters affect particle properties and characterizing particle properties effectively, particularly particle size and count, enables engineers to solve processing problems and improve product quality. Historically, particle characterization was performed by offline particle size analyzers, such as laser diffraction or sieving. In recent years, newer technologies have emerged that describe particle size and count in real time, as particles naturally exist in the process.
This article introduces some of the most common in-process particle measurement approaches, and explains how they can be deployed for the effective delivery of high-quality particle products.
Traditional particle size analyzers
Traditional particle size analysis (PSA) using an offline analyzer is a powerful and widely used technique for the measurement of particle size in quality control (QC) labs. Examples of traditional PSA techniques include sieving, laser diffraction, dynamic light scattering, electrozone sensing, and image analysis. Because these techniques employ different methods of measurement, different techniques might report different particle size distributions for the same system (6). Some techniques, such as electrozone sensing, can report particle count and size in the same measurement. Other techniques are ensemble methods that can report only particle size. Offline particle size analysis allows QC laboratories to check the physical properties of particles at the end of a process, and identify deviations from the targeted particle system specifications. To obtain useful results from traditional particle size analyzers, consider the following points.
Sampling and sample preparation. A successful laboratory analysis of particle and droplet systems requires the removal of a representative sample from the process and the preparation of the sample for analysis. Most PSA techniques have strict constraints on the range of concentration, size, and shape of particles that can be measured accurately.
Sample preparation often involves multiple steps to meet those measurement constraints, and can include methods such as filtration, rinsing, drying, subsampling, resuspension, surfactant addition, dilution, and sonication. However, it is quite possible that these steps may significantly alter the particles or droplets of interest.
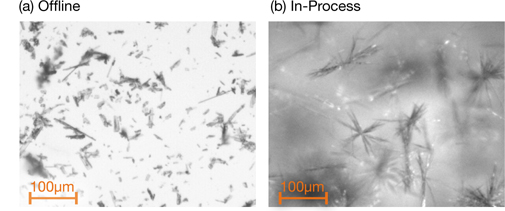
▲Figure 2. Sampling and preparation of mannitol crystals for offline analysis by a standard light microscope caused significant crystal breakage (a), which is avoided with inline analysis using real-time microscopy (b). Image courtesy of Brian O’Sullivan.
Even with the utmost care and precision in the sampling and sample preparation methods, the actual particles that are analyzed may be significantly different from the particles that were initially present in the process (Figure 2). Thus, particles must be sampled in a way that minimizes the possibility that change might occur during the removal, preparation, or measurement phases of the procedure (6).
Droplets and bubbles are particularly prone to change upon sampling. The interfacial forces present in the process stream that dictate the droplet or bubble size distribution are changed completely when a sample is taken and exposed to ambient conditions. This makes these delicate systems difficult or even impossible to sample effectively.
Particle shape. In order to report consistent results, many particle size analyzers employ simplified models that assume particles are spherical (7). A sphere is the simplest particle shape in the sense that one number, the diameter, describes the particle size completely.
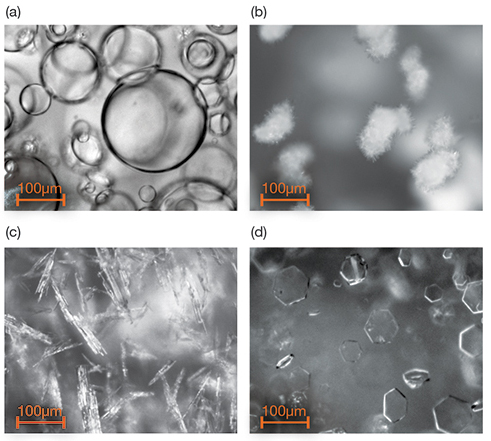
▲Figure 3. Particles may take many different forms: (a) spherical droplets, (b) agglomerated crystals, (c) elongated rod-like particles, and (d) hexagonal platelet crystals.
Often, however, particles are nonspherical (Figure 3), and particle shape can be even more important than size in determining bulk solids properties, such as flowability (8) and filterability (9). Engineers must understand how particle shape influences a traditional PSA technique, and in cases where particles are known to be nonspherical, take this into account when analyzing results.
Furthermore, particle systems consist of a population of particles of different sizes and shapes. Many traditional particle size analyzers calculate the particle size distribution according to a theoretical model that calculates an average, typically a mean or median. Particle count at the fine and coarse tails of such a distribution might be influenced by the method of measurement and the mathematical treatments of the reported measurement.
Time delay. Most particle process streams operate at a solids loading much higher than anything traditional particle size analyzers can handle. Thus, careful sample preparation is needed for accurate measurement. It is virtually impossible to apply traditional offline PSA measurements directly in a process. In order to obtain continuous information relating particle size to process parameters, samples would have to be manually extracted and analyzed immediately, without interruption. This approach is challenging from a cost perspective, and may expose operators to an unacceptable level of risk, especially for processes that operate at high temperatures and pressures or that contain toxic or explosive slurries and solvents. The inevitable time delay between sampling and receipt of results makes it extremely difficult to implement traditional PSA for real-time measurement. These analyzers, therefore, are unsuitable for monitoring processes continuously as they change over time.
Traditional PSA for QC. Offline PSA is a powerful and widely used quality control technique for the measurement of particle size, and for comparison with a specification. With care, traditional PSA can be used to identify variations in product quality and to ensure that products meet the specifications required by producers, their customers, and regulators who oversee the quality of products reaching the public.
Particle size analysis for process optimization
Traditional PSA, however, does not lend itself to characterizing particles continuously as process parameters change, and for this reason it is not well-suited to the task of process optimization. It is extremely difficult to rely on a single offline sample, no matter how reliable the data obtained, to completely understand particle behavior from the beginning until the end of a process. To develop a truly effective process understanding and to translate this into meaningful improvements for the process, continuous...
Would you like to access the complete CEP Article?
No problem. You just have to complete the following steps.
You have completed 0 of 2 steps.
-
Log in
You must be logged in to view this content. Log in now.
-
AIChE Membership
You must be an AIChE member to view this article. Join now.
Copyright Permissions
Would you like to reuse content from CEP Magazine? It’s easy to request permission to reuse content. Simply click here to connect instantly to licensing services, where you can choose from a list of options regarding how you would like to reuse the desired content and complete the transaction.